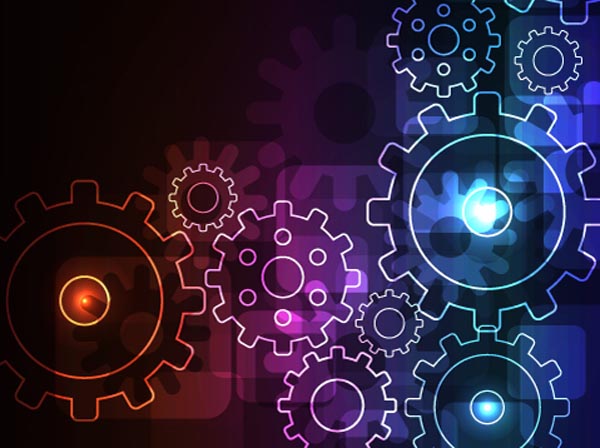
Artificial Intelligence (AI) has penetrated nearly every industry because of its ability to improve business outcomes – from employee productivity to decision-making to customer experience. It’s no surprise that organizations large and small are embracing AI. That being said, starting AI without a strong Data Strategy in place can do more harm than good.
Data Strategy refers to a set of elaborate plans and processes to generate and analyze valuable data in support of enterprise objectives. As more businesses adopt AI, it’s critical to understand the need for AI and how it fits in with an organization’s overarching business goals. In addition to that, AI comes with certain risks and challenges, such as ethical and privacy considerations, which can impact data security and compliance. This is why Data Governance must also be a key part of any strategy.
This article will focus on how and why data leaders are incorporating AI into their enterprise-wide Data Strategy to achieve long-term success.
The Value of AI
AI is the practice of using computers and other machines that simulate human intelligence to perform tasks. Despite fears of job-stealing robots, AI does not completely undercut human-led processes; instead, it automates tasks that do not require human intervention, helping to boost enterprise efficiency.
Although AI is often confused with machine learning, the two terms are not synonymous. Machine learning – a subset of AI – analyzes data and learns from it, while AI provides actionable intelligence for decision-making based on those insights.
From marketing to e-commerce to health care, numerous industries have turned to AI, with implementation on the rise: A recent McKinsey report estimated that 56% of global companies have adopted AI in at least one function, up from 50% in 2020. In addition, global spending on AI is expected to rise from $85.3 billion in 2021 to more than $204 billion in 2025.
Why are data-driven businesses investing in AI? Here are a few key benefits:
- Automated business processes: Advanced technologies such as robotic process automation (RPA) can automate tedious, repetitive tasks, freeing up employees to focus on more important tasks that may require slow or elaborate working processes.
- Improved data analytics: With the help of machine learning algorithms, organizations can use AI to analyze data objectively, resulting in improved insights (unless bias comes into play). Eventually, the interpreted data can translate into actionable reports for decision-makers.
- Fewer Errors: Human-led analysis has a critical issue – lack of accuracy. Outcomes prone to errors mean wasted time and effort. AI allows for more accuracy, though models need to be fed large amounts of data.
- Higher ROI: The significance of investment in large-scale implementation is multifold. Businesses tend to save money by using AI because it can automate tasks without taking breaks and reduce the margin of error. Plus, algorithms keep learning when more data is fed to them, making them better with time. All of this leads to increased returns and business growth.
In an op-ed, Tom Davenport, professor of IT and management at Babson College, and Joey Fitts, VP of analytics product strategy at Oracle, further explain:
“AI-enhanced analytics systems can prepare insights and recommendations that can be delivered directly to decision-makers without requiring an analyst to prepare them in advance. Small to mid-size businesses that haven’t been able to afford data scientists will be able to analyze their own data with higher precision and clearer insight.”
Why Is Data Strategy Essential?
Nitish Mittal, a partner in the digital transformation practice at Everest Group, emphasizes this point:
“I can’t stress this enough: data or the lack of the right data strategy is the number one bottleneck to scaling or doing anything with AI. When clients come to us with what they think is an AI problem, it is almost always a data problem. AI depends on viable data to prosper. That’s why it’s important to think about the data first.”
Granted, it is no easy task to create a Data Strategy, let alone one that supports AI capabilities. Data Strategy needs to be aligned with the organization’s objectives and be modified as and when those objectives change. Without having a comprehensive, up-to-date Data Strategy, the investment of time, effort, and money in AI will be futile.
How to Develop an Data Strategy That Supports AI
Data Strategy can enable the effective application of AI by providing a timeline, structure, and support to overcome challenges.
Mike Rollings, research vice president at Gartner, recommends taking the following steps when developing an AI-focused Data Strategy:
- Assess the relevance of AI to the organization’s most important business outcomes
- Determine which types of applications (e.g., virtual customer assistants) to leverage
- Address the organizational, governance, and technological challenges associated with AI
Which use cases will be most beneficial for the business to pursue? Is there sufficient clean, ready-to-use data to deliver the projected outcomes? Having an abundance of data does not provide value if it contains many errors.
Beena Ammanath, executive director of Deloitte AI Institute, stresses quality over quantity:
“It’s not enough to say you have 20 years of data. You have to have the right data. You may have high quantities of data, but you may not have the quality you need. Many companies don’t have a data architecture capable of pulling in data from different places and cleaning it up so it’s usable for AI technology.”
Establishing Data Governance will not only improve Data Quality but will also ensure it is used in an ethical way. Any underlying bias in the data or algorithms can be exacerbated if not tackled – and can undermine trust in AI. Incorporating a debiasing strategy such as using bias-detecting tools and improving data collection processes will reduce the chances of bias. Furthermore, AI governance can help organizations meet compliance with data privacy regulations.
Trends in AI Strategy
Despite the rise in AI adoption across industries, concerns about bias, privacy, quality and quantity of data, and more remain. Here are a few trends and strategies companies are exploring to minimize risks:
- Small data: Organizations are shifting their focus from big to small data stored in emails, Excel files, and the like. This approach allows for collecting larger amounts of relevant data that will ultimately make AI “less data hungry.”
- Synthetic data: Artificially generated data can help fill in the gaps of real-world data sets. Plus, it eliminates the need for access to potentially sensitive private data. Gartner predicts that by 2024, synthetic data will account for 60% of all data used for AI and analytics.
- Responsible AI: Establishing responsible AI guidelines increases the likelihood that AI systems will be secure, respect privacy, and avoid biases.
- CDO to the rescue! More businesses are hiring chief data officers (CDOs) to improve Data Strategy and speed up AI implementation.
As AI continues to become more accessible – today’s tools are more affordable than their predecessors and cloud-based AI considerably cuts costs – we can expect to see even more organizations developing an AI-first Data Strategy to differentiate themselves from their competitors and make smarter decisions over time.
Image used under license from Shutterstock.com