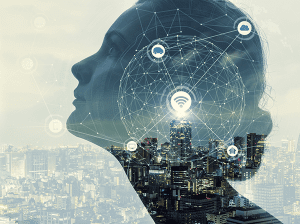
As we delve deeper into the age of artificial intelligence (AI) and machine learning (ML), it is crucial to stay ahead of the curve by identifying emerging trends that will shape our future. In 2024, several key advancements are set to revolutionize these technologies, paving the way for unprecedented possibilities. One of the top AI and machine learning trends for 2024 is the increased integration of AI and ML into everyday life.
AI and ML Trends to Watch for in 2024
From autonomous vehicles to smart homes, AI and machine learning technologies will become seamlessly woven into daily human routines. Another exciting development is natural language processing (NLP). By 2024, NLP algorithms will enhance a machine’s ability to comprehend human language with remarkable accuracy.
Explainable AI: NLP will revolutionize customer service chatbots, virtual assistants, and language translation tools. Also, explainable AI (XAI) is poised to gain prominence in 2024.
As AI systems become more complex, understanding their decision-making processes becomes increasingly critical. XAI aims to provide transparency by offering insights into how algorithms arrive at conclusions or recommendations – an essential aspect of building trust in AI applications.
Deep learning models: Deep learning (DL) and neural networks have emerged as the driving force behind many recent advancements in AI and ML. These technologies are poised to shape the future landscape of AI and ML in profound ways. One major trend that will continue to gain momentum in 2024 is the development of more complex and sophisticated DL architectures.
Researchers are designing neural networks that can handle increasingly complex tasks, such as NLP, image recognition, and even autonomous decision-making. These advancements will enable AI systems to become more intelligent, adaptable, and capable of handling real-world challenges.
Another important area of focus is improving the efficiency and scalability of DL models. As AI applications become more prevalent across industries, there is a growing need for DL models that can process vast amounts of data quickly and accurately.
Researchers are exploring techniques like distributed computing, parallel processing, and model compression to enhance the performance of DL algorithms. Furthermore, efforts are being made to make deep learning more explainable and interpretable.
Currently, one limitation of neural networks is their black-box nature – they often lack transparency in how they arrive at their decisions. Addressing this challenge will be crucial for building trust in AI systems among users and stakeholders.
NLP and robotics: NLP and robotics are jointly spearheading a revolutionary transformation in the way humans interact with machines. As technology continues to advance at an unprecedented pace, the fusion of NLP and robotics is set to redefine the boundaries of human-machine interaction by creating more intuitive and seamless interfaces.
This breakthrough technology has paved the way for voice assistants like Siri and Alexa, which have become ubiquitous in our daily lives. In 2024, we can expect NLP to evolve even further, enabling machines to comprehend complex nuances of human speech and respond with enhanced accuracy.
When combined with robotics, NLP takes human-machine interaction to new heights. Robotics provides physical embodiment to AI systems, allowing them to perceive their surroundings and interact with objects in the physical world.
This revolutionized interaction between humans and machines holds immense potential across various domains such as healthcare, customer service, manufacturing, education, and entertainment.
Computer vision, AR, And VR: Computer vision, augmented reality (AR), and virtual reality (VR) technologies are set to transform how humans perceive and immerse themselves in the surrounding world. As AI and ML continue to advance, these technologies will become even more sophisticated and impactful in the year 2024.
Computer vision, powered by AI algorithms, helps in capturing visual information from images or videos. In healthcare, computer vision can aid in early disease detection by analyzing medical scans with unparalleled accuracy. In transportation, it can enhance autonomous vehicles’ perception capabilities for safer navigation.
AR and VR will transport human perception to a unique world of “blended digital content and real-world environments.” In education, AR can transform traditional learning methods by overlaying interactive elements onto textbooks or classrooms, making lessons more engaging and interactive.
VR opens up possibilities for realistic training simulations in industries like aviation or manufacturing. Moreover, AR and VR hold promise for gaming and entertainment sectors by enabling creating “suspensions of disbelief” – blurring the line between the real and virtual worlds.
Reinforcement learning and automation of jobs: A significant trend that will shape the future of work is the increasing automation of jobs through reinforcement learning. Reinforcement learning is a type of ML where algorithms learn to make decisions based on the instant feedback or rewards they receive for their decisions. This approach enables AI systems to optimize their performance over time, ultimately leading to autonomous decision-making.
The implications for the workforce are profound. While automation has been a concern in various industries for years, reinforcement learning takes it a step further by allowing AI systems to adapt and improve continuously without human intervention.
Due to AI, traditional workplace roles that involve repetitive tasks or predictable decision-making may disappear. However, there will be an increased demand for individuals who can work alongside AI systems, leveraging their capabilities while bringing uniquely human skills such as creativity, critical thinking, empathy, and problem-solving.
Intelligent virtual assistants and ethical AI: As AI and ML continue to evolve, intelligent virtual assistants (IVAs) are emerging as an integral part of our daily lives. These AI-powered assistants, such as Apple’s Siri or Amazon’s Alexa, have transformed the way humans interact with technology by providing personalized experiences and intuitive interfaces.
A significant challenge involves bias within AI algorithms. IVAs are trained on vast amounts of data that may contain inherent biases present in society.
Another significant concern is data privacy. IVAs constantly collect data about users’ preferences, behaviors, and even personal conversations. This raises questions about how this data is stored, used, and protected. Striking a balance between convenience and privacy will be vital in maintaining public trust.
Top Five AI Trends to Continue from 2023 to 2024
The coming year holds immense potential for groundbreaking advancements in AI technology. Here are the top AI trends that are set to dominate the AI landscape:
- Ethical AI: With increasing concerns around bias and privacy, ethical considerations will play a significant role in shaping the development and deployment of AI systems. Organizations will prioritize building transparent, fair, and accountable AI solutions that align with societal values.
- Conversational AI: NLP has already transformed how we interact with machines. In the coming years, conversational AI will become even more sophisticated, enabling more seamless interactions between humans and machines through voice assistants, chatbots, and virtual agents.
- Edge computing: As data volumes continue to explode, processing power at the edge becomes crucial for real-time decision-making. Edge computing combined with AI capabilities will enable faster analysis of data at its source while reducing latency and dependence on cloud infrastructure.
- Explainable AI XAI: A lack of transparency hinders trust and limits the adoption of AI systems in critical domains such as healthcare and finance. Therefore, XAI emerges as a solution to address these challenges. XAI focuses on developing AI models that can offer plausible explanations for arriving at a particular decision. This approach involves utilizing techniques such as rule-based systems, NLP, and visualization methods.
- The intersection of cybersecurity and AI: The year 2023 witnessed a growing concern regarding the vulnerability of AI systems to cyber threats. This concern has prompted a significant focus on advancing toward secure AI by exploring the intersection of cybersecurity and AI. The potential risks associated with AI are multifaceted, ranging from adversarial attacks that manipulate AI algorithms to data poisoning aimed at corrupting training datasets.
To mitigate these threats, researchers and industry experts have started emphasizing the need for robust cybersecurity practices specifically tailored for AI systems. One area of research revolves around developing secure ML algorithms that are resistant to adversarial attacks. These algorithms utilize techniques such as generative adversarial networks (GANs) and differential privacy to enhance resilience against malicious manipulations. Additionally, efforts are being made to create trustworthy datasets by implementing strict data validation processes and employing anomaly detection methods.
Top Five ML Trends to Continue from 2023 to 2024
Here are the top ML trends that will continue to make waves this year.
- Quantum machine learning (QML): QML has emerged as a game-changer in the field of AI, with significant advancements and applications anticipated to continue from 2023 to 2024. QML combines the power of quantum computing and ML algorithms to solve complex problems that are beyond the capabilities of computers.
- Transfer learning for ML: Transfer learning has emerged as a revolutionary concept in the field of ML, bringing significant advancements and success stories. This approach enables ML models to leverage knowledge gained from one task and apply it to another, resulting in improved performance and reduced training time. One key development in transfer learning is the pretraining of deep neural networks (DNNs) on massive datasets. By training models on vast amounts of data, they can learn general features that are applicable across a wide range of tasks.
- Anomaly detection in ML: Anomaly detection has emerged as a critical component in machine learning systems, enabling the identification of outliers or unexpected patterns in datasets. As ML applications continue to grow in complexity and scale, the need for accurate anomaly detection techniques becomes paramount to ensure optimal performance and efficiency. One prominent trend in anomaly detection is the integration of advanced statistical models with ML algorithms.
- Time Series forecasting for predictive analytics: Time series forecasting has long been a crucial aspect of ML, providing valuable insights into future trends and patterns. As we move into 2024, this trend is expected to continue, with several innovative approaches that will further enhance the accuracy and efficiency of predictive analytics. One major advancement in time series forecasting is the integration of DL techniques. DL models, such as recurrent neural networks (RNNs) and long short-term memory (LSTM) networks, have shown promising results in capturing complex temporal dependencies within time series data.
- Data augmentation takes center stage in ML: In the realm of ML, data augmentation is emerging as a key trend that is set to continue from 2023 to 2024. As ML models become more complex and require larger datasets for training, data augmentation techniques offer a solution to address the scarcity of labeled data. Data augmentation involves generating synthetic examples by applying various transformations to existing data samples.