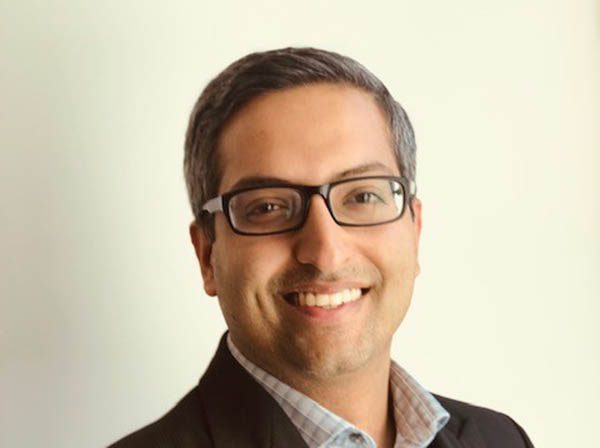
Click to learn more about author Sameer Vadera.
Artificial intelligence (AI) applications have an insatiable appetite for consuming data. Today’s AI models for business applications are built to ingest massive amounts of complex data sets. The cost of collecting and curating data for training AI models, however, can be staggering. In the context of the Internet of Things (IoT), for example, the costs of deploying sensors and other machinery in a network at a big data scale can be expensive.
But what if the training data that trains your AI products is accessible online to your users? Bad actors mimicking legitimate users can siphon off large amounts of the data you collected and then inexpensively build competing AI products using this training data. Losing data to competitors can translate to lost market share. In China, for example, a company invested heavily in attaching a network of sensors on buses to collect real-time bus location data. The company built a popular AI-powered app that predicted future bus times with high accuracy. The AI-powered app was trained using the real-time bus location data collected using the sensors. A competitor coded a bot that scraped the real-time bus location data to improve the accuracy of its competing AI-powered app. While this ultimately went to court, the company still suffered economic loss and damage to its brand as a direct result of losing its real-time bus location data to its competitor.
In light of the risk of losing training data to competitors, defining strategies for owning or otherwise protecting your business’s training data is critical. But what are those strategies, and what are the challenges of employing those strategies?
Can Copyright Protect Training Data for AI Products?
Yes, but copyright protection of training data can be thin. The individual data elements of training data cannot be protected by copyright, but the organizational structure of the training data can be protected if there is originality in the selection or arrangement of the data elements. On a big data scale, however, training data can be messy and constantly growing.
- The challenges of obtaining copyright protection for training data are significant. Collecting all information available, such as collecting all detectable information in an industrial control system, reduces the prospects of meeting the minimum threshold of originality. Is there a creative aspect to collecting all data that is collectible?
- Copyright is often better suited to static data sets. Training data at a big data scale, however, is rarely static. The variety and velocity of training data limit the value of copyright protection for robust data sets.
Can Trade Secrets Protect Training Data for AI Products?
Yes, but businesses should take reasonable measures early on to keep the data secret. In order to be a protectable trade secret, the training data must derive independent economic value from not being generally known or readily ascertainable through proper means. Given that the data collected from users to train AI products is an asset to many businesses, training data can easily derive economic value. But the key is taking steps to keep the training data under “lock and key” within your business’s network architecture.
- Vendors are often given access to a business’s training data to provide services. These vendors should be legally obligated to keep the training data safe under “lock and key” away from public exposure.
- Additionally, unlike copyright protection, trade secret protection does extend to the underlying data elements of training data.
Protect Training Data for AI Products Using Contracts
Contracts are the first line of defense for many businesses when copyrights and trade secrets do not provide adequate protection or are not feasible for some reason. Effective legal obligations should be put into place upstream before app users or third-party vendors get access to the training data. Consider using a contract to restrict access to the training data unless the user agrees not to copy or commercially exploit the training data. Common contract mechanisms include website terms-of-use agreements and contracts with vendors accessing the training data.
What Other Avenues are Available for Protecting Training Data?
- Technical measures: Technical measures can include, for example, password-protecting access to training data, encrypting the training data, configuring website designs to increase the difficulty of scraping data from your business’s website, and creating mandatory click-through agreements that create legal obligations to prevent misuse of the training data.
- Digital Millennium Copyright Act (DMCA): The DMCA prohibits users from circumventing technological protection measures. The challenge, however, is that the DMCA requires that the training data include copyrighted content owned by the party seeking protection. In the IoT context, the training data rarely contains copyrighted data.
- Computer Fraud and Abuse Act (CFAA): The CFAA prohibits accessing a computer in an unauthorized manner. While businesses have asserted CFAA claims against competitors deploying data scrapers, data scraping generally falls outside of the prohibited “access without authorization” covered by the CFAA, especially in scenarios where the data was publicly accessible on a website or through a native app.
- Competition Law: Creative approaches to protecting training data that has been misappropriated by competitors include asserting competition law claims, such as misappropriation, lost profits, unfair competition, electronic trespass to chattel, and others.
What About Open Data Licenses?
Consider whether there is business value to protecting mission-critical training data (e.g., using trade secrets) and openly providing the remainder of the training data under an open data license. Open data licenses are similar to open source licenses, in that the licenses encourage the sharing of data. This approach has the advantage of placing some of the burdens of improving Data Quality and data curation on stakeholders. In the machine learning (ML) and AI context, improving the Data Quality of training data before ingesting the data into AI models can be burdensome and costly to a business. Providing the training data to stakeholders who take on the burden of improving Data Quality can cut overall costs.
Summary
There are many avenues for the legal protection of your business’s training data. Considering all the avenues is prudent, especially considering the enormous value that training data can yield when used to train your business’s AI products.
Implementing technical measures (e.g., password-protecting data) and legal obligations (e.g., website terms-of-use agreements) early before data scrapers can access training data bolsters a business’s prospects of protecting data.
Consider protecting core training data for AI products using trade secrets and making the remaining data openly available under open data licenses. This approach can increase the certainty of protection and reduce costs, for example, by avoiding the need to improve Data Quality or manage data curation of the data.