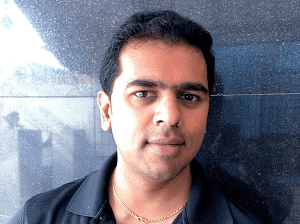
Data analytics and integration are the key components of building a data strategy. For organizations to have an effective data strategy, it requires the definition of measurable metrics and proper consideration of all data sources. An effective data strategy also needs to define how data can be moved from various sources to a location where it can further be used for analytics.
With the ever-growing edge deployments to meet the demands of IoT, smart devices, gaming technologies, and other similar technologies, combined with the recent hype toward AI and specifically generative AI, organizations are under pressure to derive the right data strategy that not only considers all data sources but also deploys it in a cost-effective manner that can help make good business decisions.
This article aims to provide an overview and factors that need to be taken into consideration for building an effective data strategy that takes all challenges and opportunities associated with edge and cloud deployments into consideration and leverages the advantages of artificial intelligence. The focus of this article will be on the integration aspects of data from various deployments only and not on analytics insights.
What Are Edge Deployments?
Edge deployment is a concept associated with the deployment of systems closer to a customer premise with the intent of providing localized, low latency and faster response. Ever since the inception of this concept, it has gained a lot of attention primarily because it has the potential to provide a localized customer experience with a quick turnaround time. These deployments are typically smaller in size and are focused on addressing critical business needs. Ideally, organizations will have their solutions deployed at multiple edge locations to address their customer base and these are expected to be connected to a main data center that is hosted in the cloud.
Advantages of Edge Deployments
Not all organizations need their solutions to be deployed at edge locations. Organizations deploying solutions at the edge do so only if they need to provide instant, customized, and localized responses to their customers. Edge deployments provide the following advantages:
- With computation in the edge, organizations can provide localized or customized experiences and quicker responses to customers. Additionally, since all the computing happens on the edge, the degree of certainty and reliability even when there are network constraints or disruptions would impact communication with the cloud.
- With AI getting into the mainstream, cloud providers are under increased pressure to meet the high demands of AI workloads. There are challenges both from hardware resources and sustainability parameters perspectives, as both are limited. Therefore, organizations need to deploy AI-based workloads in edge sites to address the concerns and balance workloads.
- Organizations deploying solutions at the edge typically collect data and store that on the same site. This activity provides advantages from both security and data governance perspectives. As data is processed in the edge, the chances of a data breach are less likely, and international laws of storing data within local boundaries can be adhered to.
- Edge computing brings the operational costs lower as data is stored and processed locally. Additionally, in situations when connectivity with the cloud or other edge data centers goes down, edge data centers can operate offline. This provides the ability for customers to provide service to their customers even during downtime.
Analytics at the Edge
With edge analytics, organizations can process the data, gain insights based on analytics at the edge, and take appropriate actions. Processing the data here would mean cleaning, aggregating, and modeling appropriately for analytics purposes. Analyzing at the edge is faster, and the latency is very minimal. Therefore, for organizations that need to derive insights from connected devices and take appropriate actions in real time, edge analytics can come in very handy.
Edge Analytics vs. Cloud Analytics
The primary intent of both edge analytics and cloud analytics is to analyze all the data, derive insights, and facilitate appropriate decision-making processes. Here are some key differences between both.
- A centralized analytics solution hosted in the cloud considers data from all the sources that typically is massive. On the other hand, an edge analytics solution can consider only the data from the edge deployment or deployments that it has visibility into.
- Since a cloud analytics solution is deployed in a cloud, all the raw data needs to be transported to the cloud, cleaned, and preprocessed before feeding into the analytics solution. Moving data from various sources to the cloud can be time-consuming and further cleaning and modeling the data could also result in delays. Edge analytics, on the other hand, processes the data generated from the edge deployments it has visibility into. As edge analytics solutions are closer to the sources where data gets generated, there is minimal latency.
- Data integration activities such as preprocessing and normalizing the data become complicated activities when the data sources generate data in different formats. This activity can incur huge additional costs and is time-consuming as well. In the case of edge analytics, data integration activities will be performed at the edge and the data is typically not expected to be in different formats.
- Cloud analytics solutions provide a complete perspective of the overall state of the business as they have access to all data sources. Therefore, for organizations to analyze key performance indicators, they rely on cloud analytics solutions. Edge analytics provides metrics associated with a particular deployment or location and does not represent or provide the performance of the entire organization.
Considerations for an Effective Data Strategy
The primary purpose of a data strategy is to identify mechanisms for measuring key metrics that are part of the overall business strategy. Therefore, a data strategy needs to consider all data sources, identify appropriate preprocessing and modeling algorithms, and eventually feed the processed data into an analytics solution for detailed insights and actions.
In the case of organizations deploying interconnected edge solutions, massive amounts of data are expected to be generated in each of the edge sites. Therefore, an effective data strategy needs to consider cost implications while processing these massive amounts of data. Here are some key considerations for building an effective data strategy:
- A data strategy needs to have a clear definition of key performance indicators that map to the overall business strategy. These KPIs need to be measured at an overall organizational level. Based on business needs or strategy, if metrics need to be measured at edge locations for real-time decision-making or quicker turnaround time, the data strategy needs to consider KPIs for separate edge locations as well.
- A data strategy needs to comprehensively cover both data integration methods and analytics tools. For analytics at the edge, the data integration method is expected to be simple, since the raw data will be in a specific format. However, for analytics in the cloud, data integration technologies are expected to be complicated due to disparate data structures and the need to transform to a common structure before using it for analytics purposes.
- Data strategy needs to cover security, latency, and bandwidth considerations. If applicable, it also needs to cover data transfer through international boundaries.
- Data strategy needs to call out the hardware limitations of deploying analytics solutions in the edge and cloud, as both solutions have pros and cons. In the case of edge analytics, the effectiveness of analytics is dependent on the computation power in the edge site. If there are resource constraints in running analytics in the edge, the strategy needs to consider an alternative, nearby edge site that can perform the task.
- Organizations deploying edge analytics solutions need to be aware that the outcome or scope of the solution is only limited to that of the edge deployments that it has visibility into. Therefore, the effectiveness of the solution can only be achieved if the key performance indicators are specific to the edge.
- The effectiveness of a data strategy can be achieved only when it details out a specific strategy for both edge deployments and the overall cloud deployment. Only an analytics solution in the cloud can provide a comprehensive view of the organization’s performance. However, moving all the data from edge deployments into a central location in the cloud is a time-consuming, costly activity. With the recent enhancements in artificial intelligence, organizations can now leverage AI to effectively build a data strategy.
Leveraging Artificial Intelligence for Analytics
For organizations to leverage artificial intelligence when building a comprehensive cloud analytics solution, all the edge analytics solutions and the cloud analytics solution must be interconnected. When these solutions are interconnected, they can move data between sites as needed and can also provide complete visibility, both from a centralized perspective and an edge perspective. Here is a proposal of how organizations can incorporate AI into data strategy:
- Keep raw data processing only in edge locations. Edge analytics should not only provide analytics for the edge site, but they should also have the ability to send the output of the analytics through the network to other sites.
- An interconnected analytics solution should have the ability to send and receive the output of analytics to or from another analytics solution through the network. Since all the analytics solutions in the network are expected to be in the same format or in a format that does not require additional transformation, data integration and transformation shall be straightforward.
- With the help of appropriate AI algorithms, cloud analytics solutions should be able to request processed data from edge locations. Likewise, edge locations also should be able to leverage AI algorithms to exchange performance metrics and other key indicators with other edge locations and the cloud.
- Edge and cloud analytics solutions should use AI to learn from other deployments within the network and provide better insights.
Using AI effectively in edge deployments and analytics can effectively reduce the turnaround time and provide comprehensive analytics to the stakeholders.
The Need for a Comprehensive Data Strategy in a Multi-Deployment Model
Organizations are constantly looking for ways to provide a more seamless, localized, and quicker experience for customers. The recent technologies, 5G and edge, have been the primary drivers and provide platforms for all these organizations to achieve their goals. As organizations embark on this journey of deploying solutions in edge locations and evolve, they need a way to measure the performance of their solution. This can be accomplished through a comprehensive analytics model that not only provides a complete end-to-end view of their deployment but does so in a real-time and cost-effective manner. Leveraging AI, as explained in this article, is one of the potential ways of achieving this goal.