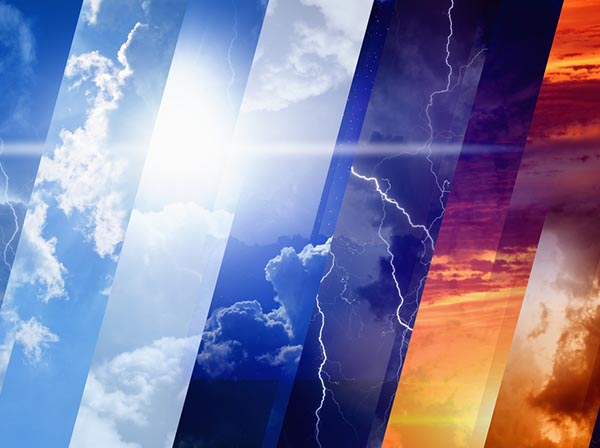
Weather companies want to do their best so that consumers and businesses can have reliable forecasts – not just for the day but in advance of it. Accuracy matters to weather services such as the Weather Channel and AccuWeather and predictive analytics, along with Data Science, is allowing them to get more precise weather reports than ever before. We all like to discuss what is going on outside, and we want to know if enough snow is expected for good skiing on an upcoming vacation, whether it will be pouring on a special occasion, or if the expectation of heavy storms with high winds requires a business to activate disaster recovery plans.
In fact, a multi-year study of forecast accuracy – Analysis of One- to Five-Day-Out Global Temperature, Probability of Precipitation and Wind Speed Forecasts – found that AccuWeather was the most accurate provider for wind and precipitation forecasts and was a co-leader in accuracy for temperature forecasts. For temperature forecasts, AccuWeather was the most accurate for high temperature forecasts, while The Weather Channel was the most accurate for low temperature forecasts.
AccuWeather’s clients include WABC in New York City, which has been using the weather service for nearly fifty years. Commenting on the report, AccuWeather founder, president and chairman Joel N. Myers noted that AccuWeather forecasts are much more localized to individual addresses and that it provides highly detailed forecasts both in location and time. “AccuWeather’s forecasts also extend farther into the future than any other source, with 90-day forecasts available for all locations on the planet,” he said.
As you might expect, data and data analytics rule in generating weather forecast success. The study mentioned above was conducted by ForecastWatch.com, a service of Intellovations, LLC. ForecastWatch defines itself as “the meterologists’ source” for unbiased data and analysis to improve forecast quality. The service collects weather forecasts and verifies them against actual observations for 86 countries, compiles forecasts and information from 1,350 locations, and stores 800 million historical forecasts in its proprietary database (so far). Weather companies and other businesses can use this for accurate analysis based on business parameters, or they can gain direct access to the database to create customizable data sets.
Between an organization’s own data and that supplied by ForecastWatch, weather services and other clients have a wealth of data on hand to measure forecast accuracy and quality. The data on hand serves as the base for conducting analytics for a number of use cases, including making strategic decisions such as which weather tools to invest in, says Eric Floehr, founder and CEO of ForecastWatch.
“Whenever an analyst or data scientist creates a model, they feed raw data into a machine learning system like a neural network or some linear regression system,” Floehr says. “They’re trying to take all the input data and find the best way to combine it all to generate predictions that will be as close as possible to what actually happens.”
Weather companies and other businesses can layer ForecastWatch’s data on top of their own in its cloud and run their models.
Who Benefits from Weather Watches?
Weather companies were the first industry to use ForecastWatch’s solution to measure forecast accuracy and quality. But its base of customers has been expanding to businesses in any field that needs highly reliable data for weather forecast predictive analytics.
In one example provided by Floehr, a large transportation company has improved its decision matrix by leveraging smart weather data for accurate analysis of upcoming weather events. “It has to do certain things to ensure the safety of their transports based on different weather conditions,” he says.
Another interesting example has to do with how sports arenas and team facility owners can take advantage of marrying their own data with ForecastWatch’s historical weather data to help build better business models. Gaining a better understanding of how weather likely will affect fan attendance – based on ForecastWatch’s historical weather data combined with their own attendance models – gives them the tools they need to predict and make decisions around how the arena should operate on certain days.
Previously, attendance models may have included data about a team’s record or the day of week or the time of a game, but without taking historical weather data into account, accuracy was hampered. Historical data about weather conditions can provide additional perspective on whether attendance will be strong for a certain game.
“Consumers are making decisions based not just on the weather at the time of the event but even days before that,” Floehr says. “With our almost billion historical weather forecasts, we can supply data in an easily used form for machine learning and AI so that [clients] can train their models effectively.”
Then, they have more insight into attendance expectations and are able to plan in advance everything from how many ushers they’ll need for a game to how many hot dogs to order from a supplier, he says.
A national grid energy utility that ForecastWatch works with uses its historical weather information as part of its data sets to create models to handle people and resource placement when there is going to be a potentially impactful event – flooding followed by severe winds, for instance. “When the ground is saturated, it’s a lot easier for trees to topple over, and with a high wind event after that, trees have the potential to bring down power lines,” Floehr explains. Now the utility can be more accurate in preparing responses to impactful weather events.
Python’s Place in All This
The ForecastWatch service is 99 percent Python and open source, Floehr says. Python is pretty much the preeminent language of meteorology as an upward level language of manipulating and dealing with the output of models, he explains. At American Meteorological Society conferences, attendees can take short Python courses.
“Python is used extensively in the meteorology community as it will handle large amounts of data, analyze it, and basically manipulate that data to meet needs,” he says.
When ForecastWatch started up in the early 2000s, a lot of observation stations for weather were still using modems and communicating their results at the end of the day. But now, not only is there so much more data, it’s coming at everyone faster, too.
“It’s almost instantaneous. You get data and more of it in real time, and that has enabled lot of amazing things,” Floehr says – including, of course, the company’s services. But there’s still room for many businesses to understand that historical weather data can help them with their tactical and strategic decision-making. “Data is the new currency. More and more of it exists and so more and more decisions can be made using it,” he says.
Image used under license from Shutterstock.com