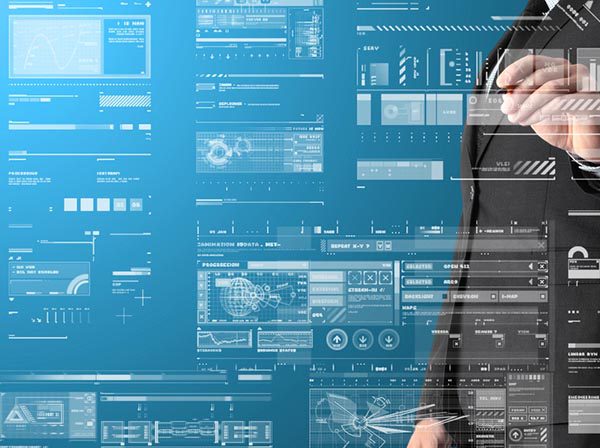
Artificial intelligence (AI), encompasses the broad fields of data capture, data storage, data preparation, and advanced data analytics technologies. In other words, AI systems are not limited to a single aspect of Data Management; rather, they are increasingly penetrating any and every aspect of business through allied data technologies like machine learning (ML), deep learning, (DL), neural networks, natural language processing (NLP), and others.
Every day, organizations are experiencing some change in business due to partial or full automation of systems, processes, and tasks — threatening to replace human labor. ML solutions have even started demonstrating that they are better than human statisticians in data preparation, thus challenging the humans in highly qualified, intellectual tasks.
A Forrester Infographic indicates that Data Quality (DQ) is one of the topmost challenges to successful implementation of AI systems in enterprises. According to Forrester analyst Michele Goetz, businesses lack a clear “understanding of data needed for ML models,” and thus struggle with data preparation in most cases.
The Impact of Data Quality in the Machine Learning Era shows that in an era of self-service analytics, Data Quality assumes more importance than before, as ordinary business users are not capable of detecting and rectifying corrupted data. The article stresses the need to address Data Quality issues within an enterprise data strategy framework.
Predictions from Forrester: Current Status of AI and Automation
Two recent Forrester Reports, indicate that businesses realize that they cannot fulfill their data-driven expectations unless the data is fit to be used with advanced AI-powered analytics systems. The current data challenge is that the data is “unclean,” thus making DQ the top concern for both the data centers and data service providers.
Forrester calls this controversy-laden AI buzz “irrational exuberance for AI adoption.” In most businesses, leaders and operators are struggling with poor Data Quality. This growing problem has significantly reduced business users’ faith in data-driven decisions. Forrester warns that the imminent transition in business will be a blend of AI with robotic process automation (RPA) to create a fully digitized work environment. but where is the matching human talent to make sense of all the outpouring data from this digitized environment? Can AI deliver digital Data Analysts?
Let’s get real about AI from OC & C Strategy Consultants provides the following market insights:
- Eighty-six percent of C-Suite executives agree that they have missed out on valuable opportunities by not embracing AI sooner.
- By 2025, 50 percent of jobs will involve 50 percent automation, whereas specific sectors like programmatic media will be 100 percent automated by 2025.
- The new entrants in global business will probably overtake their competitors due to early AI adoption.
- Global AI spending has been significant: $219 billion in 2018, with the U.S. alone accounting for $91 billion. The prediction is that AI spending will reach $400 billion by 2025.
- There is a direct link between AI adoption and business performance — businesses with AI have outperformed their competitors in all sectors.
Rumors are doing the rounds that by the end of this year, chatbots, RPA, and intelligent systems will jointly eliminate at least 20 percent of service-desk engagements. AI washing may also be something to watch for. A third trend is that most business owners are upgrading their existing AI capabilities in partnership with AI industry consortiums rather than with their current service providers.
PwC Predicts: Data Quality A Serious Challenge to Most AI Systems
According to the results of a Price Waterhouse Cooper survey conducted in January 2019, most large businesses now realize that in spite of piling up business and customer data over the years, they are severely handicapped to leverage advanced data technologies due to poor Data Quality.
The main purpose of AI up-gradation in any business is to reduce cost and increase profits, but that cannot be achieved given the “sorry state of current data stockpiles.” The current statistics indicate that while 76 percent of businesses aim to leverage their data to extract business value, only 15 percent have access to the appropriate type of data to reach that goal.
The main reasons provided by business executives in the above survey for failing to meet their data analytics targets were data silos, bad data, data compliance issues, lack of data experts, and inadequate systems.
Data Quality-related problems always surfaced in “historical data,” which may have been gathered from multiple sources with inconsistent standards and varying levels of accuracy. Apart from standardizing data formats, the PWC analysts also indicated that data privacy and data security must also be aggressively addressed to comply with regulations like GDPR.
Two important statistics included in the PWC survey report are:
- University of Texas researchers have claimed that an increase of 10 percent in data usability will boost “annual revenue” by over $2 billion.
- PwC survey respondents claimed that data cleaning will result in an average cost savings of 33 percent and an average revenue increase of 31 percent.
Enterprises Ready to Abort AI Projects due to Poor Data Quality
CIO reports that major enterprises are prematurely aborting their AI projects. These cost-conscious business owners realize that their investments will be wasted unless the data ecosystem is vastly improved.
For example, Arvind Krishna, IBM’s senior VP-Cloud and Cognitive Software, mentioned that almost 80 percent of work involved in AI projects is data preparation, and many businesses are still not ready to invest in that kind of data exercise. While interviewing with The Wall Street Journal, Krishna mentioned that the prospect of spending a whole year “collecting and cleansing data” prior to actually using any AI system for business benefits will be too much for most businesses. Although IBM is pursuing some 20,000-odd AI projects worldwide, the Data Quality issues are seriously hindering the speed of AI system implementations.
This case study includes the story of a retailer who used ML algorithms to improve their existing product and inventory Data Quality by around 30 percent. Data Management experts believe use of smart ML algorithms will vastly improve the quality of large data sets in future.
How Bad and How Much: Impact of Bad Data in Big Data AI Projects
The Smart Data Collective site offers an insightful peek into the current state of data used in AI projects, which indicates the following contribute to data-related problems in AI projects: the geographical source points, diversity of data input channels, diverse data types, data obtained from open markets, and data privacy issues.
Gradually, both the AI solution vendors and the business communities are realizing that not every data source or every data type is useful or good for ML algorithms to train on. Moreover, only so much data is “representative” of a whole set and not the whole dataset. In reality, most datasets contain inaccurate, duplicate, and missing data, which ultimately leads to wasted IT investments and reduced faith in data-driven decision-making.
Must-Know: What are Common Data Quality Issues for Big Data and How to Handle from KD Nugget offers an excellent critical review of quality issues in Big Data in terms of data volume, where the mammoth scale of data makes quality measurements an approximation game. In terms of data velocity, the tremendous speed of data flow and collection makes it difficult to gauge Data Quality in a real-time application. Thus, the concept of “near real time” has surfaced.
In terms of data variety, diverse data types cannot be measured by any standardized Data Quality metrics and metadata plays a strong role in quality assessments of disparate data. Lastly, in terms of data veracity, biased or inconsistent data often create roadblocks to proper Data Quality assessments.
Of the four Vs, data veracity if the least defined and least understood in the Big Data world. The KD Nugget post also includes some useful strategies for setting DQ goals in Big Data projects.
The growing importance of AI and ML in enterprise Data Management should not be overlooked. As of today, eight out of every 10 organizations engaged in AI and ML projects have reported their projects have either stalled or have been aborted. The truth is that nearly 96 percent of these organizations have faced Data Quality issues.
The biggest DQ issue currently challenging in-house AI projects is the lack of proper labeling of ML training data. Nathaniel Gates, CEO and co-founder of Alegion, a training data platform for AI and ML, said, “The single largest obstacle to implementing machine learning models into production is the volume and quality of the training data.”
The Role of Machine Learning in Data Preparation
Manual Data Quality assessment, cleansing and deduplication processes have gradually passed on the baton to rule-based automation, which uses Data Quality tools. Data preparation tasks take up more than half of the data managers’ and data scientists’ time. A transition in Data Quality process is noticeable from static rule-based approach to a dynamic, self-adapting, learning-based ML approach in various domains.
ML provides assistance in deriving a Data Quality index score to assess data sets’ quality and reliability in real time based on deviation from predicted parameter values. The true power of data can be unlocked when it is refined and transformed into a high-quality state where we can realize its true potential. ML has the potential to assess the quality of data assets, predict missing values, and provide cleansing recommendations, thereby reducing the complexity and efforts spent by Data Quality experts and scientists.
For IoT applications, the battle against Data Quality is most acutely perceptible now. Succeed in the Intelligent Era with an End-to-End Data Management Framework stresses on an end-to-end data-management framework to greatly enhance business decision-making.
Artificial Intelligence Can Take a Dive in the Absence of Data Quality points out that in the last 10 years, AI tools and processes have improved to the point where in addition to computing tactical tasks, machines “can also make strategic decisions and improve Data Quality.”
In fact, DQ issues must be tackled before businesses can expect returns on their AI investments. Successful AI systems need both high-quality and high-volume data. Data Quality & Data Governance can Maximize Your AI Outcomes asserts that the “predictive efficiency” of ML algorithms depends largely on the variety, volume, and quality of data used for such models.
Image used under license from Shutterstock.com