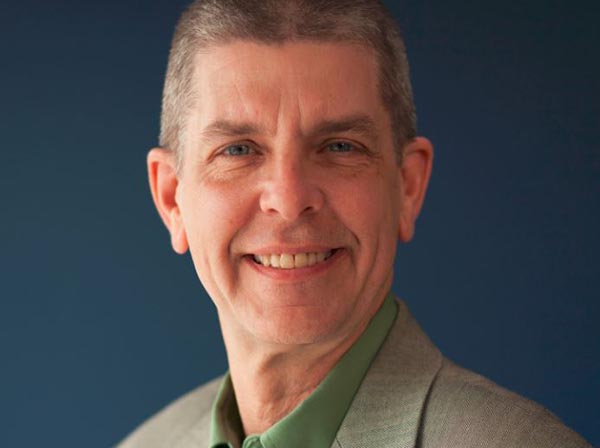
Click to learn more about author Harald Smith.
There’s a tendency to view the cloud, with its promises of continuous delivery models and built-in advanced analytics, as a platform that will reveal fresh and important business insights simply by using it. This is only partially true: Certainly, many organizations have benefited from cloud use. But just as Hadoop was our silver bullet about five years ago, there’s a tendency to see promising emerging technology as the answer to a complex data challenge.
In reality, preparing data for use in analytics still requires working through ever-increasing volumes and varieties of data – and understanding the data you are working with. You can take advantage of these platforms only when you understand the business problems and processes, as well as the data requirements and data available to help address those problems – not to mention put in place the people who understand the data and its context, and the processes to help these people validate, vet, and leverage that data.
The More Things Change …
Going from on-premise solutions to cloud-based platforms changes neither the data utilized nor the data produced. If you deliver garbage data to your cloud applications, you still have the same Data Quality problems as before. Whether that data is moved within the cloud, or between on-premise systems to/from the cloud, there are challenges in ensuring that data ends up in the correct format without loss of fidelity or context.
As we’ve seen with other Big Data platforms, the volume, velocity, and variety of data continues to increase, and is not shrinking with movements to cloud. IDC noted in their 2018 report on Copy Data Management that replicated data will cost IT organizations $55.63 billion in 2020, furthering an earlier report that organizations average 12 to 15 copies of any given data source. Michael Stonebraker’s keynote speech at the 2019 Enterprise Data World conference referred to the ever-increasing volume and variety of data as the “800-pound gorilla in the room,” where a typical enterprise may have thousands of operational systems and data stores, not including data replicated into spreadsheets.
If anything, the “elastic” nature of cloud platforms encourages continued expansion of data volumes, with cloud storage currently growing around 20 percent annually based on current market reports. The ever-growing number and copies of data sources makes it increasingly difficult to determine which data is correct, who’s using which source, and whether the copy is exact or modified from the source – all issues of provenance and lineage that the data analyst must work through.
An added twist with cloud applications is the continuous delivery model following Agile methodologies. In the continuous delivery model, applications are regularly updated, potentially every day, and often transparently to the user. We experience this with websites like Amazon. Unlike applications run on-premise where you control the pace of change and rigorously test integration points, you may be completely unaware when cloud applications are modified. If a cloud application modifies the way it structures and handles data, often to accommodate newly implemented features, the integration points in or out of these cloud applications may be blissfully ignorant of the changes, and allow new Data Quality issues, often with data structure and consistency, to emerge.
The More Things Stay the Same …
At the same time as we’re seeing increasing shifts to cloud-based platforms, we’re also seeing more Data Quality issues emerging as data scientists apply this growing volume and variety of data to organizational business needs. A new survey indicates nearly 80 percent of AI and machine learning projects have stalled due to issues with Data Quality and proper labeling of data attributes. This survey also noted two-thirds of respondents cited “bias or errors in the data” as the most common issue and half reported “data not in a usable form.” And where we have Data Quality issues, moving the data to the cloud and replicating it further simply propagates the Data Quality issues further along the data pipeline in more and more instances.
The Ongoing Need for Data Literacy and Data Democratization
This brings us back to the need for a data-literate culture, particularly around the meaning of quality data. With our use of higher volumes and greater variety of data coming at us more frequently, though, there are additional characteristics we need to address and add to our “techniques” to be data-literate.
Context becomes critical. A set of data that works just fine in operations to ensure goods are shipped to customers does not necessarily become a good set of data for training algorithms for advanced analytics. Such a data set simply tells us what our customers bought in the past. But is this data complete for all customers and all orders through all channels? Is it continuous, or are there gaps in coverage? Does this data tell us anything about returned items or customer complaints?
Someone needs to be able to answer these questions and provide context to use the data. Where knowledge of the data is limited or in specific silos within an organization (or outside it if bringing in other third-party data), either roadblocks emerge (stalling projects) or questionable data enters into the projects, systems, and data stores, leading to poor-quality models and/or outcomes that no one trusts.
Where such human decisions are needed, you need enough people knowledgeable about the context of the data who understand what they are observing from their data profiling and analytics tools. For people to be effective at the scale that our cloud-based data is progressing to, where data is increasingly large and distributed, they must have knowledge of how to use and take advantage of the tools at hand to investigate and analyze data, often in ways that may not be immediately obvious. This depends on establishing a data-literate culture including people, process, and tools. And these three elements do not exist in isolation. To ensure people become data-literate, they must be included in processes and communications that help them become informed and help them share what they know and have learned.
For example, matching or entity resolution technology is a foundation for most Data Quality tools, yet typically embedded into data integration, ETL, or MDM processes. Most people are unaware of its existence in organizations, let alone how to use it. Yet matching functionality can be used to connect data together during data preparation steps for AI and advanced analytics and resolve a number of typical Data Quality issues. Further, matching functionality can also be used as an evaluation and quality assurance tool to test multiple relationships, determine whether data is duplicated, look for inappropriately linked records, and find unexpected data correlations.
Building out a “library” of knowledge about data, processes that use data, and approaches for using the tools and techniques at hand for data analysis are central to developing a data-literate culture that is truly democratized even while organizational data is distributed en masse into the cloud.
Thinking about Data Quality broadly, and how it is a core skill in your arsenal for data literacy, helps you adopt emerging technology such as cloud more effectively. Whether working with traditional reports or looking for new business insights, building an understanding of the tools to assess your data, particularly as it moves into new cloud platforms and applications, is a key step toward data literacy. Contributing to a broader knowledge base is a further step in helping others within your organization gain the right vocabulary and context so they can confidently appraise the data they’re using. That democratization of data knowledge and skill is critical if we are to discern what is fit for our specific business purpose, with the right quality and freedom from bias, and provide that discernment at scale.