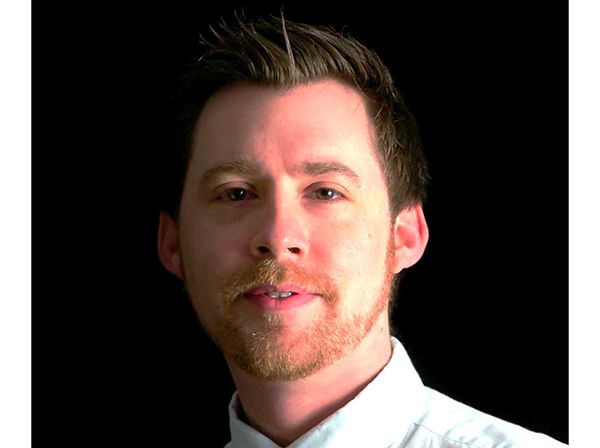
Is there data bias in your business? Recent research indicates that 65% of business and IT executives believe there is currently data bias in their organization, 13% of businesses are currently addressing data bias, and 78% believe data bias will become a bigger concern as AI/ML use increases. What this indicates is that businesses and organizations simultaneously worry while at the same time seeking a path forward to solve these data bias issues.
Bias is a part of our DNA – the human experience. Creating technology that will impact us on a global level may fundamentally change how we live our lives. These biases have the potential to do great harm, at scales we have not seen in nearly 100 years or may never see in the totality of human experience.
Where Does Bias Come From?
We live our lives based on how we take in the world. At a fundamental level, our evolved brains have in them hardwired pattern recognition, fear-based situational response, and conditional survival traits – all biases that we cannot escape. As we mature, many of these biases are for the most conquered, or at least heavily controlled by the more developed parts of our brain – the limbic brain, sometimes called the mammalian brain, which is thought to be 250 million years old.
The “higher functioning” neocortex or neomammalian brain is believed to have evolved just some 500,000 years ago, while the part of the brain handling language evolved about 70,000 years ago. It is here where we learn to control our bodies for fine-motor skills, communication, planning, forethought, etc.
These skills do not operate in silos but rather complement our experiences, developing patterns in our brains to help us see, hear, touch, smell, and taste the world around us. They help us see shapes on a head as faces, hear cockerels crowing denoting morning, etc. All of these patterns are based on the sensory information we receive – data if you will. These patterns allow us to better navigate our world, taking mental shortcuts to conclusions, of thought or experience. They build on our genetic biases, becoming internalized psychologically systemic biases.
Human Bias in Data
So, when we talk about creating artificial intelligence (AI) that aligns with “good” human morality and working towards “good” human goals, the data used to train any AI must have human-driven “correct biases” and remove biases found in data, even before it reaches the AI model to learn.
However, to do this at the scale required to train any AI, whether it is an LLM, OpenAI’s ChatGPT, or open-source AI, requires a significant volume of data. For instance, ChatGPT needed the whole internet, well up until 2021, to get to where it is today.
The problem with that level of data lies in the potential and significant risk from the number of inconsistencies, conflicting data, and erroneous or errant data posed in getting your AI to align with your goals, and perpetuating bias in your data, and AI findings.
Human Intelligence at AI Scales
You need a human eye on the data, business, and technical human expertise to validate and ensure your data is fit for consumption by the AI. All organizations seeking to capitalize on AI must solve or remove this problem or reduce the likelihood of error made by the AI, in addition to using human intelligence to again remove or reduce the bias found in data.
The scale of the data required to provide human intelligence at that level is simply unsustainable, perhaps even impossible. Organizations should look at a niche application or one where the number of available human experts is too low. Thus, organizations need a data platform that can bring human intelligence at the scale of an AI.
A stack of mission-critical application-developing technologies is one solution. For example, finding an agile, scalable, and secure combination of a data platform, a semantic AI technology, and a business rules engine is a strong approach.
With these as foundational or complementary technologies in your tech stack, it is possible to ingest, harmonize, and curate the data into the data model needed. Classified by human-led intelligent rules, semantically linked to taxonomies and ontologies, with fact extraction to the element level, this solution brings context, meaning, and insight to your data, while giving it an auditable trail to ensure your data meets bias standards or other regulatory or business standards, internal or external. The ability to apply eligibility and accuracy rules with human-led domain expertise before the data reaches the AI is powerful.
This means that you can identify biases, remove, or “improve” the bias in the data, or identify any shortfall that can lead to bias, all before reaching your new AI technology. This offers the best chance of AI being accurate and performant, and removing as much bias from the decision-making process that the AI might go through.
The Future of Data Bias
Will this stop bias in AI completely? That is impossible to tell. Remember that our intelligence was built over hundreds of millions of years, and still, we make mistakes, hold unconscious or conscious biases, and we still do not know how our brain works to make this intelligence. Over the years we have already made mistakes with technology. We unwittingly scale beyond a person’s ability to properly manage or adopt the technology, our society’s ability to regulate the impact of these global technologies, or simply lack the awareness of a technology’s capacity to affect us on the global level.
With AI, we have had 30 years of development, a picosecond in evolutional terms. So, we will make mistakes, but if we use the right tools, perform the right research, and enable alignment, then AI will become a powerful tool for businesses and large organizations the world over. We must remain conscious of any biases we train into it, even with data as thoroughly vetted as the example tech stack above can make it.