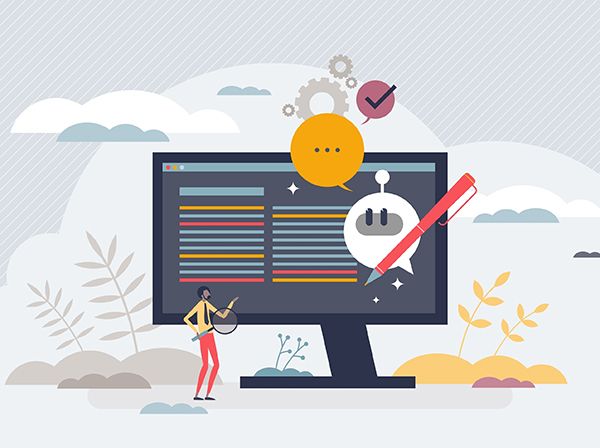
Generative AI – specifically ChatGPT – has taken the world by storm. From writing about AI in a Shakespearean style to basic programming, ChatGPT promises to disrupt existing workflows and reengineer daily life.
In the meantime, companies face another revolution with generative AI. They can remove all the data fed to ChatGPT, then replace it with a company’s data to train the generative AI. How would this capability to leverage specific corporate data transform business?
To find out, DATAVERSITY® interviewed David McGraw, senior manager in consumer and industrial products at West Monroe. David is a recognized leader in digital manufacturing transformation with a deep understanding of data science, data engineering, and cloud architecture. Below, he shares his thoughts about generative AI and how data will rule it.
What Is Generative AI and How Does It Work?
Generative AI refers to the machine learning (ML) algorithms that create new content by making decisions based on statistical models. Two products, ChatGPT, which creates text from data, and DALL-E, which creates images from data, have raised public knowledge about this type of AI.
Before generative AI became famous in 2023, the technology had existed for quite a while, notes McGraw. This type of AI works from a generative pre-trained transformer (GPT), a set of algorithms that use reinforcement learning on available data to recalculate and respond to a human’s prompt. For more technical details, read here.
ChatGPT got attention, according to McGraw, because OpenAI trained a GPT model on a large volume of data from the internet:
“Any company using generative AI technology with similar models and the same dataset as ChatGPT will see its products converge to match ChatGPT outputs. In the past, the models themselves were the IP, but with these generative AI models as a service, the new IP is the data.”
Data as the New IP
Consider the steps to get information about visiting a city. First, go into Google or another tool, enter your query and get a list of websites, internet protocols (IPs) describing different tourist attractions in that city.
Compare this process to finding information through ChatGPT. First, ask ChatGPT about what a visitor can do in a city. Then, get a couple of suggestions from the AI.
What is the difference? With generative AI, as McGraw describes, the data owned and used by a generative AI model becomes the new IP, not different website addresses.
Suppose any generative AI model receives good data from which to learn, and a person understands how to get results from that product. In that case, a person can find the information all in one place instead of distributed across multiple businesses. McGraw said:
“Have a generative AI model to summarize a large article and present several alternative responses. Then, a human reads that paper and selects the software’s best summarization, rewarding that program through that feedback data. Repeat this task often, and the model keeps searching for the most rewards.”
The AI learns how to predict what it will communicate next, then does it.
Using Generative AI to Profit from Your Data
Making data as a new IP means businesses profit directly and indirectly from their data inputs and prompts given to an AI model, explained McGraw:
“If companies train models that use the generative AI technology on their data, they can hyperfocus the modeling to answer questions relevant to [their] business objectives. So, for example, enterprises can get models to answer questions about daily operations, opening all sorts of possibilities for automation.”
Companies that leverage their data through generative AI save time and money through:
- Boilerplate writing: Automatically generate blog posts about products, services, or other subjects. “Companies can ask their personalized AI assistant to write different blog posts,” said McGraw. “Then, they can dive deeper using prompt engineering, deliberatively selecting what question they type in their generative AI interfaces. Also, organizations can keep refining their querying to update current web posts.”
- Customer service: “Have generative AI models at that first level of support after training it on the corporation’s data,” said McGraw. “Let it answer frequent calls from customers.” Customer support could have more time to dig into challenging and complex issues.
- Low-level programming: Generative AI has successfully done repetitive, low-level code. So start-up organizations have more resources to build a product, and their developers can concentrate on the heavy lifting.
- Better web experiences: “Some of the earlier bots on web pages have not provided a great personable experience,” said McGraw, noting that these computer programs use a manufactured queue to process questions and generate responses. “With generative AI models, specifically ChatGPT, people get a very personable experience. They don’t have to mention questions asked earlier in the conversation. Instead, ChatGPT knows that the topic has stayed the same. Then the user almost feels they communicate with a human instead of the AI.”
Additionally, companies can sell their data. McGraw hypothesized that “all companies have some data which is extremely valuable to someone outside the organization. As enterprises run their businesses in the future, they will hunger for quality data sets to feed their generative AI.”
McGraw would not be surprised if businesses in Silicon Valley sit down at the same time as you read these words, discussing what datasets to purchase for AI training and use: “Many companies will want to purchase specific data sets from as many companies as possible to train their AI towards a specialty.”
A Human Must Stay in the Loop
While generative AI promises higher productivity, it relies heavily on humans for guidance. Since GPT can get incorrect answers from the data it consumes, its learning style, and its decision-making capabilities, humans must stay involved throughout the process of AI’s creations.
Moreover, when generative AI goes rogue, it causes significant problems and stressful interactions. Given these limitations, humans in organizations need to step in to:
Collect data for AI training: “Generative AI requires a specific format and quality to complete training,” said McGraw. Consequently, enterprises need to think, “How do I collect my data going forward so I can train my AI?” and plan these tasks:
- Within the company: Humans must decide what data a company collects and how it does so.
- Outside the institution: If companies need to run their AI models on another person’s infrastructure, they need to know how and what to do when the website owner changes the data it presents – “for example, turns off some data sets,” said McGraw.
Train the AI models: People in companies need to consider when an AI model completes training and what the limitations are to that training.
Ensure AI returns good responses: Humans must play multiple roles to ensure quality responses during AI training and when the AI software enters the marketplace.
Prompt engineering: People must determine the best questions to ask AI for good responses and to explore information through it.
Quality assurance: Humans need to check out and test out generative AI models for:
- Accuracy: Check that the responses generated are correct.
- Good user experience: Check engineered prompts from a human lead to a personable and pleasant experience.
- Legal compliance: Ensure proper data ownership and that any data collected and returned is legal and respectful of data privacy.
- Impartiality: “AI software gets more biased upon reusing the same inputs multiple times,” said McGraw. This can lead to inaccuracies as contexts around the inputted data change. So, people need to know when companies should refresh the inputs or train the AI to get more impartial information.
Get advertising revenue: “If people turn to tools like ChatGPT over Google search, what happens to the marketing revenue spent for Google?” asked McGraw. As a result, companies need to figure out new marketing strategies for generative AI interfaces.
Deal with pushback: “Some workers will fear that generative AI could replace them and react negatively to its adoption,” said McGraw. People will need to figure out how to implement changes with AI and how to handle the politics around that.
Conclusion
As companies become enamored with and use generative AI, people will see significant business productivity and challenges, especially while hyper-focusing the AI model on their data. Data will increase in the domination of the generative AI space and will require companies to mitigate potential risks and to hire people with generative AI knowledge.
In the meantime, generative AI will get smarter and work more seamlessly in existing applications. So, expect to have many more productivity tools.
Most importantly, McGraw said,
“Data will become more valuable than people ever anticipated. Technology companies with that hunger for data can potentially integrate vertically. For example, would Google purchase pharmaceutical companies solely for their data? It could then use this data to give specialized expertise to ChatGPT derivatives.”
As time goes by, this type of scenario seems more realistic, so expect the race for data to intensify.
Image used under license from Shutterstock.com