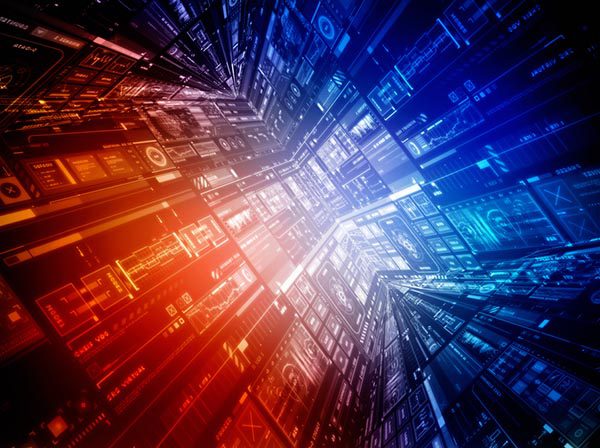
Data Management, as a principle, requires that data is brought to a single place, governed actively, and available in real time. It also includes the trust needed for business users to perform day-to-day business functions and to do it better than the competition.
Data needs are contextual and based on the role of the user. Marketing wants to understand the customer from a use case perspective to better target potential customers. Finance wants to know that product warranties are being satisfied. The sales department wants to determine how to sell more units to existing customers. “Everyone wants a different view of the same entity, and across multiple different factors,” said Ravi Shankar, Senior Vice President and Chief Marketing Officer with data virtualization company Denodo. Shankar spoke with DATAVERSITYÒ about the interconnections and challenges between Data Management and data virtualization for organizations today.
Data Management brings together all the data from different sources, and creates a trusted view of that information, made available to the business in real time, so as the business is running and gathering the data, it is immediately available for business users to consume.
However, as data comes in, not all of it needs to be made available to all users. Due to regulatory constraints about data use, the company may want to be able to audit the data and control access to sensitive information, as well as protect the source of production. “The beneficiaries of data in any company are the business users. For them, the data needs to be reliable, instantly available to them, and secure,” he said.
Fighting Gravity
Data integration collects data from multiple and varying storage systems and makes it available to consuming systems. Extract, transform, and load (ETL) is an approach where data is batched and moved from the operational systems into a data warehouse, commented Shankar.
Other forms of data integration similar to ETL bring data from different sources and collect it into a central repository, which duplicates the data, Shankar said. “It creates a latency for data consumers and a host of other problems,” particularly in industries such as healthcare, where latency can have a serious impact.
In the context of today’s massive data stores, these older tools have been fighting against “data gravity” (a concept coined by Dave McCrory), which compares data to a planet or other object with sufficient mass to exert a gravitational pull. Shankar said:
“As data accumulates (builds mass) there is a greater likelihood that additional services and applications will be attracted to this data. This is the same effect gravity has on objects around a planet. As the mass or density increases, so does the strength of gravitational pull. If large enough, data can be virtually impossible to move.”
Will Ochandarena, in an article titled The Cutting Edge: Data Fabric, Driverless, and Data Gravity, illustrates it as:
“Data gravity is the phenomenon that happens when datasets get so large that it becomes physically impossible to move and creates a ‘pull’ of applications and analytics towards it.”
Shankar said, “We have been fighting against the pull of gravity” because of this process of trying to centralize data — which has been the standard for the last thirty years. In the 1980s, we tried to centralize data into a database; when those databases started multiplying in the 1990s, we started centralizing the data in data warehouses, which started multiplying as well, he said. Then the enterprise data warehouse emerged, and that solution worked for structured data until the advent of unstructured data, at which point the need arose for the data lake.
Data gravity tends to be at the source locations, he said. We understand the value of unified data, but to continually pull the data away from the source in order to have that unified view is a constant battle, especially with the size of today’s data stores.
Data Virtualization
Data virtualization finds the data where it resides and provides a unified view for data consumers. It doesn’t collect or duplicate the data, yet consumers can get the same insights and the data stays in the same location.
According to Shankar, data virtualization offers four key benefits:
- A logical data layer, providing a virtual approach to accessing, managing, and delivering data without replicating it in a physical repository.
- Integration of data siloed across all enterprise systems, regardless of data format, location, or latency.
- Data Management via a centralized secure layer to catalog, search, discover, and govern the unified data and its relationships.
- Delivery of integrated information in real time to the applications used by business users.
Shankar said that the biggest benefit of data virtualization is the abstraction provided by having a logical view, because it liberates business users from the changes in the underlying technology. No matter whether data is moving from system A to system B, from on-premises to the cloud, from a data warehouse to the data lake: “The logical layer can go get the data wherever it is. For a business user like me, that’s a very important benefit.”
Where Data Virtualization and Data Management Intersect
One of the most important needs for business users, given the variety and volume of data across the enterprise, is the ability to find a specific customer within the company.
“I want to have a 360-degree view of that customer’s associations with our business entities, the products we’ve sold them, the warranty, as well as household and billing information.” Shankar said that in that respect, data virtualization has evolved into more comprehensive Data Management by cataloging a lot of the business data and definitions and easily providing that information to the business user.
Steps to Data Virtualization Success
Shankar said the first step is to have an understanding of all the data that needs to be brought together for a particular business function. Part of that arises from knowing the unique problems the business faces. “In all cases my business is going to be interrupted, so how do I modernize my architecture without the business being impacted?”
For example, some companies may be migrating from on-premises to the cloud, for example, whether that entails buying new technology in the cloud, or rewriting existing applications. These organizations will want to keep the data in their sources—whether on-premises or in the cloud—and use data virtualization as an abstraction layer to hide the complexity of the migration from the business users. “Once they understand the problem they are solving with data virtualization, it is very easy to get the technology up and running,” he said.
Shankar advocates starting with one department’s systems, logically integrating the data, and quickly making the data available to the business users to show the ROI, and then expanding from there.
His customers say that the biggest benefit of this approach is the flexibility the technology provides. “It’s adaptable enough that they can make it work in phases while saving in terms of the number of resources needed.”
Challenges: The Importance of Governance
Although the actual implementation of data virtualization can be accomplished relatively quickly once the business knows what it wants to accomplish, Shankar advises his clients to put strong Data Governance in place early in the process. “You have to understand who owns the data, who consumes the data, who can make modifications to the data, and how to make the data available within the confines to the business.”
The challenges for his clients are not so much in terms of the technology, but rather in the organizational aspects of defining control surrounding the use of technology, and that’s where governance comes in. “Quite often I see technology projects being delayed for months because they do not have a sound governance framework, and I would advocate putting that in place before you actually start the technology work.”
Denodo
Denodo is a leader in data virtualization providing agile, high performance data integration, data abstraction, and real-time data services across the broadest range of enterprise, cloud, big data, and unstructured data sources at half the cost of traditional approaches. New enhancements in development involve the integration of AI and machine learning to support business decision-making processes.
Image used under license from Shutterstock.com