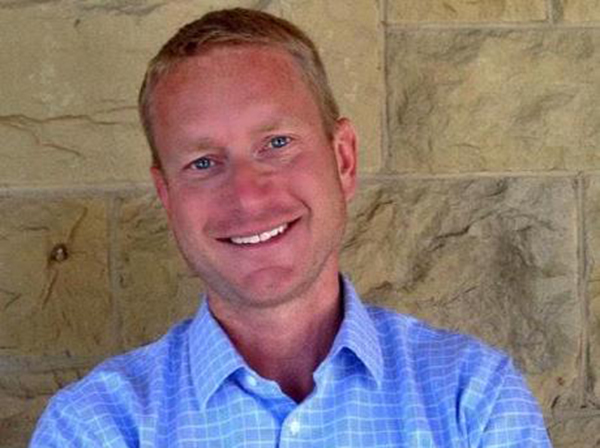
The ways in which we store and manage data have grown exponentially over recent years – and continue to evolve into new paradigms. For much of IT history, though, enterprise data architecture has existed as monolithic, centralized “data lakes.” More recently, as the role of data evolves and changes, so too does where that data is stored within your business. Increasing volumes of data have led to changes in storage architecture, accompanied by myriad processes surrounding data storage and retrieval. And the sheer volumes of data being created and captured mean that from an IT operations perspective, data management is an area that requires constant attention. The upshot is that tracking where exactly your data is housed can prove challenging.
Evolving a new paradigm means recognizing if your organization operates with a storage mindset or a story mindset when it comes to data. Are you collecting data and allowing it to go dark without a clear purpose, or are you actively identifying, capturing, and uncovering the stories data can unleash? We know that where there is data there is opportunity; the question is how to unleash that opportunity. A thorough answer to that question requires many different perspectives. In the past decade, domain-driven design has revolutionized the way we approach application modernization; however, data platform modernization has yet to benefit from an equivalent pattern. Looking at Data-as-a-Product (DaaP) can help.
In a Medium post in 2019, Justin Gage, data leader at Retool, had this to say about DaaP: “Data as a Product is the simplest model to understand: the job of the data team is to provide the data that the company needs, for whatever purpose, be it making decisions, building personalized products, or detecting fraud. This might just sound like data engineering, but it’s not: data scientists also provide data as a product, just packaged in a different way.”
The DaaP principle allows you to address issues of data quality and silos – or, as Gartner calls it, “dark data” – “the information assets organizations collect, process and store during regular business activities, but generally fail to use for other purposes (for example, analytics, business relationships and direct monetizing).” Domain data is treated as a product; consumers of that data are treated as customers. Moreover, DaaP identifies existing patterns that align perfectly with extant domain-driven approaches and can be implemented today. The result: a perfect symbiosis.
One of the hottest new approaches to DaaP is data mesh, the Next Step Up from Big Data; but what exactly is it?
As defined by Zhamak Dehghani, the original architect of the term, “a data mesh is a type of data platform architecture that embraces the ubiquity of data in the enterprise by leveraging a domain-oriented, self-serve design.” In simple terms, data mesh is the idea that instead of keeping all your data in a single silo, with no clear ownership, you create a “federated design,” which then acts as the foundation to all your data products. This is further linked through data governance standards, which then enables collaboration across multiple data domains.
Data mesh provides the distributed data architecture and team models required to liberate data at scale and support analytics, machine learning, and data-powered digital products. It’s a useful model: It allows data leaders to focus and align with business objectives, on the why and how. It allows them to address what problems businesses are looking to solve around data management within their businesses, and how they can go about solving those problems.
The key principle is that data and the owning teams should be organized in a way that aligns with the business domains that support the digital experience or analytics use cases. Data teams are no longer central and purely IT-focused; instead, they are defined around those who know most about the domain to acquire, transform, document, catalog, and ultimately provide this domain data back to the business to power these new digital products and analytics use cases. There is no longer a central data lake to serve analytics use cases. Business decision-makers all get direct read-only access to data from each domain to use as needed, as the source of the truth. Though the domains have distributed ownership, the data platform technology does not; it exists on a common cloud or hybrid cloud infrastructure. By fully embracing this approach you can incrementally create your data mesh.
The fruits of the data mesh tree are easily harvested with strategic planning, and collaborative, creative thinking. Looking at data optimization through more than just the IT lens and examining the cultural model around the complex software stack is where the true value of data lies. The frictionless collaboration that has been achieved in managing the modern software stack can also happen with data. The key here is to fully secure internal buy-in from all stakeholders – which in turn requires the ability to demonstrate the value of data.
Nothing demonstrates the value and power of data more than tangible outcomes, and for this, you need a model for establishing data maturity – or in other words, delivering value generation in short order and building conviction around a scalable, production system. For example, can we show reduced customer churn? Or reduced customer acquisition costs?
At its foundation, this means starting with a modern cloud data platform that can manage high-priority workloads, while simultaneously allowing federated and domain-driven access to the data mesh. All data and its derivatives (prediction, recommendation, and explanation) are packaged up and delivered to relevant departments and team members in an immediately accessible way, facilitating effective data management. Building on this, the cultural and human aspect of data governance must drive trust through regulatory compliance, data quality, completeness, and discoverability with a priority on high-quality insights.
As part of your modernization effort, you may also be considering a new analytics use case, modern workplace, or customer-facing digital product. This can be your forcing function to get going with a data mesh. By bringing together business domain expertise, data architecture, infrastructure, applications, and the organization’s core principles, you can make a success of this first product, iterate continuously, and align your approach to support the target end state.
Modernizing your data platform is a huge first step, but the journey from storage to story and a modern data architecture to compete with the digital natives is right in front of you. The holistic nature of data mesh overcomes one of the biggest shortcomings of data lakes: how they place data in silos. It’s a major barrier for businesses, despite the fact that many organizations can show cross-domain data success. The real business benefits of data are how it can help drive changes at scale and removing data lake silos helps create this singular value. Looking at data mesh and data as a product in the right way can lead to a powerful data mindset.