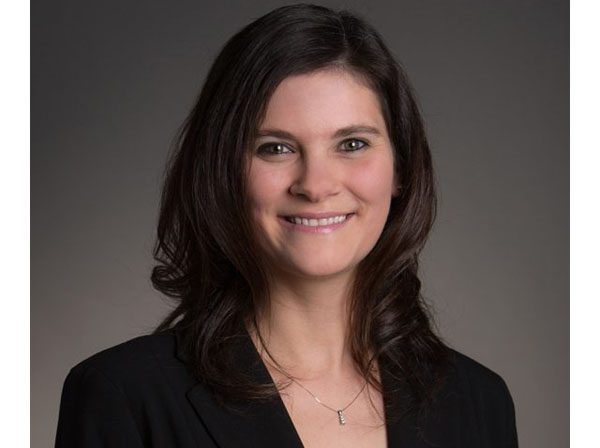
Click to learn more about author Emily Washington.
Each year we undergo significant transformation in the information technology (IT) industry. However, 2020 is a year for the history books.
Evolution in IT usually happens organically. As priorities change, so do technologies, processes, people, and techniques. When the term “big data” became mainstream last decade, IT departments tackled data projects on a limited scope.
For example, if a sales team needed information on potential prospects, IT would establish a one-off project siloed within sales, gather the information, and utilize a basic Data Quality tool to quickly measure data’s accuracy, consistency, conformance, etc.
Fast forward a few years. Data Quality concerns grew as the volumes of data increased. Still, organizations only prioritized Data Quality when revenue, reputation, or mission-critical data were at risk. However, over the last few years, complex, global regulations and the increased speed and scale of data have prompted organizations to rethink their Data Quality efforts.
Addressing Data Quality issues on a larger scale started to become a priority beyond. Leading-edge companies realized that merging Data Quality efforts with enterprise Data Governance initiatives encouraged cross-departmental collaboration to proactively resolve Data Quality issues and enhance enterprise data value.
However, it was not until 2020 that companies were forced to reimagine Data Quality technologies, processes, and procedures on the fly. And as these organizations started to grasp the relationship Data Quality has with Data Governance, many have changed how they operate.
The Unforeseen Evolution of Data Quality in 2020
At the start of 2020, many organizations have fully realized that using Data Governance to align people, processes, and technology is key to establishing data trust — and that what’s essential to data trust is Data Quality.
As data moves across an enterprise, exposing itself to new processes, uses, and changes, it transforms, and so does its quality score. By working together to monitor and score Data Quality directly within a governance framework, organizations can now implement Data Quality controls and provide end-to-end Data Quality from data creation through ingestion.
When organizations use Data Governance to engage multiple business units in Data Quality efforts, they build trust among users who leverage data to accelerate their business strategy. However, thanks to COVID-19, collaborative Data Governance initiatives hit a speed bump in March 2020.
No one could have foreseen the extent of a nearly 100 percent move to remote work or the need for rapid adoption of Data Governance initiatives. Once removed from physical proximity, communication between IT and diverging business units often suffered, reducing Data Quality awareness.
When business users can’t find the information on their data’s quality, they reach out to the IT department. While IT resources are more advanced than five years ago, they don’t always have access to the same set of tools and information they need to understand and resolve Data Quality issues from home.
As 2021 approaches, organizations need to look for self-service Data Quality technologies that make it easier for data users to find, understand, trust, and collaborate around their data issues.
Managing Data Quality in 2021
In 2021, organizations will unite Data Management to streamline data challenges. For example, they are combining Data Governance and Data Quality and data lineage and data catalog tasks.
Using Data Governance to create a centralized data catalog makes large quantities of data available to a company’s workforce in a remote work environment.
The data catalog empowers IT teams to easily manage policies, communicate data access methods, and convey usage restriction rights from anywhere. As a result, business users simply consult the data catalog to find the information they need.
Most importantly, the data catalog includes Data Quality metric baselines. By tracking and documenting data lineage visualization in a catalog, organizations can monitor and measure Data Quality scores from data creation through multiple transformation and final consumption. Socialization and collaboration features then provide transparency into Data Quality scores and empower users to discuss which data is best suited for their purposes.
Creating an enterprise-wide Data Governance framework that merges Data Quality and data lineage capabilities into a robust data catalog empowers data users to quickly locate detailed data information, including data flow and dependencies, business meaning, uses, and quality scores. As a result, businesses will have high-quality data to generate insights, ensuring business success now and in the future, while continuing to evolve the way we work.