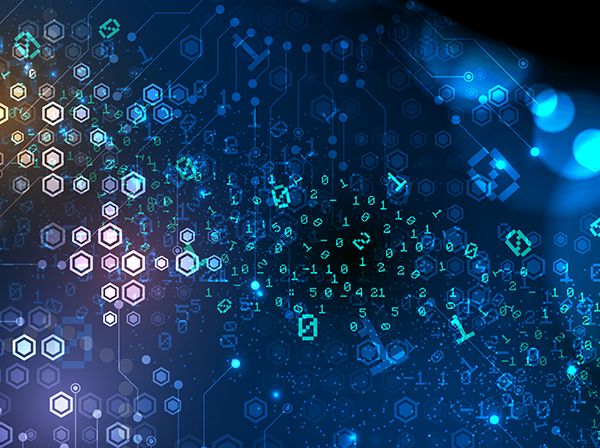
In the data economy, data is king. Today, any business – small, medium, or large – thrives on its data assets. The recent trend of offering data-driven insights as a service has opened up a profitable revenue channel for businesses. Cloud computing and hosted analytics have brought data-as-a-service to the desktops of ordinary business users, which was unheard of even a few years ago. As the global business environment is fast moving toward all things digital, artificial intelligence (AI), machine learning (ML), and deep learning (DL) will play as important roles as Data Science in reshaping businesses across the world. This article will highlight the connections between Data Science vs. machine learning vs. AI.
The evolution of Data Science and machine learning in the age of AI has been marked by significant advancements in technology and computing power. Data Science, which involves extracting insights from large sets of structured and unstructured data, has become a crucial component of modern business operations.
As AI continues to evolve, Data Science and machine learning will likely become even more critical for businesses looking to stay competitive in an increasingly complex digital landscape. Data Science, machine learning, and AI are increasingly used to improve decision-making and gain a competitive edge.
Understanding the differences between Data Science vs. machine learning vs. AI is crucial in today’s digital world. Data Science is the process of extracting insights and knowledge from data using statistical and computational techniques. It involves collecting, processing, analyzing, and interpreting large datasets to derive meaningful information.
On the other hand, machine learning is a subset of AI that focuses on building algorithms that can learn from data and make predictions or decisions without being explicitly programmed to do so. It uses statistical models to identify patterns in data and improve its performance over time.
In such a data-centric business environment, it is only normal to expect newer and better data technologies in the global IT market, threatening to displace human data scientists and business analysts in the near future.
From Data Science to Machine Learning and AI: The Technology Transition
The term “Data Science,” which is often trending on technology news sites, combines principles of mathematics, statistics, computer science, data engineering, database technologies, and more. Data Science may be viewed more as the technology field of Data Management that uses AI and related fields to interpret historical data, recognize patterns in current data, and make predictions. In that sense, AI and subsets of AI like ML and DL aid data scientists in accumulating competitive intelligence through insights from data stockpiles.
AI can be defined as a broad scientific field with many sub-disciplines – all collaboratively working toward automating business processes and enabling machines to behave more like humans. Fields like ML and DL, though offshoots of AI, have made intense penetrations into the territories of neural networks, thus pushing Data Science into the next level of automation where voice, image, text recognition, and virtual reality have merged to create an awesome digitized business ecosystem. Newer technologies related to the basic practices of Data Science and AI are still evolving every day, and now with big data, cloud, IoT, edge, and serverless – who knows where it all ends?
The Digital Journey That Does Not Seem to End
Data Science, which remained hidden behind on-premise data centers, suddenly started gaining tremendous visibility throughout the enterprise – all due to the emergence of AI. The overnight transformation of business processes and day-to-day decision-making, fueled by big data, Hadoop, and the rise of social, mobile, and IoT channels, brought data technologies to the forefront of business operations. Today, data rules in business, and this trend will not diminish in the foreseeable future.
“Data Science” is the more holistic term encompassing the “collection, storage, organization, preparation, and end-to-end management of data,” while AI-enabled technologies control and facilitate data analytics in business operations. Data Science, artificial intelligence, and machine learning work in tandem to exploit data for a wide variety of business benefits.
A blog post from mygreatlearning.com compares Data Science with AI and ML. The marked difference between Data Science and AI-enabled data technologies? Machine learning and deep learning algorithms train on data enabled by Data Science, to become smarter and more informed in giving back business predictions. In that sense, Data Science and AI share a symbiotic relationship, a complete give-and-take in both directions.
Contrasting Features Between Data Science vs. Machine Learning vs. AI
Though Data Science is an interdisciplinary field, when data scientists enter the realm of data analysis, they begin at the top automation level of AI. Then, they work their way down to DL with increasingly more complex and challenging analysis tasks. Neural networks function like the human brain, and intense analytics activities require a brain-simulator environment to resolve highly complex business problems.
So, the broad field of AI, with all of its sub-fields, enables Data Science. Data is an essential component in Data Science, machine learning, and artificial intelligence (AI). However, here are some major differences between the three fields:
Data Science
- Data Science involves using statistical and computational techniques to analyze large amounts of data, identify patterns, and make predictions.
- In Data Science careers, individuals need to have a strong foundation in statistics and mathematics, as well as programming languages such as Python or R.
- Some examples of real-world applications for Data Science include predicting customer behavior, analyzing financial data, and optimizing supply chain management.
Artificial Intelligence
- Artificial intelligence enables machines to mimic human intelligence and reasoning.
- Artificial intelligence also enables machines to perform tasks that require human-like reasoning, perception, and decision-making abilities.
- AI systems use deep learning, natural language processing, robotics, and computer vision to mimic human intelligence.
- For AI careers, individuals need to have a strong background in computer science and engineering. They must also be familiar with machine learning techniques and algorithms. Additionally, they must possess excellent problem-solving skills and the ability to think creatively.
- Some examples of real-world applications for artificial intelligence include virtual assistants like Siri or Alexa, chatbots used for customer service, and predictive maintenance in industrial settings.
Machine Learning
- Machine learning, a subset of AI, focuses on training machines to learn from data without being explicitly programmed.
- Machine learning relies on algorithms to make predictions based on patterns in data.
- In machine learning careers, individuals should have a deep understanding of algorithms and statistical models. They must also possess strong programming skills and be able to work with large datasets. Additionally, they must have a solid foundation in linear algebra and calculus.
- Some examples of real-world applications for machine learning include image recognition in self-driving cars, fraud detection in financial transactions, and personalized recommendations on streaming services.
The core difference between Data Science vs. machine learning vs. AI is that while AI and ML provide answers to business problems, the data scientist finally comes to build a convincing story through visualization and reporting tools to consume a broader business audience. The business audience may not understand what a random forest is, but once the data-driven story is in front of them, they immediately understand the relationships and patterns among different business components, along with their future impact on business. The data scientist is, undoubtedly, more of the domain expert than an AI or ML practitioner to be able to build the final story from data-driven insights.
The differences between AI and ML are best understood through their applicable use cases. AI and ML work together to automate human activities like customer service (digital assistants), driving vehicles (self-driving cars), and offering personalized recommendations (recommendation engines). One benefit of using AI and ML is often understated: the benefit of making huge cost savings by eliminating human workers from these functions.
Data Science, AI, and ML in 2023: Benefits and Limitations
Today, Data Science, AI, and ML are all mature technologies that are transforming the way we use data. Each technology has its own benefits and limitations.
Data Science is a multidisciplinary field that combines statistical analysis, computer programming, and domain expertise to extract insights from data. Its benefits include identifying trends, patterns, and correlations in large datasets, which can help organizations make better decisions. However, its limitations include the need for highly skilled professionals to analyze data.
AI is an umbrella term for systems that can perform tasks that typically require human intelligence such as visual perception or natural language processing. Its benefits include increased efficiency and accuracy in decision-making processes.
Machine learning uses algorithms to learn patterns from data without being explicitly programmed. Its benefits include automation of tasks such as fraud detection and personalized recommendations. However, its limitations include the need for large amounts of high-quality data to train models effectively. One major concern is the potential for bias in the data used to train these algorithms, which can perpetuate and even amplify existing societal inequalities. This can have serious consequences in areas such as hiring practices or criminal justice decision-making. Another ethical consideration is privacy. As more and more personal data is collected and analyzed, there is a risk that individuals’ privacy may be compromised.
Conclusion
In business, Data Science, machine learning, and AI are used for various purposes such as personalized marketing, fraud detection, customer service automation, supply chain optimization, and predictive maintenance. By utilizing these tools, businesses can gain valuable insights into their operations and customers that they may have otherwise missed.
The future of Data Science, machine learning, and AI is bright and promising. One of the trends to watch out for is the rise of edge computing. With the increasing amount of data generated by devices such as smartphones and IoT sensors, edge computing allows for real-time processing and analysis of data at the source. Another trend is the integration of AI in various industries, from healthcare to finance.
Image used under license from Shutterstock.com