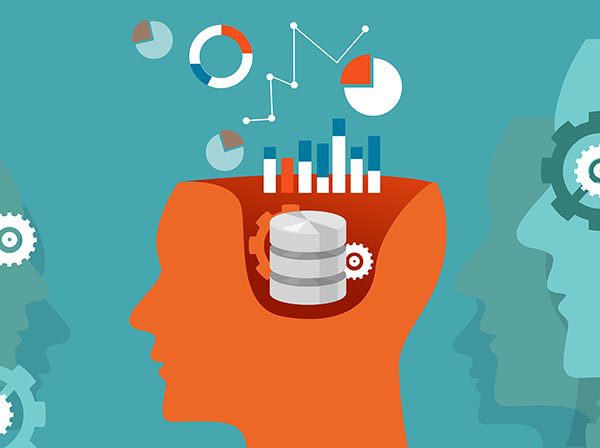
The Background of Data Science Roles
It was thought a few years ago that 2018 would amount a huge demand-supply gap in the Data Science market as supply would fail to keep pace with the rising demand for expert data scientists. However, the buzz from Gartner, which said more than 40 percent of Data Science tasks would be automated by 2020, changed all that. Boris Gorelik, an experienced data scientist, expressed the same opinion about the future of this field and cautioned against selecting Data Science as a career. But it seems that has not come to pass, the field is still growing.
The general belief in the industry was that as more and more advanced automation tools are developed, the need for pure data scientists would erode. In the future, domain experts and data researchers will be more in demand than data scientists. Another buzz in the market suggests that as Data Science moves more toward automation, the back-end, human tasks of data analytics are now coming to the foreground in the form of a data engineer. But data scientist jobs are still increasing as the need for machine learning, AI, and other advanced technologies are surging forward.
So Who Is the New Tech Whiz on the Block?
The data engineer will likely take charge in near future, guiding the users through the foundation stages of data exploration and analysis. This new data nerd will not only clean and prepare data, but compile database systems, develop appropriate queries, work across platforms, and take care of disaster recovery — all tasks rolled into a single role. The data engineer is also expected to have solid big data skills, along with hands-on experience with several programming languages like Python, Scala, and Java.
In sharp contrast to the data engineer role, the data scientist is headed toward automation — making use of advanced tools to combat daily business challenges. The future data scientist will be a more tool-friendly data analyst, utilizing a combination of proprietary and packaged models and advanced tools to extract insights from troves of business data.
With the increasing integration of AI and machine learning in data analytics platforms, the data scientist of tomorrow may no longer need to have degrees in quantitative fields or to develop algorithms from scratch. The data scientist will still play the role of an advisor or recommender, but with different skills.
If you are itching to dig more into these complimentary roles, then review the DATAVERSITY® webinar Data Governance and Data Science to Improve Data Quality. This webinar is designed for enterprises looking to build an insights-driven business model by harnessing the power of Data Science and Data Quality, along with Data Governance. The objective of this webinar is to teach profiteering from business data.
The Data Science and the Data Engineering Roles: In Sharp Contrast
A Dataquest blog explains that the data engineer usually lays the groundwork for the data scientist to “analyze and visualize data.” Some of the initial tasks performed by the data engineer may include managing data sources, managing databases, and launching tools to make the data scientist’s job easy. So, strictly speaking, the data engineer handles all the back-end tasks of data analytics that lay hidden from the public eye.
Data Engineers Will Be More Important Than Data Scientists suggests that data chiefs in modern enterprises are realizing that advanced and automated tools alone cannot deliver results, which are expected to be both superfast and at scale. In these businesses, the data engineer will aid the machines to deliver fast solutions at scale. It is more than likely that in such a scenario, businesses may think of providing insights as a service. The expense-conscious business operators are now questioning whether it is prudent to invest in data scientists when data engineers and advanced tools can deliver better results faster.
Today’s insights-driven businesses are more comfortable with the idea of vesting the responsibility of a “Data Architecture” — the blueprint for Data Management and Data Governance — to the data engineer, who is believed to be a seasoned expert across all these areas. Data Engineers are in Greater Demand than Data Scientists gives an inside view of failed big data projects due to the lack of data engineers in data teams. The article argues that every data team requires at least five data engineers for every data scientist.
Most of the available industry literature point out that data engineers are not only more valuable than data scientists, they are almost indispensable for the success of big data projects. According to Michelle Goetz of Forrester research, “There may be twelve times as many unfilled data engineering jobs as Data Science jobs.”
What Is the Special Contribution of a Data Engineer?
Simply put, the data scientist can interpret data only after receiving it in an appropriate format. The data engineer’s job is to get the data to the data scientist. Thus, as of now, data engineers are more in demand than data scientists because tools cannot perform the tasks of a data engineer.
Data Scientist vs. Data Engineer: Some Published Data
A Data camp post reconfirms that much of the Data Science tasks today will remain unfinished unless the data engineer develops processes for modeling, mining, and gathering the data. Though traditionally there was an overlap in the Data Science and data engineer roles, the differences are clearly apparent now.
The data engineer has moved far away from the data scientist of yesterday, and in today’s context, the data engineer is more involved in managing databases and setting up Data Modeling environments. The data scientist comes at the end to use knowledge of quantitative science to build the predictive models. In recent years, the data engineer has moved away from the shadow of the data scientist and come to the foreground, gaining more prominence.
To promote an understanding that Data Engineering is almost a part of enterprise Data Governance mission, the webinar Data Architect vs. Data Engineer vs. Data Modeler demonstrates the correlation between Data Quality engineering and an overall business strategy. The presenter of this webinar, Donna Burbank, is a firm believer that enterprises can identify business problems very quickly if they explore the data problems.
According to O’Reilly, the data engineer has superior programming knowledge while the data scientist has more advanced knowledge of data analytics. Then there is the machine learning engineer, who sits at the intersection of Data Science and Data Engineering. The implicit message in this publication is that while the data engineer takes care of the more nitty-gritty details of data preparation, the data scientist can now concentrate on certain other (more sublime) tasks.
Conclusion
Both data scientists and data engineers are here to stay, but data scientists may gradually fade into the background while the data engineer will gain more prominence in the foreground, handling all the manual processes of data analytics.
Image used under license from Shutterstock.com