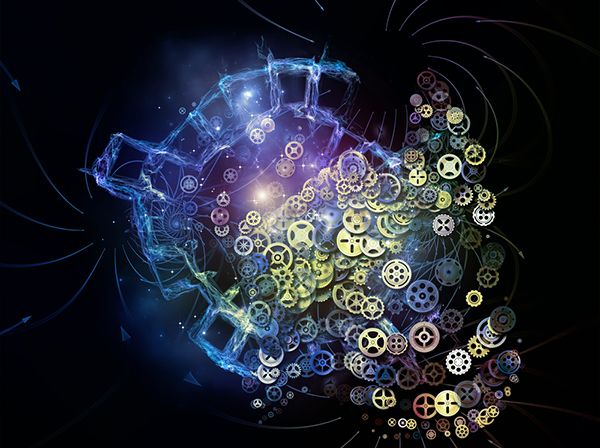
As the world becomes increasingly data-driven, businesses are turning to self-service analytics to enable business users to perform their own data analysis tasks. In self-service analytics, business users can access and analyze data without assistance or support from IT personnel or data scientists. Direct access to ML-powered analytics platforms allows them to make better business decisions by analyzing customer behavior or by identifying trends in real time.
In the past five years, fully automated and semi-automated software systems have delivered more reliable analytics and business intelligence (BI) reports than human data scientists. As artificial intelligence-powered BI technology heads towards full self-service, a general concern in the Data Science community is whether in an ever-growing self-service analytics world, human data scientists will become obsolete due to the presence of super-intelligent analytics and BI tools.
Is Self-Service Analytics and Business Intelligence a Myth?
Currently, many analytics and business intelligence tasks are handled by semi-automated or fully automated analytics platforms, especially ones powered by AI and machine learning (ML) tools. It’s interesting to note that human data scientists dominated the area of data mining until recently advanced ML-enabled tools took over many tasks. Data mining techniques closely guarded by human experts for years have now suddenly been replaced by advanced ML tools. These tools can detect patterns in data, establish correlation, and extract the required insights as needed by ordinary business users.
Self-service BI is no myth, as current businesses of all sizes are routinely using packaged machine learning algorithms for profitable decision-making. The algorithm economy is here to stay. There are two obvious advantages of using packed algorithms for business analytics: the cost and the instant availability.
Two marked trends that long ago defined the self-service business intelligence world are still visible: the deep fascination with click-button analytics rather than coding analytics function, and the preoccupation with virtual data repositories.
The Role of Data Scientists in the Self-Service Analytics World
While “data culture” is quickly spreading, data scientists are still making value additions to the business by leveraging technology to deliver quicker and more accurate solutions to all types of users.
The self-service BI revolution brings data scientists to the business corridor, where they discuss complex analytics issues with other employees. The tremendous growth of citizen data scientists and machine learning tools have resulted in the rise of self-service analytics and self-service BI. This DATAVERSITY® article describes a real-life journey into the business practice of self-service BI today. It points out that automated cloud-based tools have snatched the roles of business analytics and data scientists and put them in the hands of citizen data scientists. However, only a data scientist is qualified to bridge the gap between “raw intelligence” extracted from smart platforms and decision-friendly insights flashed through dashboards. The average business user may accomplish somewhat more than just filtering and grouping data in the self-service world, but cannot achieve advanced visualization tasks.
Data preparation and extraction still remain the biggest challenges in automated BI platforms, and the complex interrelationships between many related technologies like Hadoop, big data, and data discovery pose a threat to technology access, use, and comprehension in the self-service world. “Assisted BI” may be a better term to describe the future of self-service business intelligence. Moreover, data security and Data Governance turned out to be challenging issues in the self-service BI world, for which enterprises have had to choose between more advanced BI platforms or expensive and well-trained data professionals.
The Rise of Citizen Data Scientists in Self-Service Analytics
Today, ordinary business users need self-service platforms to get their jobs done quickly and easily. The topmost reason for this transformational business shift toward self-service BI was the impending talent gap in the Data Science profession forecasted by McKinsey many years ago.
Very quickly, businesses began to explore solutions to this manpower gap, one of which was procuring, building, and deploying self-service analytics and BI platforms to fill their in-house needs. Of course, merging technologies like cloud, IoT, and big data also strengthened the “viability” of self-service platforms in the long run. In this newly developed, self-driven analytics world, the citizen data scientist is seen as a partner and collaborator for the trained data scientist.
The Data Scientist as a Collaborator on a Self-Thinking BI Platform
Currently, business intelligence solutions cater to two widely disparate consumer segments: ordinary business users and professional IT teams. While business users are excited about becoming self-sufficient in routine analytics or BI tasks, IT team members are also enthusiastic about faster extraction of deep insights with the use of automated or semi-automated BI tools.
An AnalyticsInsights.net article explores whether human data scientists will vanish from the enterprise with the sudden rise of the citizen data scientist. There’s a strong hint in this article that a day will finally come when the average business user, along with super-powerful ML platforms, may eventually replace the Data Science community altogether.
According to the World Economic Forum, though the recent technological disruptions are threatening white-collar jobs across the world, data analysts will be in demand in the long run to aid self-service business intelligence platforms.
Self-Service BI or Assisted BI: Which Is More Achievable?
Businesses need to find users who understand both technology and business processes to ensure their success in the world of analysis. In the world of intelligent analytics, businesses are constantly on the hunt for tools and solutions that will help them make sense of the massive amounts of data they generate. However, mismanaged analysis processes can lead to inaccurate insights and poor decision-making.
This is where needed data scientists come in – they possess the skills necessary to extract meaningful insights from raw data and interpret complex data correlations that may not be obvious to the average user. While artificial intelligence and other technologies have made significant strides in recent years, there is still a need for human data scientists who can bring a unique perspective to the table.
The Data Science community plays an important role in advancing our understanding of data and creating new tools for analysis and discovery in this ever-evolving BI world.The algorithm economy is pushing business communities toward “insights” from plain information. However, the core activity that delivers business insights is analytics, and without advanced analytics or BI tools, businesses will head for failure in the future world of global competition. This is where embedded analytics come into play. In an embedded analytics project, analytics knowledge and skilled manpower are required from beginning to end. Assisted analytics will be needed along with self-service in the increasingly competitive business world.
Self-service analytics platforms are perceived as a “double-edged sword.” While the ease and power of self-service BI is undeniable, the long-range maintainability of these platforms in terms of data security, Data Governance, and data spillage poses a big challenge. The implication is that highly skilled IT teams will be required to maintain these systems.
Risks and Benefits of Self-Service BI
The biggest benefit of the self-service analytics and BI platform is that it empowers ordinary business users to become citizen data scientists. While performing their daily functions within strict time constraints, the business users certainly find the self-service platforms handy and accessible for getting their jobs done without much fuss.
The biggest disadvantage or “risk” of a self-service platform is that users may fail to derive insights from available data, misinterpret the results, or misapply the insights. While the human data expert knows how to talk to the machine in case of problems, the average business user does not have such skills. In many situations the citizen data scientist is still compelled to turn to the real data scientists for help and support.
The data explosion, increasing data types, emerging technologies, and the cloud have compounded the challenges of self-service analytics, despite data preparation and data access tools. Moreover, there are issues that need to be dealt with involving data security and Data Governance in self-service analytics platforms. All said, a strong case can be made for a “distributed BI framework” with full attention to security and governance issues.
Conclusion
In the self-service analytics world, data scientists are still needed to improve business intelligence and help companies make better business decisions. While self-service analytics platforms allow users to access and analyze data on their own, it is limited by the user’s knowledge of analytical methodologies. Data scientists can enhance BI activities by using predictive analytics and ML power tools to generate predictive insights.
In the self-service analytics world, business people are now taking more responsibility for their own data needs. However, they still need teams of data experts to come up with solutions. Data scientists are still important in this world, as users need them to have information at their fingertips when they ask questions.
While self-service analytics tools can help business users perform basic analytics tasks, data scientists are needed to help these same users perform more complex tasks and conduct deep-dive analytics.
Image used under license from Shutterstock.com