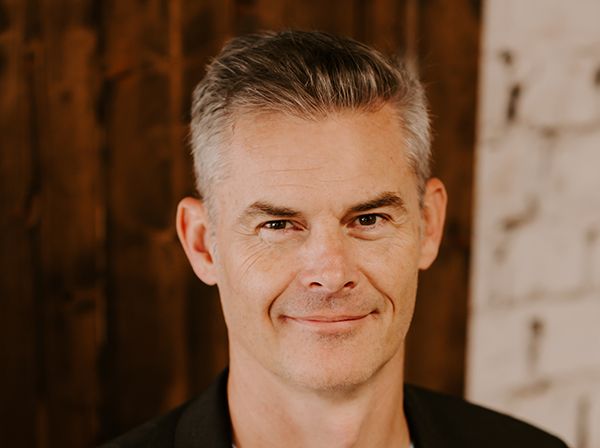
According to Gartner, 80% of executives believe organizations can apply AI to any business decision. However, an AI-based decision is only as effective as the training data on which the AI model is built. The most effective, advanced solutions focus on domain-specific AI and ensure training data used in AI models is highly accurate and reliable. Humans perform or supervise a labeling and tagging process to generate the training data. The more accurately a qualified human labeler tags and creates training data, the more effective and reliable the model.
Particularly important to conversational AI is the source of its training data and the data source’s relevance to the desired use case. AI models trained on non-industry-specific or publicly available data sources – like AI for all and open source – won’t (and can’t) consider the intent or meaning behind words used in different industries.
Many industries rely on AI models trained in the manner described above. Too many nuances become lost, resulting in:
- Inaccurate, off-base interpretations of conversations’ meaning.
- A lack of trust in the models to accurately predict and present reality.
- User disengagement – and mistrust in AI’s ability to solve real issues.
By training domain-specific AI models to understand industry-specific jargon and words’ situational contexts – and then build on that knowledge – companies gain increased clarity, more reliable data, and deeper insights.
Organizations that want to improve their customer service must bring structure, visibility, and meaning to their high volumes of conversational data. The best way to achieve that goal is through domain specificity. This is particularly important in the healthcare industry.
The Value of Domain-Specific AI
To work most effectively, the healthcare industry needs people to review and train data models, ensuring accuracy and reliability. AI is emerging as a critical tool to help support more automation and elevate human decision-making with enhanced data and context.
Success looks different depending on the industry – and that’s why a one-size-fits-all approach doesn’t work. Whether working in finance, technology or healthcare, leaders need AI that understands their specific sector.
AI has a much higher capacity than humans to collect and analyze vast quantities of data. True domain-specific AI has been infused with expert-level knowledge and can work at higher speeds and scale to distribute highly specialized insights and information for informing business decisions. Within the healthcare industry, finding and focusing on highly sensitive, highly complex healthcare conversations and extracting meaning for leadership in a highly contextualized, relevant way becomes easier. Use cases for this approach include:
- Identifying non-compliant conversations (e.g., HIPAA and adverse events)
- Understanding the connection between customer sentiment and diverse topics
- Highlighting customer experience disruptions like the Eddy Effect (when customers get stuck in a loop of frustrating obstacles) and other pain points along the customer journey
- Determining agent performance, quality, and training needs
Healthcare Benefits from AI Trained on Industry-Specific Data
When exclusively trained using healthcare-related discussions, AI models derive significant insights from healthcare conversations. Healthcare organizations leveraging a conversational intelligence solution specifically built for their industry benefit from AI that:
- Brings speed and value to leadership and key stakeholders across the organization
- Deeply understands healthcare’s regulatory nature
- Successfully extracts the most value from conversations between customers and agents
Healthcare organizations using domain-specific AI gain a competitive advantage in identifying customer pain points, drivers of long calls, and call influxes; finding opportunities to upgrade and expand agent training; and improving customers’ accessibility to mental health and other resources. Training AI models with healthcare-specific terminology enables them to sift through the noise to surface themes, patterns, and urgent customer concerns.