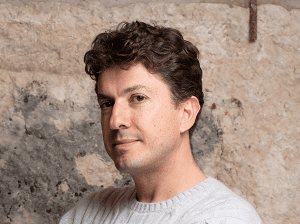
Commerce today runs on data – guiding product development, improving operational efficiency, and personalizing the customer experience. However, many organizations fall into the trap of thinking that more data means more sales, when these two factors aren’t directly correlated. Often, executives will become overzealous in their digital transformations and cut blank checks for data collection, without realizing that data is a means to an end, not the end itself. Too much data isn’t just a waste of capital and labor, it risks obscuring and altering the actual truth, steering organizations off course when it comes to their sales and marketing campaigns.
So how can organizations ensure that they’re valuing quality over quantity in their data collection processes? The answer lies in data granularity. When the right level of data granularity is dialed in, businesses can separate signal from noise – and execute more effective merchandising and inventory plans.
Data granularity refers to the level of detail within data. Granularity measures the density of information contained in a single point. Today, markets rapidly fluctuate to satisfy ever-changing patterns of consumer demand, desire and behavior, making access to highly granular data even more crucial. For many organizations, data granularity can be the difference between having the right stock in the right location versus losing customers to out-of-stocks and competing retailers.
Breaking from the Linear Model
To illustrate the importance of data granularity, let’s examine the price of luxury goods in the U.S. Traditional thinking dictates that East Coast residents are less price sensitive, because their incomes are, on average, higher. Many will make this incorrect assumption because they’re familiar with the luxury industry – where discounts are often ineffective and sometimes even detrimental to sales – and therefore conclude that a customer with more income will be less swayed by incremental changes in price. However, this logic is disproven when comparing Manhattan and say, Kansas City. In NYC, there’s a proliferation of luxury retailers; therefore, the competition between them will be fierce, leading to higher price sensitivity in New York than in Kansas City, where there are fewer luxury stores.
Because the correlation between price and the chance of purchasing is not linear, a discontinuous approach to data analysis is needed. In this case, a standard trend line cannot be drawn, because there are too many outliers and rapid volatility among plot points. The optimal price can only be calculated if we zoom in to the block-by-block level. Many mistakenly value quantity over quality of data by gathering incredibly detailed data on a city-wide level, without realizing that this blunt approach isn’t applicable to such a hyper-localized market.
Granular data acknowledges that the average customer is multifaceted – they’re motivated by their financials, their location, their friends, and their personal goals. There are countless non-price-related factors to consider. For example, the psychology behind why customers have an aversion to buying goods priced at $20 compared to $19.99 is complex. Granular data doesn’t seek to answer why this is, it simply recognizes that the pattern occurs and alters prices accordingly. If retailers relied only on linear pricing models, they would be completely blind to this phenomenon.
The Post-Pandemic Landscape
A silver lining of COVID and the ensuing supply chain crisis was that it forced organizations to reevaluate their linear data models for the first time. Brick-and-mortar retail plagued with overstocks tried drastic discounting measures, yet inventory wouldn’t budge. This is because the normally price-sensitive customer stopped buying due to external factors. The inverse happened in 2023 – it was a bumper year for many companies, but discounts were hardly responsible.
Volatile macroeconomic conditions shed light on the value of agility, another bonus of considering data granularity to the needed levels for pattern recognition – something only prescriptive data models can achieve. Linear data models cannot account for global health crises, geopolitical tension, or ships getting stuck in the Suez Canal. Predictive data models try to but are constantly playing catch-up. Prescriptive data models tuned to the right granularity help organizations react fast enough that they never need to predict the future.
Powered by AI
Advancements in artificial intelligence and the push for accurate data granularity go hand in hand. The technology is getting there. Soon AI will help you get to the level of granularity equivalent to having a million analysts working exclusively on your problem. AI can help organizations avoid a common pitfall during digital transformations: adjusting to the wrong KPIs. The rough transition from traditional processes to data-backed operations can be smoothed with AI tools – ensuring that organizations do not over-fit or under-fit analytic data models.
As AI progresses, we will shift from a culture of learning to answer questions to learning how to correctly ask them. Rote tasks will be automated away, and real value will stem from framing a problem rather than manually solving it. Using the same logic, data itself will become more accessible and less coveted – while a premium will be placed on granularity.