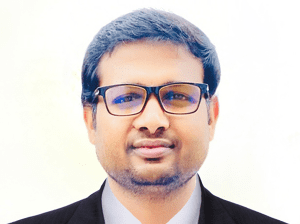
In the digital age, organizations increasingly rely on data for strategic decision-making, making the management of this data more critical than ever. This reliance has spurred a significant shift across industries, driven by advancements in artificial intelligence (AI) and machine learning (ML), which thrive on comprehensive, high-quality data. This evolution underscores the importance of master data management (MDM) to ensure data quality and consistency across various domains and sources.
Defining a Robust Data Governance Framework
MDM is a strategic, methodical approach to managing an organization’s critical data. It involves the creation and management of a single, consistent, and accurate view of essential business data, often called master data. Master data covers core business entities such as customers, products, employees, and suppliers and is used across various business processes and systems. The primary goal of MDM is to provide a unified, accurate, and consistent view of this data across the organization, thereby enhancing operational efficiency, improving decision-making, and enabling better customer experiences.
The process of ensuring data quality and consistency within MDM is multifaceted. It encompasses the implementation of data quality checks, the leveraging of data matching and merging techniques, and the adoption of a comprehensive data governance framework. For master data management to succeed, it is imperative that companies create a data governance framework that will assist them in setting standards and regulations for data, determining who owns and is responsible for what data, monitoring data performance, and troubleshooting. All MDM sources and domains are defined inside a data governance architecture, outlining their interactions and integrations. Stakeholders are provided with accessible and comprehensible documentation of data definitions, metadata, lineage, and quality metrics.
This framework sets the foundation for effective data management by establishing clear policies, roles, responsibilities, and processes for collecting, storing, managing, and utilizing data. It aligns MDM strategies with business objectives, sets data standards and rules, assigns data ownership and stewardship, and implements mechanisms for monitoring data quality and performance.
Establishing the Correct MDM Architecture
Selecting the right MDM architecture is crucial for tailoring the approach to the organization’s specific needs. There are three common options for MDM architecture: registry, repository, and hybrid. Each has its strengths and is chosen based on factors such as data volume, complexity, organizational capabilities, and overall business strategy.
The registry MDM architecture creates an index or a pointer system to master data that resides in disparate systems across the organization. It doesn’t store the master data but provides a consolidated view by linking to the original data sources. This read-only approach minimizes disruption to existing systems. It is beneficial for organizations seeking a quick win in mastering data without the need for extensive data migration or consolidation efforts.
The repository (or centralized) MDM architecture creates a single, authoritative source of master data maintained in a central repository. All systems and applications across the organization consume master data from this central source. This model supports the highest level of data quality and consistency but requires significant effort in data consolidation and governance to maintain the central repository.
Hybrid MDM architecture combines elements of both the registry and repository models, offering a balanced approach that accommodates a wide range of use cases. It allows organizations to manage some master data centrally in a repository while linking to other data sets that remain in their source systems. This flexibility makes it ideal for organizations with complex data landscapes, including high-quality, centralized data needs and decentralized, department-specific requirements. For example, a global retail chain might choose the hybrid model. This allows it to centralize its product information to ensure consistency across all locations while allowing for local variations in pricing, promotions, and inventory levels.
This architecture melds the centralized repository approach, where core product data such as names, categories, and standard prices are uniformly managed, with the registry model’s ability to link to decentralized, region-specific data. As a result, each store accesses up-to-date, accurate master data that aligns with corporate standards and local market requirements. Ultimately, the efficacy of an MDM architecture does not solely rest on the model chosen but on how well it is tailored to meet the unique blend of the organization’s global integration and local flexibility needs, ensuring that the chosen architecture serves as a dynamic enabler of business strategies rather than a constraint.
The Lifecycle of Data
Data quality and consistency are crucial in MDM to ensure data’s accuracy and integrity throughout its lifecycle, from acquisition and cleansing to integration and maintenance. This process tackles the challenge of harmonizing extensive and complex data sets from varied sources and systems, using tools and sampling techniques to streamline quality assessments and maintain reliability.
Documentation of data definitions, metadata, lineage, and quality metrics is key to clarity and stakeholder comprehension, aiding in the establishment of data quality standards and the application of a structured quality framework. Automated tools enhance this framework, which supports precise documentation and data validation and foster better organizational collaboration and decision-making.
Leverage Data Matching and Merging Techniques
Utilizing data matching and merging techniques allow for the consolidation and harmonization of data from various sources and domains into a unified master record. Identifying and connecting records that pertain to the same entity or concept involves data matching using criteria like name, address, email, phone number, or identifier. Combining matched records into a single record consists of following rules like survivorship, precedence, or consensus. Data matching and merging techniques are used to remove data redundancy, inconsistency, and ambiguity and to establish a unified view of the data. Effective data quality communication and reporting is critical to elevate data quality, compliance, and operational efficiency.
Implementing MDM with a Change Management Approach
Change management is of the utmost importance to minimize disruption and optimize the adoption of MDM. Change management is the process of coordinating the effects of modifications to a data environment, including the addition of new sources, domains, regulations, or requirements. In addition to planning, communicating, implementing, testing, and monitoring the changes, change management provides training, support, and engagement for all data stakeholders. This ensures that all team members are informed of and agree with the modifications and that data quality and consistency are preserved or enhanced throughout and following the changes.
Learning from the experiences of others, such as retail companies integrating customer data or technology firms merging MDM systems, can offer valuable insights. These examples highlight the importance of a structured change management process in maintaining data quality and ensuring the success of MDM initiatives. Ultimately, the effectiveness of MDM depends on technology and an organization’s ability to adapt to change, keeping data as a strategic asset.
Looking Ahead
Organizations are managing their data and transforming it into a pivotal strategic asset by establishing comprehensive data governance, the strategic selection of MDM architectures, and the rigorous implementation of data quality checks. The integration of emerging technologies like AI and ML promises to revolutionize MDM, offering even more nuanced insights and fostering a more agile data management environment. As businesses evolve in this data-centric world, the mastery of MDM becomes a key differentiator, empowering organizations to navigate the complexities of the digital age with confidence and foresight. This evolution in data management is not just about maintaining efficiency; it’s about pioneering a future where data’s potential is fully realized, driving innovation and success in an increasingly data-driven global economy.