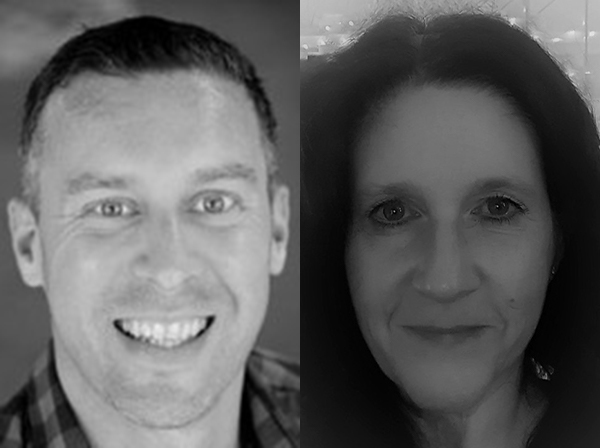
Regardless of how clever the machine or how brilliant the algorithm, the success of intelligence-based solutions is intrinsically tied to the quality of the data that goes in. That’s why, aside from its people, data is the most important thing an organization owns. Data must be the first stop on the journey to implementing artificial intelligence (AI) or machine learning (ML) in any business setting, with retail being no exception.
Why Is Data Important in Retail?
With the rise in popularity of AI and ML applications in virtually all business settings, the importance of good data is undeniable. The success of cognitive applications relies heavily on the data that’s put in; clean, quality data is the end goal, but getting there isn’t necessarily a one-size-fits-all process.
Sewing together all the bits of information retailers capture about customers – their journeys and search history, their transaction and order history, their delivery preferences, their responses to marketing – is how retailers can stand a fighting chance at predicting trends. These predictions will inform businesses on where to place stock in order to maximize sales and profit and how to evolve products and services to continue to engage customers.
The importance of data doesn’t stop at capacity or demand planning, however. In a global economy, data’s role in compliance is critical. For retail brands, that means data is accurate and factual in order to support an efficient critical path and supply chain – most notably following the U.K.’s exit from the EU and the increased focus on data that enables the efficient movement and taxation of goods across borders as well as region-specific compliance to standards. As our world becomes more data-reliant, businesses will have to capture more information to become more accurate, lean, and competitive.
The Challenge of Unifying Data
With the proliferation of “big data” come challenges of standardizing that data. For most organizations, pulling data from several different systems that all speak different languages and then formatting that information in a meaningful way can become tedious. In some respect, the ability to get systems to talk to each other is quite often the easiest part. The most difficult piece is figuring out the best way to ensure consistency, quality, and enduring data governance.
When we approach data consistency in retail, it’s wise to look at other sectors for insight into what standardization can look like. In telecommunications and energy sectors, there have to be standard processes in place for exchanging data due to legal mandates (i.e., breaking up monopolies) and ethical requirements (i.e., fair exchange of data). While retail doesn’t quite fit into that mold perfectly, we can glean some elements from highly standardized industries and apply them to our own space.
Each retailer’s approach to introducing a standard data model will look a bit different than the next, but the important element is that there is a standard format that can be applied. For instance, tried-and-true Electronic Data Interchange (EDI) formats are used frequently in retail. While it is considered old-fashioned, EDI is a proven method for retailers to communicate with one another and keep goods moving. Depending on the maturity of an organization, the standardization model will vary.
Walk Before You Run
The process of cleaning, organizing, and standardizing data is nothing short of overwhelming; it’s quite complex and there is a lot to learn even for big-box retailers that have seemingly endless resources to allocate. But that should not deter organizations from pursuing a future in AI and ML technology. Chiseling away at data can be a slow and steady process, and as companies get more comfortable and confident in how the process works, they can begin to move faster, make decisions more intuitively, and even get creative in the way they process and utilize their data. In simple terms, AI can be considered an added team member that takes the mundane tasks off the hands of humans in order to make room for more complex decisions. Here are a few jumping-off points for businesses starting out:
- Demand Forecasting: Collecting and analyzing data on purchases or completed transactions is a simple introduction of intelligence-based tools. This information can be used to determine where demand is coming from and indicate trends. This can also include search queries generated on a website to glean information on what shoppers are looking for so that fulfillment plans can be created.
- Capacity or Network Planning: Making sure goods are in the right location is always a good place to start when it comes to things like distribution and supply chain. Collecting this data ensures that stores or vendors are optimizing their execution and that those vendors or associates have what they need to properly complete certain tasks.
- Customer Data: Tracking online and offline interactions can help retailers piece together customer personas. Each customer has a digital footprint, and that information can inform businesses on important things like what shoppers value and their preferences. This data has endless value, helping businesses construct stories and inform product development and adapt to the needs of the customer.
Toiling the Data Soil
The saying “you get out of life what you put into it” cannot be more true in the context of high-tech tools that utilize data. The better the data fed to a system, the more accurate the information and insights a business will receive. The most important thing to know about AI, ML, or any cognitive-based tool is that they are a living, breathing entity that requires constant correcting, training, and tweaking in order to do the job right. These machines are far from perfect, but when the humans behind the curtain understand the criticality of the data put in, the possibilities are endless.