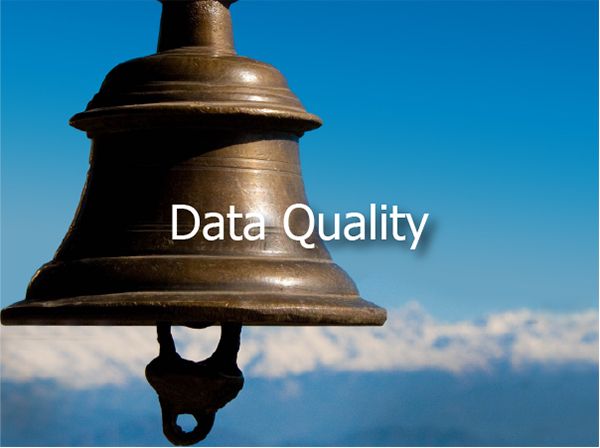
Data Quality (DQ) describes the degree of business and consumer confidence in data’s usefulness based on agreed-upon business requirements. These expectations evolve based on changing contexts in the marketplace.
As people get new information and experience different interactions, business requirements face updating, redefining Data Quality needs within the data’s lifespan. Since DQ represents a moving target, it requires ongoing discussions and consensus to get it to and remain at a trustworthy level.
While some people may have Data Quality expectations based on past experiences or implicit assumptions, these factors must be verbalized to avoid misinterpretation when necessary. Consequently, for Data Quality to be helpful, conversations need consensus on what level of DQ is feasible or good enough and how much deviation from the DQ threshold would be considered tolerable.
Once firms understand these measures, they can execute activities designed to maintain and improve DQ, such as effective Data Quality management, tool usage, and audits. Most importantly, companies must see DQ as an ongoing service necessary to stem increasing problems and incidents.
Data Quality Defined
Most Data Quality definitions cover a collection of techniques designed to meet the needs of those consuming that data. This methodology includes data planning, implementation, and control to make data fit for a purpose upon its use.
Moreover, common themes appear in DQ descriptions. According to Gartner, DQ meets parameters and comprises technologies for “identifying, understanding, and correcting flaws in data that support effective information governance across operational business processes and decision making.”
The Wang-Strong framework further expands the conception of DQ to meet additional data consumer requirements for trustworthiness. They type DQ attributes into intrinsic, contextual, representational, and accessibility characteristics.
While Wang-Strong provides valuable insights into data consumers’ expectations around DQ, these could be expanded to include those of data producers, administrators, and others who also have a stake in DQ. So, all possible DQ descriptions and dimensions can grow exponentially, potentially overwhelming the reader.
Data Quality Dimensions
A list of DQ dimensions or attributes should be recognizable, objective, easily understandable, and standard across most DQ content. To this end, DAMA-DMBoK2 and DATAVERSITY’s introduction on data quality dimensions have provided information about the following dimensions:
- Accuracy: Accuracy measures how well the available data corresponds with experiences in the real world. For example, DATAVERSITY is a company with headquarters in California. This fact is represented in the data shown on the website.
- Completeness: Completeness covers the extent that data and its metadata are present. For example, DATAVERSITY has a web page called “Contact Us” with a header “Corporate Headquarters,” containing its physical address and phone number.
- Consistency: Consistency describes how similar the original data and that delivered to another system, storage, interface, or through a pipeline match. For example, Tony Shaw’s email is consistent between the “Contact Us” and press release web pages.
- Integrity: Integrity measures how well any data set maintains its structure and relationships after data processes execute. For example, should DATAVERSITY experience a temporary outage, the web page returns when the issue is fixed as the same as prior and uncorrupted.
- Uniqueness/Deduplication: This dimension uncovers one or more versions of an entity described by the data. For example, all the information on the “Contact Us” page occurs only once and does not repeat on that or any other page on the DATAVERSITY website.
- Validity: Validity confirms that data behaves according to business expectations. For example, DATAVERSITY’s “Contact Us” page does not have webinar information or an article and only has information to get in touch.
Data Quality vs. Data Cleansing
While data cleansing overlaps with Data Quality, they do not mean the same. Data cleansing defines the automation of preparing a system’s data for analysis by removing inaccuracies or errors.
Data Quality has data cleansing and includes the practices and policies required to manage DQ, meeting good-enough data quality. These guidelines intersect with Data Governance – the different components needed to control data formally and guide DQ roles, processes, communications, and metrics.
Through Data Governance, organizations learn what data cleansing tools to purchase and how to use automation to get better DQ. Data Governance and other aspects of DQ planning steer companies on their data cleansing and how to assess its progress toward good-enough DQ. As business context and experiences change, this aspect of DQ has become even more critical than only data cleansing.
For example, a company executes data cleansing on several systems. It buys a new AI system for better and faster insights. Data Governance and DQ activities recognize that the organization needs to update its data cleansing process, among other tasks, to improve DQ for transport to the new AI system.
Why Is Data Quality Important?
Achieving an acceptable level of Data Quality remains critical for any business to stay profitable and thrive. Doing so means striking a balance between leaving DQ to chance and becoming paralyzed in pursuit of absolute confidence in data.
On the one hand, businesses and consumers need to trust the data they process and use. Doing DQ with less rigor costs money, time, and potentially lives. Alternatively, covering every possible avenue where DQ fails is not feasible. For example, companies cannot ensure 100% validity of each emergency phone call and text to every dispatcher in the U.S. from every LAN line and mobile phone version. If a validator checked every type of phone for a potential 911 misdial, there would be no time to respond to the emergency.
Good DQ assures businesses and consumers balance and confidence in critical data elements (CDEs), vital business information for successful operations and usage. For example, ensuring 90% of devices used over the last three years will show a 15% improvement in returning valid emergency calls achieves a balance.
Benefits of Good-Quality Data
Many articles connect DQ to reduced risk and cost, improved administrative efficiency and productivity, and a positive reputation. Additionally, DQ reduces costs and increases the chances for business growth.
Good Data Quality promises additional benefits. It makes businesses more agile, especially when confronted with dynamic changes, and provides a pathway for reconciling DQ issues and achieving DQ improvements.
These benefits become apparent upon DQ failures, which inevitably happen. Companies with good DQ can more easily identify the root causes and the steps to take and communicate both well.
Since it established business trust by implementing good enough DQ, businesspeople and customers will be more likely to back recommendations and activities around remediation. Consequently, a business with good Data Quality has more momentum toward growing its services or products.
Image used under license from Shutterstock.com