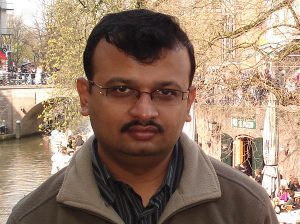
Are you drowning in data? Feeling shackled by rigid data warehouses that can’t keep pace with your ever-evolving business needs? You’re not alone. Traditional data storage strategies are crumbling under the weight of diverse data sources, leaving you with limited analytics and frustrated decisions. But what if there was a better way? A way to embrace the vast ocean of data at your fingertips and unlock its limitless potential? Enter the game-changer: hybrid architectures.
This revolutionary approach shatters the siloed world of data, marrying the flexibility of data lakes with the analytical power of the Data Vault model. Imagine effortlessly capturing all your data, structured and unstructured, from sensor readings to social media mentions. Picture a curated, business-oriented core that lets you slice and dice your data to uncover hidden insights and propel your decisions to the next level. Sound like a pipe dream? It’s not.
In this article, we’ll show you how hybrid architectures can transform your data strategy from a sinking ship to a high-seas cruiser, ready to navigate the turbulent waters of your business landscape.
Components of a Hybrid Architecture
The Data Lake
Imagine a vast, ever-expanding repository, the Grand Canyon of your data. This is the data lake, the landing zone for raw, unfiltered data from all corners of your enterprise: structured (databases, logs) and unstructured (social media, sensor readings). Think of it as the raw material fueling your analytical engine.
- Advantages: Scalability for massive data volumes, flexibility for diverse data types, cost-effectiveness for storing raw data.
- Challenges: Data governance to ensure quality and lineage, schema evolution to manage new data types, and query optimization for efficient exploration.
The Data Vault
Now, picture a meticulously crafted cathedral within the data lake, organized with a purpose. This is the Data Vault, the heart of your analytical power. It houses core business entities (customers, products, transactions) represented by “business keys,” independent of any specific source system. Data from the lake is cleansed, transformed, and enriched before entering the vault, becoming the building blocks for analysis.
- Advantages: Historical analysis through event-driven data capture, efficient querying through a normalized data structure, agility, and adaptability through modular design.
- Challenges: Maintaining data integrity during integration, balancing schema stability with evolving business needs, and ensuring data accessibility for diverse users.
The Links
Imagine intricate bridges connecting the cathedral to the surrounding landscape. These links connect data across the lake and vault, revealing relationships and context. They allow you to explore how customer orders connect to social media mentions or how sensor readings correlate with product performance.
- Advantages: Unlocking deeper insights through cross-domain analysis, enriching the vault with context from the lake, and enabling flexible exploration of data relationships.
- Challenges: Designing intuitive link structures for efficient querying, maintaining consistency between links and their corresponding data elements, and ensuring data security and access control across linked data sources.
The Tools and Techniques
Think of the architects, builders, and caretakers of this data ecosystem. Tools and techniques like ETL/ELT pipelines, data quality tools, data lake management platforms, and Data Vault modeling techniques are crucial in building, maintaining, and utilizing the hybrid architecture.
- Advantages: Automation for efficient data flow, governance for data quality and security, and best practices for optimizing performance and scalability.
- Challenges: Choosing the right tools for your specific needs, staying up to date with evolving technologies, training, and empowering data management teams.
Advantages of Hybrid Architectures
The promise of hybrid architectures in Data Vault 2.0 extends far beyond simply throwing data into a lake and building a neat house on top:
1. Flexibility to dance with the data: The data lake welcomes all data types, whether sensor readings, social media buzz, or traditional transaction logs, without forcing them into rigid schemas. This opens doors to unforeseen analyses, allowing you to discover hidden correlations and previously unimaginable insights.
2. Scalability: Hybrid architecture scales effortlessly. The data lake’s vastness accommodates data volumes that would make traditional systems choke, allowing you to capture every aspect of your business activity.
3. Cost-effectiveness: Budget constraints often pinch data initiatives. Hybrid architectures offer a breath of fresh air. Raw data resides in the cost-effective data lake, while the curated core of the Data Vault minimizes storage needs for frequently accessed analysis. This intelligent allocation of resources lets you maximize your data ROI.
4. Agility: Adapt and conquer in the data jungle: Hybrid architectures equip you with agility. New data sources can be easily integrated into the lake, requiring minimal changes to the Data Vault structure. This translates to quicker analysis, swifter decision-making, and the ability to outmaneuver your competitors.
5. Deeper insights: Traditional data warehouses often offer surface-level views. Hybrid architectures unlock hidden treasures. By connecting the dots between structured and unstructured data in the lake and the carefully curated Data Vault, you gain a 360-degree view of your business.
Challenges and Considerations in Hybrid Architectures
1. Data governance: Clear policies and procedures are crucial for managing data flow between the lake and the vault, preventing inconsistencies, and maintaining trust in your data assets. Think data dictionaries, audit trails, and access control mechanisms – all essential tools for keeping your data intact.
2. Schema evolution: While the core business entities should remain consistent, accommodating new data sources might require careful adjustments to the Data Vault schema. Striking the right balance between agility and data integrity requires thoughtful planning and collaboration between data architects and business stakeholders.
3. Query optimization: Efficiently querying across the data lake and the Data Vault can be tricky due to their different structures. Utilizing tools like MPP query engines and optimizing link structures becomes paramount for navigating the vast data landscape and retrieving the insights you seek.
4. Skills and training: Implementing a hybrid architecture requires expertise in Data Vault modeling, data lake management, and data integration tools. Invest in training your teams or recruit individuals with the necessary skills.
5. Tool selection: With many tools available, choosing the right ones can feel like navigating a minefield. ETL/ELT pipelines, data quality tools, data lake management platforms, and Data Vault modeling tools all play their part, but selecting the wrong ones can hinder your progress. Research, compare, and choose tools that seamlessly integrate and align with your needs and data landscape.
Conclusion
When implemented thoughtfully, hybrid architectures empower data-driven organizations to leverage the flexibility of data lakes alongside the analytical power of Data Vault 2.0. By carefully addressing the challenges and utilizing the right tools, organizations can unlock deeper insights and improved decision-making from their diverse data assets.