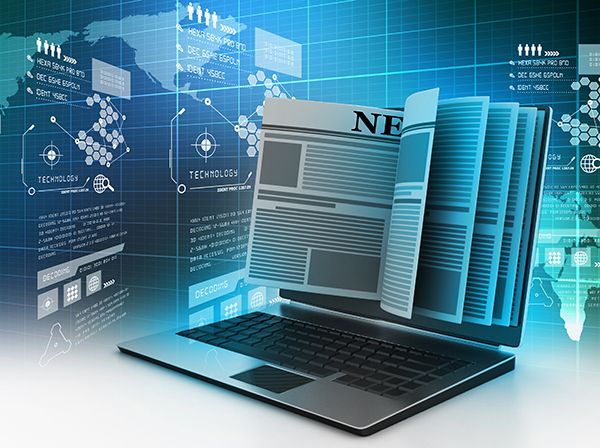
According to a new press release, Kinetica, a leader in real-time analytics, has unveiled a native large language model (LLM) integrated with its innovative architecture, enabling users to perform real-time, natural language-based data analysis without the need for external API calls. Unlike public LLMs, Kinetica’s solution prioritizes data privacy and security by keeping data within the customer’s environment. This announcement follows Kinetica’s previous integration with OpenAI, making it the first analytic database to do so.
With the increasing interest in LLMs, organizations and government agencies are seeking ways to automate business tasks while safeguarding sensitive information. Kinetica’s native LLM addresses data privacy and security concerns by staying within the customer’s network perimeter, offering enhanced protection. Moreover, this LLM is tailored to various industries’ syntax and data definitions, resulting in more reliable SQL generation and efficient handling of complex tasks for improved decision-making in fields like telecommunications, financial services, automotive, and logistics.
Kinetica’s CEO, Nima Negahban, emphasized the company’s commitment to expanding real-time, structured data analysis capabilities, powered by SQL-GPT. The U.S. Air Force has already leveraged Kinetica’s embedded LLM for advanced analytics on sensor data to enhance airspace security. The company also plans to integrate with other LLM platforms like NVIDIA NeMo in the future, providing clients with a choice of models. Kinetica’s database seamlessly converts natural language queries into SQL and delivers rapid responses, even for intricate questions, thanks to its native vectorization capabilities.
Kinetica’s native LLM is immediately available for customers, offering a secure, containerized environment for on-premises or cloud deployment. This development represents a significant step forward in harnessing real-time data for various industries and government agencies, ensuring both security and efficiency in data analysis.
Read more at kinetica.com.
Image used under license from Shutterstock.com