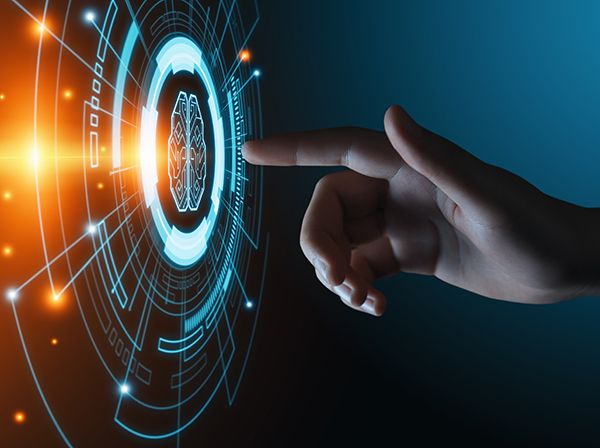
A subfield of artificial intelligence, machine learning (ML) uses algorithms to detect patterns in data and solve complex problems. Numerous fields and industries depend on machine learning daily to improve efficiency, accuracy, and decision-making. Recently, ML technologies have been reinforced by two subfields of their own: deep learning (DL) and computer vision. Read on for real-world examples of how machine learning and these subfields are being used in business and education today.
The Role of Deep Learning and Computer Vision
In 2023, deep learning has enabled computer vision to evolve further, providing machines with the ability to carry out rapid testing with several million test images. This has allowed the latest ML applications across sectors to use various vision-based applications such as driver distraction detection, object and computer vision techniques, 3D vision, and image segmentation. The combination of these techniques with deep learning algorithms and multiple deep learning methods has allowed for the development of real-time algorithms to assist in driving activities.
Additionally, image recognition technology is now being used to recognize different traffic scenes and objects, while feature extraction is employed for object tracking and image recognition. By combining computer vision with ML algorithms, industry applications can develop real-time algorithms that can help identify different traffic scenes and objects in them.
Machine Learning Examples by Industry
Here are more machine learning examples from the industry sectors:
Manufacturing: The manufacturing sector is reinventing its operational ecosystems with advanced ML applications impacting everything from supply chains and inventory controls to predictive maintenance. These seven real-life applications illustrate how ML technologies are revolutionizing the manufacturing business.
Medical: In the medical field, ML technologies have been used to diagnose neurological and musculoskeletal diseases. For example, trained ML algorithms can detect a wide range of diseases by identifying patterns in patient data. Additionally, ML can estimate the severity of diseases with great accuracy – enabling efficient patient-care delivery. Chatbots and image recognition help doctors with timely, life-saving services.
Computer vision algorithms are used to analyze radiology images such as MRI images. This technology can detect tumors and other anomalies in the body much faster than doctors can. It is also helping improve patient outcomes through early diagnosis.
In 2023, AI-enabled deep learning platforms will be widely used in the health care industry. These platforms enable deep learning to analyze the complete patient medical history, radiology images, and blood tests. They provide medical practitioners with actionable insights about a patient’s health. Deep learning algorithms can also use images and genomics to analyze unstructured data such as medical records. This will reduce the cost of testing and improve the accuracy of diagnosis.
Finance: In the financial sector, tailored ML algorithms can be used for fraud detection and other forms of security. Deep learning can also be used to analyze data via a process called “continuous multivariate analysis.” This process uses predictive analysis that is based on previous inputs and outcomes, allowing businesses to make decisions using more reliable data.
Cybersecurity: Cybersecurity professionals can use ML technologies to develop antivirus models, block possible cyber-attacks, and design email spam filters. Machine learning can also be used to detect potential frauds or cyber threats by cross-referencing customers’ activity with other IT security tools.
Sales and customer service: The use of ML algorithms and real-time, online data can help companies build ML models that make smart personalized recommendations. Amazon uses artificial intelligence, commerce brain, and artificial neural networks to create its own recommendation engine for customers. Other commerce companies such as Walmart also use neural networks to recommend relevant products based on customers’ recent order history and browsing history.
Industrial automation: Industrial robot-makers are now using intelligent systems with machine learning to reduce costs and increase efficiency. Industrial automation firms are also using artificial neural networks and deep reinforcement learning models to create better automation solutions.
Business analytics: Machine learning is revolutionizing businesses by providing powerful analytics solutions. With its ability to recognize patterns from large datasets quickly and accurately, ML is becoming an invaluable tool for companies of all sizes. Machine learning can help businesses make better decisions based on data-driven insights that can lead to long-term success.
Education: Machine learning examples from the real world can be applied to students’ knowledge and learning. Allowing students to have a hands-on approach within a workplace or room setting outside of the usual classroom setting reinforces their practical value and understanding of ideas within a professional context. These examples involve project-based learning and learner-centered teaching models. Challenging projects allow students to think outside the box and debate ideas on how best to apply existing knowledge to new problems.
Machine learning examples can be used to give students opportunities to interact with their peers, giving them a way to explore and learn, as well as reinforcing many skills that are necessary for success.
Research: Machine learning will continue to be a major player in industry research labs, with AI algorithm designers and research teams looking for the best solutions to implement. Explainable AI is an example of how machine learning is being used to better understand and explain machine learning models. DL models are used for detecting patterns in large datasets.
Intelligence systems such as the Semantic Web allow users to query information online with natural language processing (NLP) queries. This is a great way to expand research and bring researchers together to collaborate on various projects.
Advanced examples: Logic genetic algorithms are being used in various industrial applications such as in predicting customer behavior, data mining, analytics solutions, predictive internet, networks, fuzzy logic, and more. Electricity production plants are also using machine learning for predictive maintenance to reduce plant downtime.
Case Study: Machine Learning in the Classroom
Machine learning examples from the real world can help inquiry-based learning, as it can provide students with the latest research and resources to develop their problem-solving and critical-thinking skills.
Inquiry-based learning allows students to explore and connect the world around them, and conduct experiments in the classroom that have real-world implications. While conducting research, students move forward by continuously asking questions, which promotes a holistic learning experience. To enforce inquiry-based learning within the classroom, students are encouraged to explore the world independently.
This can be done through projects, problem-solving, and exploring complex topics. Thanks to ML technologies, machine learning examples can help students remember information better as they explore problems or create projects in a hands-on environment. In this way, students are allowed to practice their skills while also engaging with material that is interesting and relevant to them.
Machine learning examples in the field of learning motivate and engage students. Students can apply their knowledge and skills in a more focused environment, increasing their engagement and disciplinary knowledge and providing context for their learning.
This type of learning approach also helps students to build real-world ML applications in the classroom.
At Northeastern University’s School of Education, educators have been using program resources to help their students engage in real-world machine learning applications. The projects have been centered around developing tools for room management, data analysis, and predictive modeling that can be used in classrooms or school settings.
By engaging students with machine learning exercises that have real-world applications, teachers can help their students develop skills and knowledge beyond just science literacy skills. Through these projects, teachers are helping their students become well-rounded problem-solvers and critical thinkers while also inspiring them with new ideas for research projects they may want to pursue independently or within the classroom setting.
Image used under license from Shutterstock.com