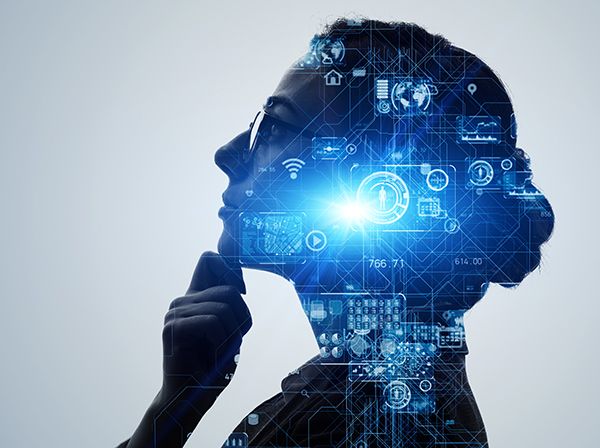
Artificial intelligence (AI) programs have existed longer than many people have been alive, with the first successful applications implemented in the 1950s. The 2020s promise greater AI potential that more businesspeople can use. Although modern AI brings powerful, accurate, and easier-to-use tools, organizations struggle to get the most value in their AI investments and achieve frictionless AI. To understand these barriers, global computational science and AI provider Altair conducted an AI Global Survey.
Notable findings include:
- 75% of organizations said that they could not get enough Data Science talent
- 63% of respondents noted that their organizations complicate work with AI-driven data tools
- 67% of respondents indicated that more than a quarter of AI projects never finish
Why do AI implementations fall short, and how can businesses succeed better? Dr. Ingo Mierswa, senior VP of product development at Altair, talked with us to understand AI frictions – inefficiencies organizations experience when implementing AI solutions – and approaches to overcome them.
Understanding AI Friction
Friction causes organizations to either never finish or to release AI deliverables late. Initially, businesses express growing excitement around AI, but “enthusiasm slows down as they discover they don’t know how to use it,” said Mierswa.
He attributes the problem to two factors:
- Acceleration: The efficiency of getting AI into production
- Collaboration: Trust and shared understanding, creating a culture of accountability
When acceleration and collaboration decrease, AI projects consume more energy – taking longer to succeed, wasting money, and producing unnecessary pain. Consequently, it becomes less likely that organizational stakeholders will buy into the next AI trial or support restarting the failed initiative.
Lack of acceleration and collaboration create drag on businesses due to the following:
- Organizational friction: Limitations in finding Data Science talent and conflicts between departments, teams, and individuals
- Technological friction: Complications in AI-driven tools an organization uses and its integration with its existing infrastructure
- Financial friction: A lack of resources to establish and evolve AI use cases because of tight budgets
When companies fail to recognize and address these three friction points, they set themselves up to poorly execute AI.
What Is Frictionless AI?
Of course, businesses want to succeed in integrating AI into their operations. However, for this to happen, organizations require the proper infrastructure to address organizational, technological, and financial frictions, including shared knowledge about AI’s role and how to use it.
Given this situation, companies must employ frictionless AI to manage risks better and pave the way for innovations. Mierswa explained:
“Sometimes people have a simplistic understanding of AI and think that upon providing some data through interacting with the AI, magic will happen, with perfect results. How can we expect this outcome when humans are imperfect, and AI models mimic human behavior through the training data provided? We need to think of AI as a game of probabilities where it functions similarly smart to human decision-makers but can offer more solutions simultaneously because of the power of its automation.”
Frictionless AI sets businesses up to win this game of probabilities by increasing acceleration and collaboration.
Winning the Game of Probabilities
Winning the game of probabilities means frictionless AI requires a data-driven culture that understands the rules. Mierswa explains through an example of a digitally transformed company – let’s call it XYZ – that wants to use AI models to detect fraud.
XYZ deals with a trillion credit card transactions annually and automates the process of detecting fraud. Occasionally, the application lets a dishonest purchase go through XYZ’s systems as though it were valid – say, 25% of all its transactions. XYZ wants to upgrade fraud detection with newer AI.
Mierswa said:
“XYZ does not need to solve its problem perfectly with the newer AI. Instead, it should do so better than the prior application. For example, if XYZ wants reliable detection of 90% of credit fraud cases through its newer AI system but only gets 80%, XYZ can acknowledge it did 5% better than the prior system, which finds fraud at 75%. So XYZ succeeded in improving fraud detection but did not meet the 90% goal.”
Yet, while implementing this AI project, XYZ has a better idea of how to move forward. Mierswa added, “If XYZ’s workforce understands AI expectations within the rules set by the game of probabilities, then the projects that look like failures can be reframed as providing valuable outcomes.”
A Common Shared Knowledge and Understanding
Having realistic expectations around AI requires that organizations share their knowledge and facilitate collaboration. Organizations need the right technologies and processes to support this level of collaboration, including moving their workforces to higher data literacy and AI literacy.
Getting to a higher data literacy and AI literacy requires that organizations retrain their workforce by teaching basic concepts of AI for the following reasons:
- Businesspeople inside a company “often have a deeper and better understanding about the business problems than the data scientist,” said Mierswa. “It is easier to allow everyone to create AI models than to look for superstar data scientists.” Hiring more data scientists means a significant learning curve in knowing about the organization’s pain points, resulting in less impact from AI technologies. Instead, bring together the businesspeople and the experts in the business with the AI models and more beneficial results.
- Mierswa noted that upskilling people in relevant roles to work with AI models leads to greater success than relying on external contractor data scientists. Short-term contractors increase risks to AI implementations because they are not invested in establishing a culture of accountability in the organization, where workers within an organization take responsibility when AI makes decisions and follows through. Mierswa said, “AI thrives on a level of collaboration, trust, and a common and shared understanding. Businesspeople and data scientists get more realistic expectations and make decisions by collaborating when this foundation is formed. Whoever owns the business problem has the power to define and reassess success. These interactions require everyone to be on board, which is easier to achieve with permanent hires.”
- “AI implementations never stop,” Mierswa said. “Production AI models will need to adapt.” In the example of XYZ’s drive to use AI to detect fraud, the context in which the AI model executes changes. Mierswa noted: “By running its AI model, that organization changes the rules of the game, resulting in the people who want to commit fraud changing their behavior. As a result, the AI models need updating to deal with this new reality.”
Additional Tips for Frictionless AI
In addition to getting the data-driven and AI-oriented culture right so everyone is aligned about what to expect, Mierswa provided some other tips for frictionless AI:
- Choose the AI project wisely: He advised, “Focus on projects with high levels of feasibility, where the odds favor quick success and a positive impact on an organization.” Mierswa explained that organizations sometimes choose the complex projects proposed by IT folks who get excited about the technology and want to explore AI technologies. But at the end of the day, AI needs to solve real-world problems for the business. Consequently, organizations should think carefully about selecting suitable projects.
- Plan to fail fast: Figure that some projects may fail. Mierswa advised, “Learn from this experience, move on, and do better next time.” He said that failing fast rather than creating friction from a struggling project makes it easier to get the next AI project off the ground.
- Get stakeholder buy-in: If an AI project has failed, stakeholder buy-in makes a big difference. First, it helps to create a data-driven culture. Second, stakeholder buy-in means less organizational friction, and paves the way toward a good outcome with the next attempts.
- Assess AI readiness: At Altair, Mierswa and others have developed a complete methodology to identify where AI can function within the organization and evaluate the supporting resources and their speed of delivery.
Such an assessment delves into how much AI models impact decisions, how well AI works, and how long it takes to get to the model collectively created versus the value it provides. From this process, companies can identify friction points by measuring efficiency and the side effect of creating a culture of accountability. This information can inform what kinds of AI projects to undertake.
Conclusion
Frictionless AI has a bright future, and Mierswa foresees that it will bring higher success rates. He attributed this optimism to the following:
- An upskilled workforce with relevant Data Science skills addressing the talent shortage of data scientists
- A democratization of AI that makes it easier for businesspeople to create solutions with models independent of requiring programming knowledge
- Deeper collaboration between data engineers and domain experts that leads to creative business solutions
All three benefits from frictionless AI will bring effective solutions in the future, leading to more of its presence and benefits for people. Mierswa concluded that AI’s magical potential happens when you bring people together and let them collaborate.
Image used under license from Shutterstock.com