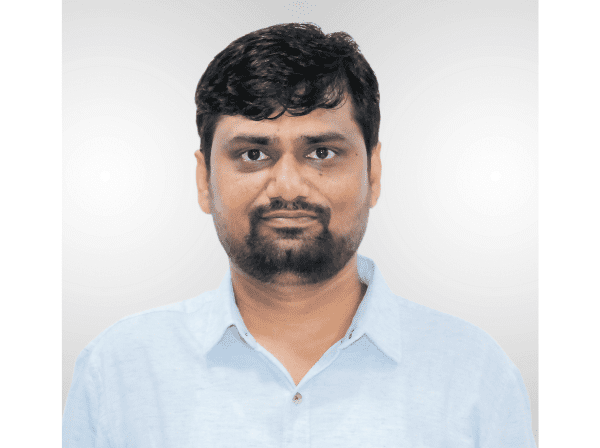
Click to learn more about author Sawaram Suthar.
Every marketer needs to know which aspect of their campaign led to a conversion or a sale. While it’s different every time for every consumer, a rough estimate is still enough for making enterprise-level decisions.
Nonetheless, to deal with the marginal errors, professionals have come up with various marketing attribution models. Using these models, you can make an informed decision on prioritizing a certain channel or touchpoint for budget allocation and other subjective purposes.
Let’s dive a bit deeper.
What Is Multi-Channel Attribution?
Multi-channel attribution is the process of keeping track of marketing channels that are responsible for conversions and sales. Through this, marketers can now quantify the value of each marketing touchpoint and its contribution to the sales cycle at large.
According to Oracle, 67% of consumers are inclined to use more than one channel to buy a product online. In a way, multi-channel attribution lends more visibility to the success and failure of each channel in the conversion journey. This, in turn, will help you revise your budget distribution in a way that is more efficient, allocating funds for channels that help you generate more revenue.
Why Multi-Channel Attribution Is Important
Digital marketers are usually under immense pressure to bridge the gap between marketing and sales and improve the business’s ROI. And that’s because the ones in charge don’t value the number of conversions as much as they value your choice of channels and whether it was the single biggest factor affecting the ROI.
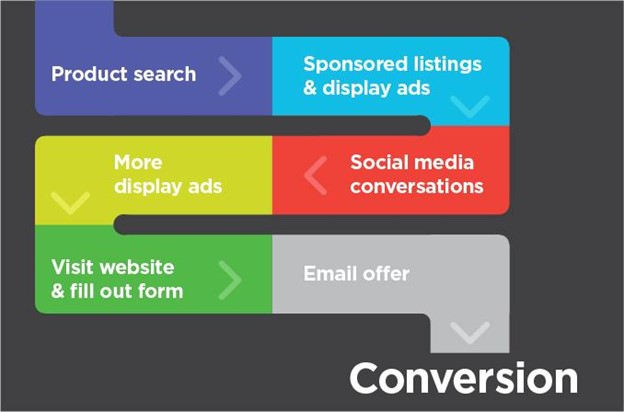
Most marketers will agree that associating marketing with conversion events is crucial for a business’s growth. And multi-channel attribution is the technique we have developed to evaluate how effective our marketing is and to find the channels that are most successful at driving sales.
From another perspective, it offers a more sophisticated alternative to the more traditional attribution techniques, which are, in fact, faulty and biased.
Choosing A Multi-Channel Attribution Model
There are various multi-channel attribution channels at play, and with each model, you’ll find varying degrees of refinement.
That said, the methods dependent on predefined rules are rather subjective and allocate credit to one or more points in the customer journey. There are also algorithmic methods that are more objective and implement known statistical and machine learning techniques to arrive at a result. Nonetheless, they also vary in terms of granularity and sophistication.
The Different Attribution Models
Whichever model you go with, it will help you determine the impact of your investment at its very core and assign authentic credit for any given KPI event to multiple touchpoints.
With this in mind, here’s an overview of the major attribution models:
Rules-Based Single-Channel Attribution
- First-Click: In this model, the very first touchpoint in the customer journey receives full credit for the KPI event. This method is often employed to measure the net effect of your marketing endeavors to spread awareness about a cause, product, service, or event. Obviously, it’s for reaching newer consumers.
- Last-Click: The last-click model prioritizes the last touchpoint in the customer journey, and is often regarded as the “default” attribution model. You can treat it as a reference model when comparing it with other multi-channel attribution models.
Rules-Based Multi-Channel Attribution
- Time-Decay: In this model, the credit is usually allowed to build up all the way from the first touchpoint leading to the last one, which receives relatively the highest credit. So, if your offering is expected to have a short consideration cycle, this model is the one to choose.
- Position-Based: Also known as the u-shaped or bathtub model, the position-based model prioritizes the first and the last touchpoints in the customer journey. The touchpoints in the middle receive lesser credit. It’s the one to go with if you intend to focus on a single marketing stage like opportunity creation or lead generation.
- Even-Weighting: Also known as the linear model, even-weighting assigns equal credit for a KPI event to all the touchpoints. You may go ahead with this model if your marketing involves longer consideration cycles and values repetitive reinforcement.
Algorithmic Multi-Channel Attribution
- Fractional Attribution: This technique makes use of machine learning to assess and allocate fractional credits to successful touchpoints, campaigns, creatives, offers, etc. This is one of the most effective models in the sense that it can go beyond channels and sub-channels to assess creatives, their placement, and even keywords. With this, you can optimize your campaigns while they’re live.
- Incremental Attribution: This model is used to measure the spike in the conversion rate caused by expenditure on advertising. It does so by taking into account your present brand awareness and demographics. This model will help you make an accurate determination of your budget allocation to the point where you can make an educated decision on the extent of budget allocation too.
While your campaigns are active, you can decide whether or not to invest any further in a particular channel.
Final Thoughts
As you understand the perks and shortfalls of each model, you should perhaps completely ignore the last-click attribution model. All it will do is drag your analysis behind, so it should only be used as an anchor or reference. On the other hand, for a more comprehensive and comparative analysis, time decay is usually the most preferred model, and then perhaps either fractional or incremental attribution models.
You can do your own research and come up with a customized model that suits your industry, although one of the above is bound to lend you a close analysis.