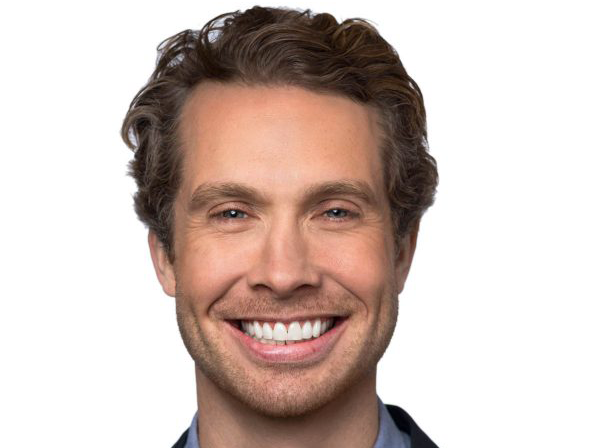
Click to learn more about author Ryan Welsh.
Almost any vendor doing machine learning (ML) is appropriating valued enterprise data for its own advantage; however, this is at the enterprise customer’s expense. Regardless of whether it’s a platform or an application using this technology, ML companies of all sizes want your data and are essentially using it for their own business gains.
Everyone knows larger companies like Google have been amassing user data on the web. The playbook is simple: Get people to use free services (social networks or search engines), collect their data, and sell it to advertisers. ML-specific vendors have their own playbook: Attract enterprise users at low or no cost, train models on their data, then sell it to other users – even competitors. What most people don’t realize is that smaller vendors – including the average five-person garage startup – are doing this too.
Customer data used to belong solely to the customer. Today, vendors need to train their ML using customer data, own the model, then return that customer data.
There are two business incentives for these vendors to effectively collect enterprise data. The first is for model training. The majority of ML vendors use the same algorithms and approaches, so there’s no competitive advantage there. The model’s training data is the true competitive differentiator, making one better than the other. The model’s value (and the vendor’s) directly relates to the uniqueness, quality, and quantity of training data and is the underlying reason why vendors are so aggressive about accumulating it.
The goal for ML vendors is to establish a data moat – proprietary data others don’t have – so they can sell ML capabilities others can’t. This attracts venture capitalists, as these models can’t be created from public data that everyone can access. Private enterprise data builds data moats, which is why it’s so expensive to protect.
The second incentive is to create ML products, not services. ML technology requires lots of time and effort to build accurate models; vendors don’t want to start from scratch with each customer. If they spend up to 18 months crafting models for enterprise customers, for example, they’re services companies – which is problematic because venture capitalists prefer product companies for their superior margins, multiples, and business valuations. Reselling models from enterprise data creates ML products, not services.
Since ML companies are gathering as much unique enterprise data as they can to succeed, CIOs must take steps to protect their data assets. If not, they’re in the unenviable position of allowing ML companies to take their data, train their algorithms on it, and sell it back to them and their competitors.
The Data Moat Myth
The problem is that data moats rarely exist outside of proprietary enterprise data because they’re more difficult to acquire than people thought. Andreessen Horowitz detailed the hardships here. Consequently, the primary way to establish a data moat is with proprietary enterprise data. For example, an insurance company might use computer vision to accelerate damage assessment and repair. Doing so would require reviewing numerous accidents, vehicle parts, schematics, and more, creating a unique dataset on which to train the underlying computer vision models. An ML vendor doing this would have a data moat because no one else has this data, enabling it to build a peerless image recognition model for this niche. Venture capitalists invest in these companies because they can corner the market.
ML vendors can exploit their data moats by selling the models trained on this data as much as possible. That also includes selling these models to the competitors of the organizations supplying the data moats to ML companies. Thompson Reuters, for example, sells its news to as many customers as it can. It would take an exorbitant amount of capital to convince it to sell news to just one customer. Data moats are the same: Vendors monetize this proprietary enterprise data by selling it to as many parties as possible.
Knowing When to Label Data
When organizations label their data and give it to ML companies, the latter acquires their human expertise and sells it in the marketplace. An app like Grammarly, for example, provides opportunities to label data by presenting grammar corrections to users. Each time people accept or reject these changes, Grammarly’s algorithms get smarter. This labeled data becomes a data moat based on end user knowledge and is analogous to the below financial analyst use case where an investment banking firm is using an ML tool and is paying its researchers top dollar for sentiment analysis.
If they override a system recommendation stating a particular news item is negative when it’s really positive, this could become proprietary labeled data for the vendor unless the firm has specific contractual language protecting its interests. Without it, vendors are paid to extract decades’ worth of financial knowledge from human experts to improve the vendor’s algorithms. Granted, the experts’ organization benefits from this improvement, but so does the entire market (including competitors) the vendor sells to. Imagine selling the model’s outputs of this data labeled by Goldman Sachs to Morgan Stanley and Credit Suisse. Unless an organization safeguards its interests, it ultimately loses in this transaction.
Ensuring Data Ownership
Enterprises must insert specific language into traditional software contracts to specify data ownership and prevent ML companies from selling valuable enterprise assets to their competition. Ownership consists of the following three aspects:
- Raw Data: Owning the raw data an organization provides to a vendor has become an established consideration for software end users. It’s particularly critical for hiring ML specialists who create and tailor models for multiple organizations.
- Labeled Data: Ensuring ownership of an organizations’ labeled data is much less obvious than doing so for their raw data, as many end-user companies aren’t clear on this point. In the investment banking use case above, human subject matter experts’ corrections of the sentiment analysis becomes a form of labeled data the organization, not the vendor, should own; and this is distinct from owning raw data alone.
- Model Weights: Many organizations don’t know they should own the ML model’s weights that are trained on their labeled data. ML models consist of coefficients, weights, parameters, and hyper-parameters that are necessary for prediction and that are estimated or learned from data. When these are estimated or learned from a company’s labeled training data, the organization is entitled to ownership of that part of the model.
Denoting ownership of the raw data, labeled data, and model weights prevents data theft by precluding vendors from selling these model parts to competitors. Vendors want the opposite: to learn on your data, generate weights for the given predictive modeling problem, then resell it to others, especially to other companies in the same industry, such as your competitors.
Intellectual Property
Much of the concern about data and model ownership for protecting end users’ interests with ML companies comes down to protecting intellectual property. Organizations should understand that vendors’ objectives about enterprise data are based on their supervised learning’s dependence on labeled training data. This dependency fuels vendors’ needs to get and exploit data via a data moat to attract investments from venture capitalists. It’s also critical to get this data to become a bona fide product company instead of a services company.
It’s important organizations realize that labeled data and model weights are an asset. And as is the case with any other asset, like IP, the value is compromised when those labels or model weights are transferred to a third party such as a vendor or a competitor. Although there may be challenges in enforcing these new contractual obligations, simply including them will make vendors think carefully about violating them and incurring extensive, costly legal or compliance repercussions.