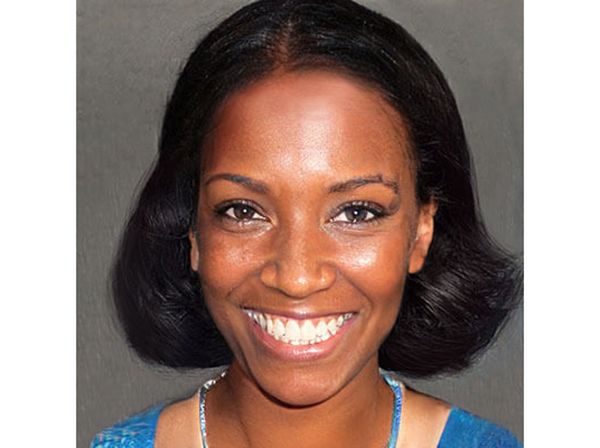
Data is the cornerstone of innovation in today’s highly digital world. However, in its raw state, data isn’t very useful as it lacks meaning and context. This is where the Semantic Web comes in – it provides a framework that imbues data with meaning, allowing machines to understand and process it just as humans do.
At the same time, advances in artificial intelligence have led to the development of algorithms and models that can analyze, predict, and make decisions based on vast datasets.
The intersection between the Semantic Web and AI offers an exciting premise. The Semantic Web allows machines to understand data, while AI enables them to analyze the data and drive actions, decisions, and innovations, leading to optimized knowledge graphs. By leveraging this convergence, it becomes possible to power smarter applications and refine user experiences in totally unique ways.
Understanding the Semantic Web
The Semantic Web is an extension of the World Wide Web that aims to make data on the internet readable and interpretable by machines. It focuses on creating meaning between the vast amounts of data on the web and enabling computers to understand the context and relationships between different pieces of data as humans do.
When the vision of the Semantic Web is fully realized, it will allow machines to interpret, relate, and act upon data in sophisticated ways. This will ultimately lead to the development of smarter search engines, more intuitive recommendation systems, enhanced AI applications, and a more interconnected digital world.
Role of AI in the Semantic Web
For centuries, humans have been obsessed with creating machines that think and act like us. This obsession ultimately led to the development of artificial intelligence (AI), which provides the necessary algorithms and frameworks to simulate human-like reasoning in machines.
However, as data became increasingly interconnected and semantic, the role of AI went beyond simulating human-like reasoning. AI has now found new applications and capabilities in conjunction with the Semantic Web.
The interconnected data furnished by the Semantic Web presents a huge opportunity for AI. Unlike isolated datasets, interconnected data offers richer context and relationships that AI algorithms can exploit. These include:
- Contextual understanding: AI models, especially those rooted in deep learning, benefit from the structured and semantic nature of interconnected data. This structure allows them to discern patterns, relationships, and hierarchies in data more effectively.
- Enhanced decision-making: Algorithms can make more informed decisions by traversing the linked data, understanding the semantic relationships, and weighing factors based on context.
- Semantic search and reasoning: AI can facilitate more accurate searches by understanding the intent and context behind queries, providing results that are not just textually but semantically relevant.
Essentially, the convergence of the Semantic Web and AI creates a harmonious blend where the Semantic Web provides a structured framework of data that AI, with its intelligent algorithms, can leverage to extract deeper insights and meanings.
Knowledge Graphs: The Bridge Between AI and Semantic Web
Knowledge graphs stand at the intersection of AI and the Semantic Web, functioning as a conduit that seamlessly merges the structured world of semantic data with the analytical capabilities of AI. These graphs map out a vast, interconnected network of entities and their relationships, making them instrumental in many modern AI-driven applications.
At their core, knowledge graphs are a structured representation of real-world entities, concepts, and their interrelations. They aren’t merely data repositories; they weave intricate connections that highlight context, significance, and semantic meanings. By doing so, they are able to provide context, enable semantic searches, and offer a unified data view.
Many of today’s most promising startups have recognized the game-changing potential of using knowledge graphs to fuse Semantic Web technologies with AI. These cutting-edge companies are creating highly intelligent applications that go beyond basic data collection and processing.
By employing knowledge graphs powered by the Semantic Web, organizations enable their AI algorithms to understand complex relationships, context, and semantic nuances in the data. This, in turn, dramatically enhances the performance and capabilities of various AI applications, from recommendation systems and search engines to natural language processing and data analytics.
Real-World Examples of the Fusion Between Semantic Search, AI, and Knowledge Graphs
The theoretical benefits of merging the Semantic Web, AI, and knowledge graphs are evident. But it’s in real-world applications where the true value of this integration shines. Below are two examples that demonstrate the tangible outcomes of employing this trio in the business and tech landscape.
Google’s Knowledge Graph
Perhaps the most well-known implementation, the Google Knowledge Graph was introduced in 2012 to enhance its search engine’s results with information gathered from various sources. It’s a structured knowledge base used to understand facts about people, places, and things and the relationships between them.
Following the implementation of the knowledge graph, Google’s search became more context-aware. Instead of merely matching keywords, it began understanding intent, providing direct answers, and suggesting relevant content.
IBM’s Watson
IBM’s Watson, famous for its Jeopardy! win, utilizes Semantic Web technologies, AI, and a vast knowledge graph to understand and respond to complex queries.
Beyond Jeopardy!, Watson has been applied in numerous sectors, including healthcare, where it assists in diagnosis and treatment suggestions by analyzing vast amounts of medical literature.
Best Practices for Integrating Semantic Web with AI
Here are some essential steps for a seamless and effective integration of the Semantic Web with AI-driven applications:
- Define clear objectives: Understand the purpose and desired outcomes of the integration. Are you aiming for better user personalization, more accurate decision-making, or some other goal?
- Data collection and cleaning: Amass relevant data sources and ensure they are clean, consistent, and devoid of inaccuracies. The quality of data directly impacts the effectiveness of the application.
- Choose the right ontologies: Depending on your domain, opt for established ontologies or design custom ones that best represent the data’s structure and relationships.
- Employ suitable AI models: Different AI models cater to different needs. Whether it’s deep learning, reinforcement learning, or another approach, choose models that align with your objectives.
Future Prospects of the Semantic Web and AI
The convergence of the Semantic Web and AI, while already transformative, is in its nascent stages. The pace of technological advancements shows that this partnership is poised for further growth and evolution.
Here are some predictions on the emerging trends and technologies from this integration.
- Enhanced personalization: As Semantic Web technologies and AI models become more sophisticated, applications will move from generic personalization to hyper-personalization, understanding user needs and preferences at an unprecedented granular level.
- AR and VR integration: With a structured Semantic Web feeding data into AI-driven AR and VR applications, users might experience a seamless blend of the real and virtual worlds, enriched with context-aware information overlays.
- Distributed knowledge graphs: With the rise of decentralized technologies like blockchain, we might see the emergence of distributed, tamper-proof knowledge graphs that ensure data integrity and trustworthiness.
- Self-healing systems: Advanced AI algorithms might enable applications to self-diagnose issues, make auto-corrections, and continuously optimize themselves without human intervention, using insights derived from the Semantic Web.
- Increased interoperability: As more systems adopt Semantic Web standards, there will be an increase in seamless data exchange and collaboration across platforms, domains, and applications, fostering a more connected digital ecosystem.
These trends show that the future is bright for devs and software engineers interested in the confluence between AI and the Semantic Web and act as a call for them to enter the job market and develop their skills.
They have good reason, too, as research indicates that AI will result in the creation of 97 million new jobs by 2025. This influx of talent will accelerate the development and adoption of these technologies, making them more robust and widely applicable. For businesses, this means greater access to advanced tools that can optimize operations, enhance customer experiences, and facilitate data-driven decision-making.
Wrapping Up
Today, we do most things online, which has resulted in vast amounts of data. However, most of it remains underutilized or misinterpreted. The fusion of the Semantic Web with AI offers a formidable solution, turning raw data into context-rich, actionable insights.
This combination doesn’t merely enhance the digital experience – it revolutionizes it, providing tools that can understand, predict, and adapt in ways that were previously considered the realm of science fiction.
For developers and businesses, the message is clear: the future belongs to enterprises that can skillfully navigate this confluence. Whether it’s about building more intelligent search engines, crafting personalized user experiences, or making informed decisions, the duo of Semantic Web and AI will lay the foundation for the next era of innovation.