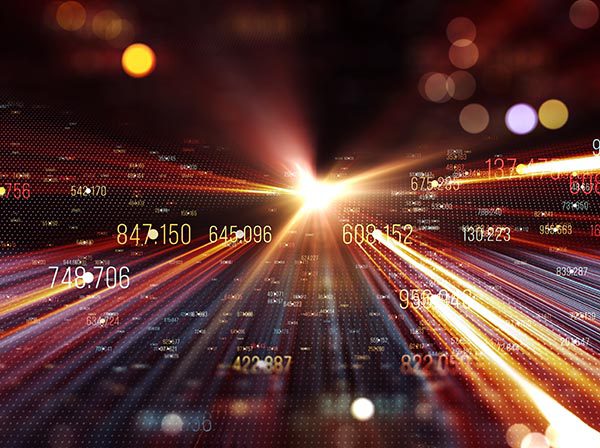
Deep learning (DL) became an overnight “star” when a robot player beat a human player in the famed game of AlphaGo. Deep learning training and learning methods have been widely acknowledged for “humanizing” machines. Many of the advanced automation capabilities now found in enterprise AI platforms are due to the rapid growth of machine learning (ML) and deep learning technologies.
This comparative post on AI, ML, and DL discusses the “ubiquitous” presence of DL in many facets of AI – be it NLP or computer vision applications. Gradually, AI- and DL-enabled automated systems, tools, and solutions are penetrating and taking over all business sectors – from marketing to customer experience, from virtual reality to natural language processing (NLP) – and the digital impact is everywhere.
Facebook Researchers Plagued with Privacy Dilemma
Here is a look back at the 2018 controversy over public demand of absolute privacy of personal data. This consumer demand is in direct conflict with Facebook’s current AI research endeavors. The AI researchers at Facebook need to “mass harvest” personal data to train learning algorithms.
Facebook realizes that the utopian concept of end-to-end encryption was indeed a myth in a research world seeking answers from piles of personal data. For future efforts, researchers are now seriously considering training algorithms on “dead data” on individual devices rather than mass harvesting personal data. In that case, Facebook engineers will install content-moderation algorithms directly on users’ phones to bypass data privacy violations.
In an AI Multiple article, the author details several unique DL methods such as self-supervised learning, FLS, and GAB-based data augmentation, which may survive the controversies surrounding the shelf lives of many deep learning methodologies.
Another severely limiting characteristic of DL-enabled solutions is that the learning algorithms still cannot provide detailed reasons for their choices, which can provoke users to accept decisions provided by AI tools blindly and then concoct “fake” explanations for any rejected answer. That is not very encouraging for decision-support solutions!
Democratization of Deep Learning in Five to 10 Years
The AI industry insiders have, for many years, suggested that entire ML environment should be democratized. DL tools will become a standard part of the developer’s toolkit. Reusable DL components, incorporated into standard DL libraries, will carry the training characteristics of its previous models to speed up learning. As automation of deep learning tools continue, there’s an inherent risk the technology will develop into something so complex that the average developer will find themselves totally ignorant.
New Predictions About Deep Learning
Out of the top 10 predictions made about deep leaning in 2022, here are some worth watching this year:
- Integrated hybrid models
- Use of DL in neuroscience
- General adversarial networks (GAN)
- Use of edge Intelligence
- NLP at the next level
Deep Learning Applications of the Present and Future
Google was the pioneer in pursuing deep learning in marketing. Google’s acquisition of DeepMind Technologies shook the business world. Google’s mission is to make DL a serious solution for search marketers who care about SEO.
The most notable application trend in the real world of ML technologies and tools is that they are beginning to transform one business at a time “from chatbots and digital agents in CRM to virtual reality (VR)-powered shop-floor demos.” The future ML technologies, which include DL, must demonstrate learning from limited training materials, and transfer learning between contexts, continuous learning, and adaptive capabilities to remain useful.
Deep learning’s powerful technology has been utilized many times over in popular applications like speech and facial recognition, or image classification. The more recent applications and use cases include fake news detection, predictive models for health care, and automatic image and handwriting generation.
Future Trends in a Nutshell
Some of the primary trends that are moving deep learning into the future are:
- Current growth of DL research and industry applications demonstrate its “ubiquitous” presence in every facet of AI — be it NLP or computer vision applications.
- With time and research opportunities, unsupervised learning methods may deliver models that will closely mimic human behavior.
- The apparent conflict between consumer data protection laws and research needs of high volumes of consumer data will continue.
- Deep learning technology’s limitations in being able to “reason” is a hindrance to automated, decision-support tools.
- Google’s acquisition of DeepMind Technologies holds promise for global marketers.
- The future ML and DL technologies must demonstrate learning from limited training materials, and transfer learning between contexts, continuous learning, and adaptive capabilities to remain useful.
- If deep learning technology research progresses in the current pace, developers may soon find themselves outpaced and will be forced to take intensive training.
Interested in a Career in Deep Learning?
Depending on whether you are a complete newbie or already experienced in other Data Science fields, you may be familiar with some of these useful tips for launching a career in deep learning:
- Explore the wide field of deep learning and narrow down your focus area.
- With a specific focus area in mind, the next step is to cultivate relevant programming languages. For example, if your focus area is ML algorithms, then developing Python language skills will be helpful.
- It is equally important to brush up on your analytical skills continuously. For this, you may need to review training sites and attempt their exercises.
- Finally, reviewing actual job descriptions on job sites may enhance your knowledge of deep learning job roles and responsibilities.
Image used under license from Shutterstock.com