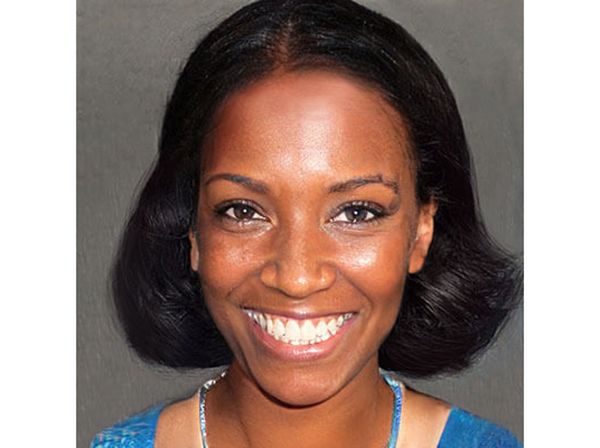
While AI’s ubiquity is becoming increasingly evident through everyday tools like chatbots, smart cameras, and smart content generation, there’s an expansive universe of less recognized but highly potent advancements poised to redefine how data scientists interact with and leverage the burgeoning volume and complexity of datasets. Emerging AI trends such as natural language processing, reinforcement learning, and computer vision are all set to bring in the next frontier of Data Science, providing powerful tools that will enable professionals to extract maximum value from their data.
These tools promise to streamline existing processes and offer fresh perspectives on how we view and understand data. To showcase them, we will go over some key lesser-known trends in AI that we believe will fundamentally reshape the field of Data Science in 2023.
The Rise of Automated Machine Learning (AutoML)
AutoML stands at the forefront of emerging AI trends, poised to bring revolutionary changes to the field of Data Science. The technology greatly simplifies the creation of machine learning models by automating complex tasks like model selection, hyperparameter tuning, and feature engineering.
The adoption of autoML will not only enhance the democratization of AI, enabling professionals with minimal ML expertise to develop effective models, but it will also usher in a new era of efficiency and productivity in Data Science.
Decoding AI Decisions with Explainable AI (XAI)
As AI models become more complex, their decision-making processes often become more opaque. This frustrating issue has given birth to the trend of explainable AI (XAI) models.
XAI aims to demystify how AI reaches its decisions, providing new levels of transparency and comprehensibility, which are increasingly crucial as AI continues to find new applications in critical sectors such as healthcare, finance, and law.
For data scientists operating in these growing sectors, thoroughly understanding XAI will be indispensable for effective communication and regulatory compliance.
Unleashing Potential with Reinforcement Learning (RL)
Although not a novel concept, reinforcement learning (RL) is rapidly gaining traction in Data Science. From ad placements to recommendation systems, RL’s adaptive and personalized approach promises to bring transformative changes by optimizing various processes.
This year, in particular, is expected to see the rapidly accelerated adoption of RL as businesses realize and harness its untapped potential.
Balancing Insights and Privacy with Federated Learning
The growing demand for robust privacy and data security across all areas of IT has led to the rapid emergence of a concept known as federated learning. This technique allows AI models to learn from multiple decentralized devices or servers holding local data samples, bypassing the need to exchange sensitive data.
As privacy regulations continue to tighten worldwide, federated learning could offer data scientists a way to unlock valuable insights while maintaining user privacy.
Unlocking Insights with Graph Neural Networks (GNNs)
Graph neural networks (GNNs) are becoming increasingly more relevant, with more complex, interconnected datasets becoming available.
Unlike traditional AI models, GNNs are equipped to handle data that form a graph, making them an invaluable asset for data scientists working on tasks such as social network analysis, recommendation systems, and even molecular chemistry.
Accelerating Computation with Quantum Machine Learning
Quantum machine learning, also known as QLM, is a key trend in AI that has already impacted several areas of Data Science. This exciting intersection of machine learning and quantum physics is set to bring significant disruptions to the industry as a whole, making it important for companies of all kinds and sizes to watch.
With quantum computers’ potential to perform complex computations faster than classical computers, they can accelerate data processing and analysis tasks, a development that every data scientist should watch.
Simplifying Coding With AI
The increasing integration of AI into coding tasks is transforming how software development and Data Science function.
Prominent examples of this technology, like GitHub’s Copilot, demonstrate the potential to automate routine coding tasks, improve code quality, and reduce bugs. This trend promises a significant boost to efficiency in the field.
Overcoming Data Challenges with Synthetic Data Generation
Synthetic data generation, which involves creating artificial data that closely mimics real data, provides a solution to challenges in data collection.
Particularly beneficial when privacy concerns limit data availability or when rare events are hard to capture during data collection, synthetic data generation could potentially redefine how data scientists train their models.
Furthermore, it offers the potential for improved testing scenarios, enabling rigorous evaluation of machine learning models under diverse conditions, thus enhancing their robustness and reliability.
Enhancing Models with Data Augmentation
Another trend known as data augmentation, which involves creating new data by modifying existing datasets, holds immense promise in improving model performance and robustness.
This emerging AI trend is especially beneficial in fields with limited data availability, such as medical imaging. Data augmentation is expected to become an essential tool for data scientists aiming for more accurate and robust models.
Prioritizing Privacy and Efficiency with Edge AI
The proliferation of Internet of Things devices has led to the emergence of edge AI, which involves processing data directly on the device rather than in the cloud.
Offering plenty of notable benefits in terms of speed, privacy, and cost-effectiveness, edge AI is an important trend that will influence how and where data processing tasks are performed, making it crucial for data scientists to keep their eyes on.
Refining Human Language Understanding with Natural Language Processing (NLP)
While NLP is not a new field, its continuous evolution and increasing sophistication make it a critical trend to watch.
Advances such as transformer models and improved sentiment analysis offer data scientists powerful tools to understand and generate human language, revolutionizing data analysis and interaction and opening new doors of opportunities.
Pioneering Rapid Developments in AI for Computer Vision
Computer vision has seen a surge in popularity over recent years, and the momentum is expected to carry forward into 2023 with a slew of advanced technologies on the horizon. A case in point is the recent release of YOLOv8, which proves that innovation in this field is far from over, heralding a year of continuous progress and enhancements.
Synthetic data generation, an approach we discussed earlier, plays a pivotal role in computer vision, underpinning many of the advancements in this field. Its rapid growth underscores its significance in creating diverse and complex datasets for training increasingly sophisticated computer vision models.
The Impact of AI Trends on Data Science Going Forward
With the Data Science platforms market projected to rise from $96.3 billion in 2022 to a staggering $378.7 billion by 2030, growing at a compound annual growth rate (CAGR) of 16.43%, it’s clear that Data Science is a discipline of immense importance and potential.
This projected growth is driven by rapid advancements in Data Science, spanning big data, predictive analytics, artificial intelligence, and the increasingly sophisticated theoretical and practical uses of data and technology.
As businesses try to remain competitive and capitalize on emerging opportunities, they are deploying Data Science more comprehensively than ever. Harnessing these AI trends will be key to riding the wave of this digital transformation, unlocking new possibilities, and propelling the field of Data Science to uncharted territories.