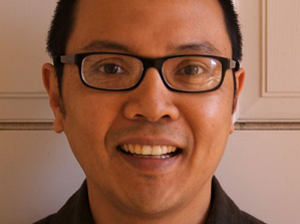
Click to learn more about author Ben Lorica.
It’s no surprise that Natural Language Processing (NLP) technology has been gaining steam in the enterprise over the last several years. With the power to predict disease, sift through hundreds of resumes to find a perfect job candidate, help identify and resolve customer service issues, and even help automate mundane litigation tasks for lawyers, the industries and applications in which NLP can be useful are seemingly endless.
While it’s nice to reflect on the progress made in this subset of artificial intelligence, there’s still much to be learned and applied – especially in the enterprise – to truly reap all the benefits NLP has to offer. To explore this further, John Snow Labs issued the first 2020 State of NLP Survey, which gives a comprehensive view into where the enterprise is with NLP adoption currently, and what these promises and pitfalls mean for the future.
Here are the five main takeaways IT and technology leaders should keep in mind as they approach NLP implementation or expansion.
1. NLP Spending is Increasing, Despite Shrinking IT Budgets
Perhaps the most promising finding from the survey is that, despite the global coronavirus pandemic and shrinking IT budgets as a result, spending on NLP did not decrease. In fact, it’s quite the opposite: Respondents indicated spending was increasing consistently, and in many cases, significantly. 53% of technical leaders indicated their NLP budget was at least 10% higher compared to 2019, with 31% stating their budget was at least 30% higher than the previous year. At a time when overall IT spending is down and many businesses are focused solely on ‘mission-critical’ technology, it’s safe to say NLP fits into this category.
2. Accuracy is a Key Criteria, but also a Key Challenge
Accuracy is paramount for all users of NLP technology, but especially in highly regulated industries, such as healthcare and financial services, where compliance and safety are of the utmost importance. So, it’s no surprise more than 40% of all respondents cited accuracy as the most important criteria they use to evaluate NLP libraries. That said, accuracy is also the most frequent challenge cited by all respondents. This is true for organizations in all stages of adoption, from those using NLP (21%), to those exploring it (31%). Additionally, 51% of respondents cited accuracy as a key challenge for users of popular NLP cloud services, such as Amazon and Google.
3. NLP Cloud Services are Slow to Service Market Needs
Continuing on with cloud services, 77% of all survey respondents indicated that they use at least one of the four NLP cloud services listed in the survey (Google, AWS, Azure, IBM), with Google’s service topping the list. Google Cloud is particularly popular among respondents who are still in the early stages of adoption. Despite the popularity of cloud services, respondents cited cost as another key challenge, along with concerns about extensibility, since so many NLP applications depend on domain-specific language use and cloud providers have been slow to service these market needs.
4. Spark NLP and SpaCy Voted Most Popular
More than half of all respondents (53%) used at least one of the top two libraries: Spark NLP and spaCy, and a third of all respondents stated they use Spark NLP, making it the most popular library in the survey. This varied slightly in several key industry sectors: Healthcare (Spark NLP), Technology (spaCy), Financial Services (nltk). The industry preferences are not surprising, given that John Snow Labs created Spark NLP specifically for healthcare users. What is surprising is that a rookie (3-year-old) library dominates more than half of the healthcare vertical – a generally slower-moving industry with well-established competitors.
5. Classic Applications of NLP are Still the Most Used
Given the mix of healthcare and finance respondents, it was predictable to see that document classification, named entity recognition (NER), and sentiment analysis topped the list of use cases cited by all respondents. NER models seek to automatically extract named entities – company name, location, etc. – from unstructured text. More than a third (39%) of all technical leaders stated that they also use NLP for entity linking and knowledge graphs. Data from files and databases topped the list of data sources used to run the aforementioned applications, with 61% of technical leaders stating that they used files, such as pdf, txt, docx, etc., for their NLP systems.
It’s encouraging to see that enterprise organizations understand the value of NLP – a vote of confidence made clear by increasing financial investments in the technology. But despite NLP spending, it’s evident that there are still hurdles to face when it comes to accuracy and scalability, especially with NLP cloud services. These topics and real life use cases will be explored further at the upcoming NLP Summit (Oct. 6-16). This is the first applied NLP event, and it’s free to those interested.
A lot can change in a year, and It will be interesting to see the benchmarked survey results, post-pandemic, election, and continual progress in the evolving tech space. Until then, explore your options, make sure it’s a viable and scalable option for your organizational and regulatory compliance needs, and keep improving your models.