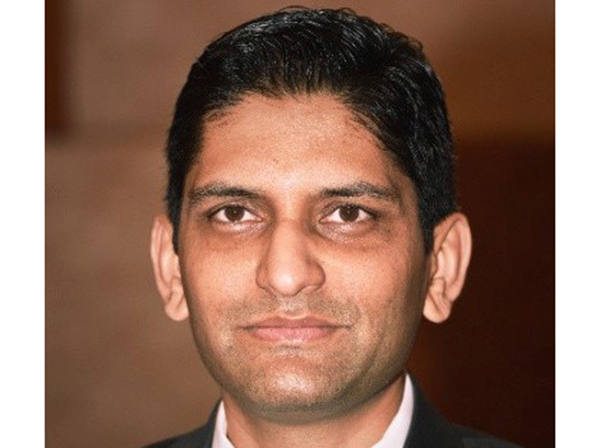
In the era of globalized markets, burgeoning international trade, complex financial systems, ever-evolving compliance and regulatory landscapes, and rapid technology advancement, white-collar crime has unfortunately seen a significant uptick in scale, variety, and sophistication. Whereas white-collar crime used to conjure images of high-flying executives stealing from company coffers, the modern landscape is much more complex, encompassing misconduct of all shapes and sizes, such as international bribery and corruption, sophisticated money laundering, health care fraud, complex accounting and financial reporting fraud, securities trading schemes, and cybercrime, to name but a few.
Today’s white-collar criminals are smarter and more technology-savvy, often exploiting complex and siloed systems and circumventing often archaic fraud- and compliance-monitoring solutions used by corporations and government entities. And while bad actors are effectively leveraging the massive swathes of data to their advantage in obfuscating investigators and avoiding detection, organizations are struggling to store, manage, and utilize data effectively to investigate and prevent compliance issues, fraud, waste, and abuse.
As an added challenge, regulators have raised the bar and expect corporations to employ data-driven methods to tackle white-collar crime.
The good news is that data science and big data analytics are catching up fast and already offer a plethora of solutions and techniques to prevent, detect, investigate, and remediate white-collar crime. Let’s talk about some of the best practices organizations and government agencies have employed to tackle white-collar crime using data science and big data analytics.
- Aggregating siloed data: While analyzing individual data sets can be informative, linking disparate data sets together to identify trends and correlations can be transformative for identifying corporate misconduct.And while stitching together scattered data is never trivial, advancements in data engineering have made this task a lot easier. Software solutions are available today to integrate data from the commonly used data sources, such as ERP system data (such as vendor payments), CRM/sales databases, HR and payroll systems, and other third-party sources. Many available tools have visual “drag-and-drop” functionality for the most common use cases. For more complex integrations, tools can be used without significant customized programming to allow business users to configure and modify variables on the fly with ease.
- Leveraging non-traditional data sources: Internal data, such as system access logs, building access data, employee surveys, performance appraisals, and even external data such as social media information can provide valuable insights and help plug gaps in fraud and compliance investigations and monitoring. For example, social media analytics is being increasingly used to cross-reference facts relevant to an investigation (e.g., the who, what, where, why, and when an event occurred). Analyzing the date proximity of events, attendees, locations, and sentiments referenced on social media in association with transactions in company systems, such as reimbursable employee expenses, can provide a “smoking gun” to investigators of fraud and misconduct.
- Applying rule-based analytics: Rules-based tests are a tried-and-true method to identifying red flags or statistical anomalies to steer investigators toward potential misconduct or compliance issues. Once a consolidated data repository across multiple data sources has been created, rules-based tests to identify specific attributes of data records (such as keywords, monetary metrics, statistical outliers, user information) can help identify correlations, anomalies, and high-risk cohorts, such as transactions, employees, vendors, departments, or geographic locations.
- Risk scoring: This is a commonly used method to distill the results of data-driven tests whereby data that “hits” on certain parameters can be aggregated so higher-risk items of interest, be it a person, payment, vendor, customer, etc., are bubbled to the surface. For example, if an employee has anomalies with reimbursable expenses, such as certain keywords in free text comments, duplicate, or just below approval threshold expenses, they would be scored as “higher risk.” Those results can then be correlated to tests on other data sources, such as training system reports, time-keeping systems, or compliance department data, to create a composite risk score of the individual.
- Predictive modeling: As organizations become more analytically mature with easy access to reliable and real-time data, the sophistication of anomaly detection improves dramatically with the usage of machine learning and artificial intelligence. At that stage, the solutions to detect white-collar crime often mimic the advanced fraud detection techniques used in the payments and e-commerce world (think real-time credit card fraud alerts one receives) Trends and patterns gleaned from past fraudulent transactions and behaviors can be leveraged to create predictive solutions that enable early identification of potential fraud.
- Creating dynamic visualizations: Interactive visualizations for synthesizing large amounts of complex information and presenting it in an easily understandable format is a critical step in any analytics solution. Features such as geographic mapping, temporal analyses, relationship charts, and risk-scoring graphics enable effective data storytelling and provide visible, tangible evidence of high-risk activities that have either happened or are likely to happen. While most off-the-shelf dashboarding tools are sufficient for the most common visualization use cases in compliance and risk, some organizations choose to invest in bespoke web-based User Interface (UI) solutions that offer maximum flexibility, speed, and accuracy.
Perhaps the most tangible way of understanding how data science and big data analytics can be used in combatting white-collar crime is by real-world example. Following a whistleblower allegation regarding misreporting of time-keeping activities by certain employees, we were engaged by a large government entity to design and execute forensic data analytics to identify indicators of possible fraud, waste, and abuse. Using a combination of rules-based, statistical, and visual analyses, and composite risk-scoring, we identified time reports and individuals with a heightened risk of reporting false hours. With custom queries, we correlated information from multiple distinct data sets, including detailed daily time report data, building access log data, and a dedicated system that recorded communications between employees in the field and home office. This analysis allowed us to corroborate hours worked, and more importantly, identify those that were unsupported by other corroborating evidence. The client was able to seek recovery of losses, take action against individuals, and remediate control weaknesses with its time-keeping system.
In the fight against corporate misconduct and various forms of white-collar crime, the devil is most certainly in the details. Data science and big data analytics are must-have tools in any organization’s arsenal.