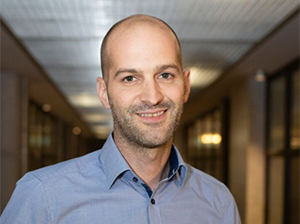
In an increasingly data-driven world, companies worldwide have transformed the way they operate. Much of this is thanks to their ability to access a volume of data that was not available in the past. However, more recently, organizations have realized that simply collecting data is not enough, with many struggling to master the language of data. If they are to turn this wealth of information into actionable insights at scale, they need people within the business who can provide the context of what the data is telling you. This is where data storytelling comes into the picture.
Organizations are increasingly having to rely on data storytelling to help their organizations leverage their data assets in the most impactful and efficient ways possible. Data storytelling enables businesses to glean the most accurate, meaningful, and actionable insights from data. In doing so, it provides organizations with the context for painting a clearer picture of what the data actually means so that important insights don’t fall flat or slip through the cracks. In short, data storytelling explains what the data is showing and why it matters. In fact, my company’s own research found that nearly all (92%) of IT and data decision-makers surveyed agreed that storytelling is an effective means of delivering the findings of data and analytics.
Given this trend, there is a major demand for data storytellers across all industries – most notably health care, financial services, and retail, among others – with companies seeking to build best-in-class data teams with people from different backgrounds with various skillsets. One such role is that of a data scientist storyteller. Data scientists are the specialists capable of taking billions of bytes of structured and unstructured data and creating concise, tangible business insights, weaving them into a compelling narrative that can have a real impact on the bottom line of any business.
As data storytelling becomes more of a priority for organizations, these modern data scientists need to have more than just technical knowledge and advanced data science skills, but also the ability to interpret data for business-focused stakeholders. After all, data scientists can have all the data in the world, but without grounding the numbers in reality or context, the meaning, learning, or insight can be lost.
Data scientists who have been successfully implemented data storytelling across their entire organizations typically follow four key steps:
1. Empower knowledge workers to become more data-savvy so they can also interpret the data along with their more technical counterparts: Realize that data storytelling isn’t just about being able to work a data platform. It’s also about data literacy skills and the ability to communicate more widely – understanding the business context, the importance of numbers, and then breaking those down into a pithy, compelling narrative.
2. Embrace new smarter self-service tools that help both data scientists and knowledge workers turn data into stories: Consider implementing new easy-to-use business intelligence tools, self-service data exploration and data preparation solutions, and auto-machine learning tools that enable employees to interpret complex information on their own, act on their findings, and then let them tell their own data stories.
3. Make ongoing employee education a priority: Employees need continuous ongoing education to keep up with data trends. This, in turn, will also help improve data literacy at all levels of the business. Organizations must provide employees with faster access to tools that help them understand the story behind the data, and critically, how to best communicate that story effectively to the C-suite and key stakeholders.
4. Modernize the data software stack: This can be done by introducing self-service tools and systems that can be operated without any need for tuning or administration, and a powerful analytical database or data warehouse that can scale with the additional use cases and user groups. This means that far fewer resources will be needed to cope with increasing data demands. Often, knowledge worker teams want to become data-driven, but the sheer number of those requirements frequently lead to the central data engineering teams becoming the bottleneck. Smarter self-service tools will solve that problem while offering additional ways of organizing the data ownership.
Translating data insights into a story that anyone can relate to and understand will make it easier for all employees to buy into data-driven decision-making – a key strategy that will have a highly positive impact on an organization’s bottom line. Furthermore, “automated decision-making,” also known as operational business intelligence, is one of the most impactful ways to optimize businesses and make them more competitive and nimbler in an increasingly challenging global environment. Moving forward, this approach will go beyond mere reporting and dashboarding of the past and evolve to more advanced predictions and prescriptive data analysis. By embracing data storytelling now, organizations can leverage innovative predictive analytics that could transform their businesses in the future.