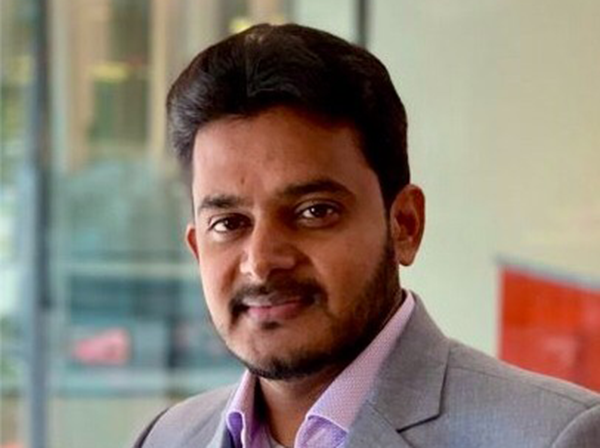
We exist in a diversified era of data tools up and down the stack – from storage to algorithm testing to stunning business insights. In fact, it’s been more than three decades of innovation in this market, resulting in the development of thousands of data tools and a global data preparation tools market size that’s set to expand at a compound annual growth rate (CAGR) of 18.6% from 2021 to 2028. This begs the question: With all these tools at our disposal, as well as ample awareness of the importance of data, why is Data Quality still so hard to achieve?
For starters, even with all our focus on data, there are still several factors creating cracks in our modern data pipelines. What are they, and how can we address them to help organizations align and ultimately achieve higher Data Quality?
Exploding Data Volume
One major factor putting pressure on our data pipelines is the sheer volume and variety of data at our disposal. The amount of data that exists has exploded, growing exponentially in recent years. In fact, it is estimated that 90% of the world’s data was generated in the last two years alone. And by 2025, global data creation is projected to grow to more than 180 zettabytes. This indicates that organizations are collecting an ever-increasing amount of data.
Even if at one point an organization felt that it had achieved high Data Quality, it’s incredibly unlikely that they’ve been able to maintain that level of assurance as the volume of data has grown. And with the advent of big data and the proliferation of data sources, such as social media, IoT devices, and sensors, organizations are grappling with enormous datasets that also have varying structures and formats. This diversity makes it challenging to maintain consistent Data Quality standards across the board – especially as new structures and formats continue to be created.
Persistent Silos Separating Data
Second, it’s a well-known issue that data is often siloed within organizations. Different departments and teams collect and manage their data independently, leading to fragmentation and a lack of standardized practices for Data Quality. As individual departments continue to add on more and more tools, it becomes even more challenging to get those tools to integrate at the department level and the organization level, resulting in inconsistencies and errors that are difficult to detect and rectify. Furthermore, when data is siloed, each tool introduces new transition points across each stage of the data pipeline, from data ingestion and transformation to analysis and reporting. This can compromise Data Quality – creating new opportunities for errors to creep in – and identifying the source of issues can be like searching for a needle in a haystack.
Difficulty Creating a True Data Culture
Finally, Data Quality is not just a technical issue; it’s also a cultural and organizational challenge. Currently, most data tools are designed for use by data professionals working with data, not for the average user. Because they are often used by data analysts or data scientists to manipulate data in order to gain insights or create reports, they may require coding knowledge or prior experience using other data tools for data cleansing, data transformation, data visualization, and data analysis. This may have worked well when data professionals were the only ones using data tools, but that is no longer the case. Modern Data Quality requires collaboration across departments and teams – not just IT teams or data scientists. Without a real culture of Data Governance and accountability, Data Quality issues will persist.
How To Fix the Cracks in Your Data Quality Foundation
To correct these issues and help guide Data Governance, security, and IT teams toward better Data Quality and integrity, I recommend a three-pronged approach.
- Start by improving the data itself: Of course, data is just one piece of the Data Quality puzzle, but it’s one of the most important factors. Consider exploring new Data Quality tools and platforms that can automate data profiling, cleansing, and validation and help identify and rectify data issues more quickly and efficiently. Implementing continuous data monitoring and auditing processes can also detect anomalies and errors in real-time before inaccuracies move downstream and cause greater negative business impact.
- Facilitate cross-silo data use: There’s no getting around persistent data silos, but you can make them easier to manage. Introducing a robust data catalog can make it easier to search for data across multiple sources and find the most accurate, up-to-date data. Implementing metadata management and data lineage tracking can also make it easier to uncover data sources and dependencies, facilitating Data Quality assessment. To address the issues caused by multiple data transformations across disparate tools, I suggest integrating data integrity checks into the data lifecycle itself. By introducing integrity checks at every transition point across tools or platforms, you’re able to catch data issues or errors before they’re ingested into another tool, thus further enhancing Data Quality.
- Build a data-focused culture: Lastly, take steps to educate every member of the organization on both the value of Data Quality and the individual’s role in ensuring it. Establish clear Data Governance policies that encompass Data Quality standards, data ownership, and data stewardship roles – and assign accountability for Data Quality at all levels of the organization. If this is fairly new for your organization, you may need to invest in training and educating staff on the importance of Data Quality and security, as well as best practices for maintaining them. But by promoting collaboration between disparate teams, you can ensure that Data Quality remains a top priority across the board.
There is no doubt that Data Quality will continue to remain a challenge for many organizations, thanks to the evolving data landscape, persistent data siloes, and a general lack of a true data culture in many organizations. However, with a concerted effort to establish clear governance, employ modern Data Quality tools, foster collaboration, and prioritize Data Quality as a cultural imperative, organizations can make significant strides in ensuring the integrity of their data in the modern data pipeline era.