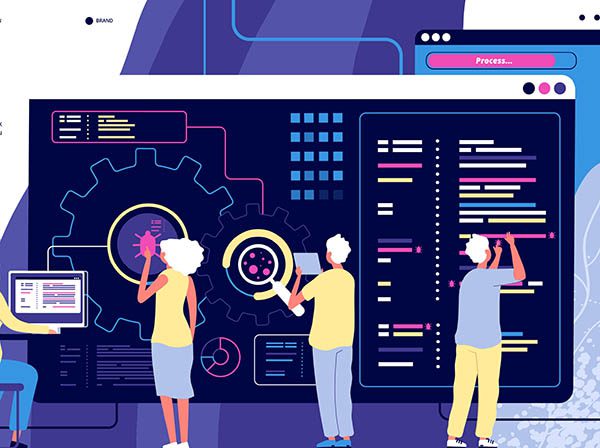
The term “Data Quality” focuses primarily on the level of accuracy possessed by the data, but also includes other qualities such as accessibility and usefulness. Some data isn’t accurate at all, which, in turn, promotes bad decision-making. Some organizations promote fact-checking and Data Governance, and, as a consequence, make decisions that give them an advantage. The purpose of ensuring accurate data is to support good decision-making in both the short term (real-time customer responses) and the long term (business intelligence). Data is considered to be of high quality when it correctly represents reality.
With this in mind, executives and decision-makers must consider the quality of their data, and that potential inconsistencies may result in unreliable business intelligence insights. For example, when working with predictive analytics, projections should be based on accurate and complete data. When data is not accurate and complete, projections will have only limited value, and false assumptions may seriously damage an organization. Issues to consider in Data Quality include:
- Accessibility
- Completeness
- Objectivity
- Readability
- Timeliness
- Uniqueness
- Usefulness
- Accuracy
Some organizations perform significant research and establishing good Data Quality may include developing specific protocols for research methods. These behaviors would be part of a good Data Governance program.
The Origins of Data Quality
In the year 1865, Professor Richard Millar Devens established the term “business intelligence” (abbreviated to BI) in his Cyclopædia of Commercial and Business Anecdotes. He used the term to describe how Sir Henry Furnese gathered information, and then acted on it before his competition did, to increase his profits.
Much later, in 1958, Hans Peter Luhn wrote an article describing the potential for gathering BI by way of technology. The modern version of Business Intelligence uses technology to collect and analyze data, and transform it into useful information. This information is then used “before the competition” to provide a significant advantage. Essentially, modern business information is focused on using technology to make well-informed decisions quickly and efficiently.
In 1968, people with extremely specialized skills were the only ones who could translate the available data into useful information. At the time, data taken from multiple sources would normally be stored in silos. Researching this kind of data typically involved working with fragmented, disjointed information, and produced questionable reports. Edgar Codd recognized this problem, and presented a solution in 1970, which changed how people thought about databases. His solution suggested creating a “relational database model,” which gained tremendous popularity, and was adopted worldwide.
Database Management Systems
Decision support systems (DSS) are described as the earliest database management system. Many historians have suggested modern business intelligence is founded on the DSS database. In the 1980s, the number of BI vendors grew substantially. Business people had discovered the value of big data and modern business intelligence. A broad assortment of tools was created and developed during this time, focusing on the goals of accessing and organizing the data in more efficient and simpler ways. Executive information systems, OLAP, and data warehouses are examples of some of the tools developed. The importance of Data Quality helped to spark the development of relational databases.
Data Quality-as-a-Service (DQaaS)
In 1986, before inexpensive data storage, huge mainframe computers were maintained that contained the name and address data used for delivery services. This allowed mail to be routed to its proper destination. These mainframes were designed to correct the common misspellings and errors in names and addresses, while also tracking customers who had died, moved, gone to prison, divorced, or married.
This was also the time that government agencies made postal data available to “service companies” for cross-referencing with the NCOA (National Change of Address) registry. This decision saved several large companies millions of dollars, because manual corrections of customer data was no longer necessary, and wasted postage costs were avoided. This early effort at improving data accuracy/quality was initially sold as a service.
The Internet Offers a Flood of Data
In the late 1980s and early 1990s, many organizations began to realize the value of data, and data mining. CEOs and decision-makers increasingly relied on data analysis. Additionally, business processes created larger and larger amounts of data from different departments for different purposes. Then, on top of that, the internet became popular.
In the 1990s, the internet became extremely popular, and relational databases owned by large corporations could not keep up with the massive flow of data available to them. These problems were compounded by the variety of data types and non-relational data that developed during this time. Non-relational databases, often referred to as NoSQL, came about as a solution. NoSQL databases can translate a variety of data types quickly and avoids the rigidity of SQL databases by eliminating “organized” storage, and offering more flexibility.
Non-relational databases developed as a response to internet data, the need to process unstructured data, and the desire for faster processing. NoSQL models are based on a distributed database system, using multiple computers. Non-relational systems are faster, organize data using an ad-hoc approach, and process significant amounts of different data types. For general research, NoSQL is the better choice when working with large, unstructured data sets (big data) than relational databases because of their speed and flexibility. The term “big data” became official in 2005.
Three Basics for Controlling Data Quality
There are currently three basic methods for achieving true Data Quality. They help significantly in providing accurate data that can be used in gathering useful business intelligence, and in making good decisions. These approaches for developing and maintaining Data Quality are:
- Data profiling is the process of assessing the integrity and condition of the data. It is generally recognized as an important first step in controlling an organization’s Data Quality. This process emphasizes transparency of the data, including metadata and sources.
- Data Stewardship manages the data lifecycle from its curation to its retirement. Data stewardship defines and maintains data models, documents the data, cleanses the data, and defines its rules and policies. These steps help to deliver high-quality data to both applications and end users.
- Data preparation involves cleansing, standardizing, enriching, and/or transforming the data. Data preparation tools offering self-service access are now being used to accomplish tasks that used to be done by data professionals.
Data Governance
By 2010, data volume and complexity continued to expand, and in response, businesses became more sophisticated in using data. They developed methods for combining, manipulating, storing, and presenting information. This was the beginning of Data Governance.
Forward-thinking companies formed governance organizations to maintain the business’ data, and developed collaborative processes to use the data necessary for business. But more significantly, they developed a “policy-centric approach” to Data Quality standards, data models, and data security. These early groups ignored visions of ever-larger and more complicated repositories, and focused on policies that defined, implemented, and enforced intelligent procedures for the data. One procedure makes it acceptable to store the same type of data in multiple places, providing it adheres to the same policies. As a result, businesses took more and more responsibility for their data content. Data is now widely recognized as a valuable corporate asset.
Data Governance covers the overall management of data in terms of usability, integrity, availability, and security. A good Data Governance program has organized a governing body of well-informed individuals and developed responses for various situations. Data Governance behaviors must be clearly defined to effectively explain how the data will be handled, stored, backed up, and generally protected from mistakes, theft, and attacks. Procedures must be developed defining how the data is to be used, and by which personnel. Moreover, a set of controls and audit procedures must be put into place that ensures ongoing compliance with internal data policies and external government regulations, and that guarantees data is used in a consistent manner across multiple enterprise applications. Machine learning has become a popular way implementing Data Governance.
Data Governance reflects the strategy of the organization, with Data Governance teams organized to implement new policies and procedures when handling data. These teams can be made up of data managers and business managers, as well as customers using the organization’s services. Associations that are committed to promoting best practices regarding Data Governance processes include DAMA International (Data Management Association), the Data Governance Institute, and the Data Governance Professionals Organization.
Data Quality Tools
Stand-alone Data Quality tools will often provide a fix for one situation, but will not solve multiple problems over the long haul. Finding and using the right combination of Data Quality tools is important for maximizing Data Quality and the organization’s overall efficiency.
Finding the most appropriate Data Quality tools can be a challenge. Choosing smart and workflow-driven Data Quality tools, preferably with embedded quality controls, promotes a system of trust which “scales.” The general consensus is that a single, stand-alone Data Quality tool will not provide optimum results.