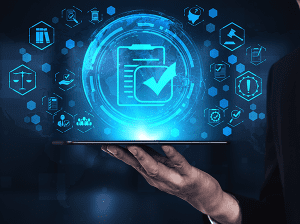
Creating a successful Data Quality program is essential for any organization seeking to use their data for improving efficiency and better decision-making. Data of poor quality can result in decisions that damage the business. Building a successful Data Quality program helps to ensure the data is of the highest quality, making it both useful and profitable. If the data cannot be trusted, the decisions made while using that data can’t be trusted.
The impact of low or poor Data Quality can result in significant damage to a business. An effective Data Quality program helps to ensure that the organization’s data is accurate and useful. Developing a successful program requires having the data steward assess the business’s current level of Data Quality, put functional strategies in place, and develop a system of best practices. Data stewards are necessary for the management and organization of a business’s Data Quality program.
In his article Data Quality is Everyone’s Business — Managing Information Quality, Tom Breur, vice president of advanced analytics at Health Advances made this observation:
“With data stewards in place, and quality control supported by a Data Quality scorecard, we observed an interesting phenomenon. With no additional measures, merely raising attention for the importance of Data Quality, and constant feedback on error rates, the accuracy kept climbing to levels previously deemed impossible.”
A sample of a Data Quality scorecard that can be adapted to suit the organization’s purposes is offered by ABX MEDIA.
Human error plays a major role in distorting data and producing data of poor quality. However, the implementation and use of automated software services can reduce these errors significantly. Whenever and wherever possible, automated services should be implemented. One of the biggest Data Quality concerns in any business is human error.
According to Alexander Wurm, a senior analyst at Nucleus Research,
“You tend to see the greatest risk anywhere there are human touch points. That’s why automating processes like onboarding and offboarding can have value both in improved data security, as well as in gaining new process efficiencies or time savings.”
The Importance of the Data Steward in a Data Quality Program
The data steward is responsible for the data’s quality – the data’s accuracy, consistency, and formatting. The data steward is also responsible for managing Data Governance policies, monitoring compliance, and dealing with data-related challenges.
More and more, business owners and managers are realizing the need for data stewardship, particularly as their business expands. Additional responsibilities of a data steward may include, but are not limited to:
- Data storage
- Ensuring that the new data doesn’t overlap any existing, contradicting data
- Ensuring that the data is error-free
- Looking for possible errors in the data structure
- Approving the consistency of data
By monitoring the data (or keeping track of the software monitoring the data), a data steward can identify and deal with data-related problems, maintain appropriate privacy and security standards, and promote data-driven decision-making.
The workload of data stewards varies, depending on the size of the organization, and its Data Management needs. A small organization, with minimal Data Management needs, might assign and train a current member of the staff to be the part-time data steward. In a larger organization, a few data stewards may be needed to deal with technical data, security data, etc. Additionally, a large, complex organization might decide to add a data steward “manager” to oversee multiple data stewards (and possibly the data pipeline manager).
It is important the data steward has a strong understanding of the business’s overall goals and objectives.
The philosophy of “If it ain’t broke, don’t fix it!” should not be applied to modern data-driven businesses. Modern businesses are in a constant state of evolution, with the goal of beating the competition. As a consequence, data stewards should schedule regular reviews of their practices and tools to ensure Data Quality standards continue to evolve.
Data has value – as long as it is accurate and consistent.
The Key Features of Data Quality
By assessing the data’s quality, by measuring its accuracy, completeness, and consistency (a customer’s address is the same in both billing and sales), the data steward can help to ensure fairly reliable data.
High Data Quality data provides information that is reliable and actionable. Providing good Data Quality requires identifying and correcting errors, removing duplicates (preferably by relying on master data), and properly formatting the data.
Assessing Data Quality often includes establishing a standard of acceptable Data Quality, using data profiling and analysis techniques, and using statistical methods to identify and correct any Data Quality issues. The key features (often called “dimensions”) that should be examined and measured are:
- Completeness: Data should not be missing or have incomplete values. (A completeness assessment can be used to ensure vital information isn’t missing.)
- Uniqueness: Locate and eliminate copies to ensure the information in the organization’s data files is free of duplication.
- Validity: This refers to how useful the data is, and how well the data conforms to the organization’s standards. (Storing useless data is a waste of resources and it can confuse and damage research.)
- Timeliness: Old information that is often no longer true or accurate needs to be removed. Data can be measured using its relevance and freshness. Out-of-date data should be eliminated, so as not to cause confusion.
- Accuracy: This is the precision of data, and how accurately it represents the real-world information.
- Consistency: When data is copied, the information should be consistent and accurate. The need for a single source of accurate in-house data provides a good argument for the use of master data and its best practices. (A consistency assessment ensures there are no contradictions or informational conflicts.)
Using Data Lineage and Data Cataloging to Improve Data Quality
The use of data lineage and data cataloging to Improve Data Quality are fairly recent innovations. Increasingly, organizations are recognizing the importance of using data cataloging and lineage to improve and maintain Data Quality.
Data catalogs can be used to provide a history leading back to the source, and data stewards can use the data lineage to monitor and maintain Data Quality.
The Assessment Process
Assessing the organization’s Data Quality helps in identifying gaps, improving Data Governance, and making decisions that are based on reliable, high-quality data. Generally speaking, performing a “manual” Data Quality assessment requires so much effort the majority of managers would never approve it.
The assessment process includes several steps:
- Reviewing the organization’s overall business objectives is always a good first step before planning and making organizational improvements.
- The second step involves identifying specific areas where Data Quality improvements will promote the business’s success. Consider which key features – accuracy, completeness, validity, consistency, uniqueness, and timeliness – should be improved to have the greatest impact on the business’s processes and decision-making.
- Develop a measurement system. For example, in assessing the uniqueness feature, the number of files having the same title can be located and counted. (I have several “old” resumes, and the current updated one, all with the same title, stored in various places on my laptop. They take up some storage space, but more importantly, they often cause a little confusion before sending the updated one to a potential client. This qualifies as poor Data Quality management.) By examining 20 to 50 different file titles for copies, a statistical estimate of uniqueness can be made. If 50% of the titles have multiple copies, uniqueness should be a concern. If only two of the titles have a single copy, statistically speaking, uniqueness should not be a high priority.
- Assess the data using key features to identify Data Quality issues.
- After examining the data, the data steward can begin the process of eliminating unnecessary or inaccurate data (data cleansing), and establish procedures, based on best practices, that will promote the storage and use of high-quality data.
Best Practices for a Successful Data Quality Program
Historically, developing Data Quality has been treated as a maintenance and repair issue – a process of detecting problems after the data has already been stored in the organization’s databases. However, a Data Quality program can be designed to address data concerns proactively as data moves through the organization.
Some best practices for maintaining Data Quality are listed below:
- Examine and assess the organization’s commonly used external data sources for limitations or formatting issues.
- Maintain a focus on the business strategy.
- Recognize that applying Data Quality is a practice with no completion date.
- Use automation whenever possible to minimize human error and complete work tasks.
- Develop a standardized data processing vocabulary for good communications.
- The data steward should identify and establish responsibilities for other staff in maintaining Data Quality.
- The data steward should educate and update the staff and management.
- Provide updates (weekly reports, then shift to monthly reports).
- Implement a regularly scheduled data cleansing program (software can be used).
- Implement regular Data Quality assessments (perhaps every six months).
Image used under license from Shutterstock