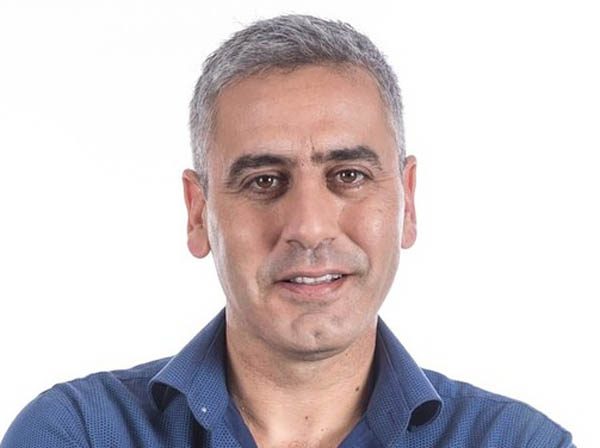
Click to learn more about author Itamar Ben Hamo.
Data scientists are some of the most in-demand professionals on the market. A LinkedIn Workforce Report in 2018 found 151,000 unfilled data scientist jobs across the United States, with “acute” shortages in San Francisco, Los Angeles, and New York City. And the demand for data scientists is only rising. The number of data scientist positions is expected to grow by 15 percent between 2019 and 2029.
With such a scarcity of data scientists, many companies face a critical skills gap that cannot be closed by hiring. The costs for such a gap can be significant. In a data-driven marketplace, companies that lack the personnel to maximize the value of big data operate at a competitive disadvantage.
Due to this shortage, many companies are reimagining how to perform Data Science. Companies are closing the Data Science skills gap, and unlocking the full potential of big data, by leveraging Data Management platforms and citizen data scientists.
There Are Too Few Data Scientists – and They Spend Most of Their Time on Non-Expert Tasks
Say the phrase “data scientist” to someone, and you might conjure an image of women and men concocting complex machine learning algorithms, perhaps while wearing white lab coats. After all, many data scientists hold Ph.D.’s in mathematics, physics, computer science, and other rigorous disciplines. They have often mastered an alphabet soup of programming languages, from R to SQL to Python.
The core value of data scientists is directly tied to this expertise, through the generation of models, algorithms, and business insights. However, any data scientist will tell you that on a day-to-day basis, devoting uninterrupted time to such projects is difficult. Most data scientists spend many hours performing rote tasks that fail to put their advanced skills to good use.
Consider the numbers. Studies have shown that most of a data scientist’s time is spent cleaning data. In fact, gathering and cleaning data accounts for about 41 percent of a data scientist’s time, whereas building and running models accounts for only about 31 percent. That’s the sad truth: Many data scientists spend more time performing grunt work than they do producing insights.
This represents a problem within a problem. Not only are data scientists in short supply, but they are also wasting their precious time performing tasks that do not require advanced skills. But by eliminating this grunt work, and outsourcing other Data Science tasks, data scientists can focus on delivering the valuable insights they were hired for. And that’s where Data Management platforms and citizen data scientists come in.
Data Management Platforms: Free Data Scientists to Focus on Their Core Mission
Data scientists work on a variety of projects with diverse data sets. The raw data is rarely ready for modeling or analytics, hence the statistics about cleaning and preparing data. But before data scientists can even arrive at this stage, the data must be aggregated from various data sources, both internal and external.
Inefficiencies during the data integration stage impact time-to-results for data scientists. If a company manually builds data connectors for data source APIs, then data scientists must delay the completion of critical projects. Sometimes, data scientists might have to manually pull the data using cumbersome, time-consuming methods, further straining their valuable bandwidth.
This is one of the reasons why so many data teams have adopted Data Management platforms. Data Management platforms are equipped with pre-built data connectors, and can often ingest raw data from API sources in a matter of clicks. Some platforms can quickly add bespoke data sources through an API on-demand program or a custom API. For data scientists, these sorts of capabilities remove the friction of data integration, so there’s no waiting for or scrounging for the right data sets.
Once data scientists acquire raw data, they must clean and prepare data for modeling and analysis. One study found that data scientists spend 80 percent of their time on the unskilled task of cleaning data. Cutting this to just 40 percent would allow data scientists to focus a majority of their time generating insights.
In recent years, augmented Data Management platforms have reduced grunt work such as data cleansing. Augmented Data Management performs the key functions of Data Management, including ingesting, storing, organizing, and maintaining data. But ADM also uses machine learning and AI to automatically refine data. Augmented Data Management performs low-level tasks, such as data preparation, eliminating the need for human input. By nixing these manual tasks, augmented Data Management enables data scientists to focus on modeling and analysis, boosting productivity and efficiency.
The rise of cloud data warehouses has also contributed to more efficient data cleansing and preparation. Data Management platforms can now perform SQL-based transformations directly inside of cloud data warehouses. Furthermore, some Data Management platforms can ingest and transform data in the same automated workflow. With this kind of automated data orchestration, data scientists can directly receive the data they need, in the format they need, without having to lift a finger.
As organizations move more toward DataOps, Data Management platforms will deliver data directly to individual stakeholders, including data scientists. This new paradigm will minimize the amount of time data scientists spend on data gathering and preparation, allowing them to focus on coding and building models, rather than on performing inexpert tasks that make poor use of their advanced skill set. Anybody – or better yet, any machine – can execute basic Data Management tasks. But the sophisticated insights must come from data scientists themselves. By freeing data scientists to focus on their core mission, Data Management technologies help close the demand problem at the heart of the skills gap.
Citizen Data Scientists: Unleash the Skills of Your Existing Human Capital
Data Management platforms eliminate gruntwork and streamline data analysis, but closing the Data Science skills gap is not solely a function of technology. Companies are also turning existing workers into “citizen data scientists” to diminish the skills deficit and facilitate Data Science across their organizations.
Gartner defines a citizen data scientist as a “person who creates or generates models that use advanced diagnostic analytics or predictive and prescriptive capabilities, but whose primary job function is outside the field of statistics and analytics.”
By leveraging technology, including Data Management platforms, citizen data scientists produce complex diagnostic analysis and generate models that harness predictive or prescriptive analytics. This enables citizen data scientists to create deeper and more robust analytics than the average business user. In terms of closing the skills gap, citizen data scientists are key to producing advanced insights.
In most companies, data professionals are natural fits for the role of citizen data scientist. Data analysts, BI engineers, ETL architects, and other data experts all have the skill sets to perform Data Science functions. But citizen data scientists can also be found outside of the data team. Each worker brings different technical skills to the table. Those who can adapt to change are often the best candidates for the role.
By using tools such as AutoML and Metadata Management, citizen data scientists can generate advanced analytics for broader initiatives, or for their respective teams. According to McKinsey, only 43 percent of B2B sales teams effectively harness advanced analytics. But a citizen data scientist on the team, such as a sales engineer, could deploy advanced analytics with the right technology.
As the data scientist shortage becomes more pronounced, more companies are turning to citizen data scientists to fill the hole. By one measure, citizen data scientists now produce more advanced analytics than actual data scientists. And this trend will only increase as the skills gap grows wider. Companies must develop the technologies, processes, and educational resources that will unlock the citizen data scientists in their workforces.
But utilizing human capital goes beyond citizen data scientists. Even workers that are not versed in generating analytics can perform basic tasks, such as data formatting, and adhering to data best practices. The Data Science skills gap requires an organization-wide response. Companies that invest in initiatives such as Data Literacy can improve all data operations, including Data Science.
Companies Must Harness Technology and Workforce Skills to Close the Data Science Gap
The economy is predicted to add 11.5 million new Data Science jobs by 2026, but the number of available Data Science workers is not growing fast enough to meet the market need. A skills gap is inevitable; in fact, a skills gap is already here for most companies. In order to remain competitive, companies must develop a way to close the gap.
That’s why some companies are turning to Data Management platforms and citizen data scientists. With these two factors working in tandem, companies can eliminate the rote work that eats up the time of data scientists, and leverage existing workers to develop advanced analytics.
This frees up data scientists to focus on generating the insights they were hired for, and empowers citizen data scientists to produce similar insights. By unchaining the expert skills of data scientists, and the dormant skills of existing workers, companies can close the Data Science skills gap.