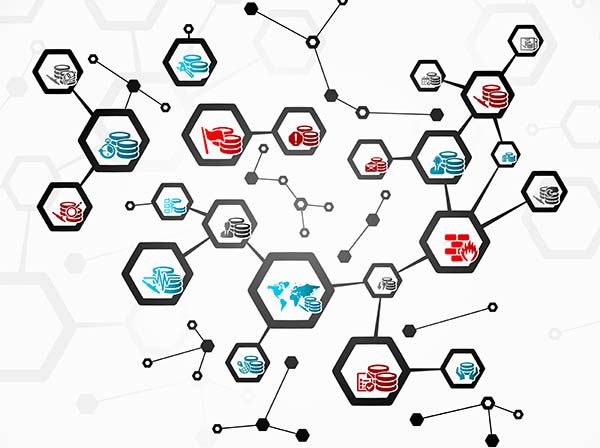
This next year demands quicker data-driven decisions, better automation, more integration, and more data, requiring optimized Data Modeling. Data Modeling refers to documentation describing core business rules and definitions around data. Business and technical shareholders benefit from Data Modeling by seeing complex data concepts in an intuitive, visual way.
Throughout 2020, Data Modeling will need to do more, faster, with less. Economic and political uncertainties will flatten IT spending while newer technologies process information at higher speeds. Add to this situation greater data volumes from a multitude of systems, including cloud computing and the Internet of Things (IoT). Plus, put in a low tolerance for activities that can cause bottle-necks and executives who want bold artificial intelligence results in automating business tasks. This leaves data modelers to solve the highest number of complex business problems, with the most data points in the shortest time frame.
In the meantime, the Data Modeling world has become bigger, extending to retailers and wholesalers. Industries that have Data Modeling on their periphery will put it closer to the front and center to do advanced analytics. According to DATAVERSITY® Trends in Data Management 2019, about 49 percent of companies actively use Data Modeling, and those that have not implemented Data Modeling are feeling the pain of skipping this step. Increasing data volumes and formats and business competition makes starting or restarting Data Modeling harder. To stay competitive, companies will want to optimize Data Modeling.
In 2020, at least, four Data Modeling trends will emerge:
- Just-in-time Data Modeling
- Better automation and machine learning
- More widespread use of Data Modeling
- Focused Data Modeling
Just-in-Time Data Modeling
Data Modeling will have to be conceptualized and created faster and more fluidly to adapt to a rapidly changing world. That means 2020 will see more of:
- Agile Data Modeling: Agile Data Modeling is just-in-time Data Modeling using “a minimally sufficient design” and “the right data model for specific situations.” This philosophy deals well with a mix of unstructured data, relational data, master data, and dimensional data.
- Graph Databases: Graph databases provide easy, quick, visuals of business cases. This database type contains nodes and edges, grouping data sets and their descriptions. Lines connecting these entities describe relationships. Graph databases provide a mechanism for group data evaluation and a comprehensible view of business rules.
- Business Intelligence and Reporting: Data modelers will continue to diagram and document relational databases, as they are heavily used by many businesses, as Donna Burbank states in her webinar Data Modeling Best Practices – Business & Technical Approaches. In these situations, graph databases are not the best tools to conceptualize or detail data. data modelers will continue to use E-R relational, star schema, or data flow diagrams in 2020. These Data Modeling methods will be less comprehensive and chunked based on specific business and technical use cases.
- Cloud-based Computing: Data Modeling will happen more in the cloud. Firms continue to increase spending on cloud-based Data Management because of scalability, speed, and low-cost. Emerging cloud-based quantum computing, a cloud-based invocation of quantum emulators, simulators, or processors, promises to increase computing strength and power within the next few years, resulting in faster interconnects. The low cost and high scalability of the cloud computing will encourage “larger applications that circulate more data through their hardware.” Access to the data models, for anyone, will require an internet connection.
- Wi-Fi 6: Wi-Fi 6 will make Data Modeling more portable in 2020. This soon to be released technology will “deliver up to 40 percent higher data rates” for a single client device and increase network efficiency more than four times. Data modelers will see a higher volume of data from a wider range of systems, including the cloud. Business and technical stakeholders will demand to see data models from anywhere — on the train, plane, and factory floor.
Better Automation and Machine Learning
Data Modeling automation will continue to be disruptive in 2020. Data architects and data scientists, who sometimes employ Data Modeling in their work, will find these tasks mechanized by more than 40 percent. Automation will enable the typical business analyst, with some basic training, to create data models. Firms like Data Robot have provided self-service Data Modeling for those who are less technical. While automation will make data modeling easier, a human will still be needed. Data scientists, with domain knowledge, will need to clarify and reframe any business problems, handle complex data models, and validate automated data models that make sense. Data Modeling automation and humans’ skills will need to work together.
Machine learning technologies, used to model data, will generate mixed results in 2020. Augmented analytics will continue to drive Data Modeling. Knowledge base construction or KBC will find heavier use to train algorithms in 2020. In a review of the literature, Xiaoming Wang, et al. talk about how machine learning built a KBC that can outperform human efforts in paleobiology. These kinds of results will make machine learning look very attractive to create data models.
However, machine learning initiatives will flounder without quality, integrated data sets and targeted questions. Where Data Governance initiatives are at an early stage in many organizations, data sets may not be accurate, leading to algorithms learning from false data.
Likewise, misunderstanding machine learning technology will result in asking for analysis that algorithms cannot do. As these kinds of decisionmakers learn from their mistakes, they will become savvier when viewing computer-generated data models, leading to greater and faster knowledge than given by a human analyst.
Data Modeling Becomes More Widespread
Data model applications will become more plug and play and mainstream in the 2020 marketplace. Already, some retail businesses are on board. Dealer Tire, a distributor of tires and other automotive parts, already has built predictive models to know when customers need tires. “People who buy tires at a dealership are 2.7 times more likely to return” for service. Dealer Tire has seen a 21 percent increase in revenue from marketing to customers needing a replacement, suggesting they buy new tires.
Clint Boulton at CIO cites Gartner research which says:
“By 2020, analytics that can help companies predict outcomes and prescribe courses of action will attract 40 percent of enterprises’ new investment in business intelligence and analytics software.”
Retailers that digitally thrive, account for “40% of the retail market and are generating over 60% of the economic benefits.” Businesses need predictive analysis from Data Models to stay competitive.
Data Modeling will open up to people who work outside of data science, statistics, and analytics. These citizen data scientists can generate prescriptive, predictive, and diagnostic data models due to advances in Data Modeling automation. Gartner predicts by “2020 that citizen data scientists will surpass data scientists.”
With so much information to analyze, a growing number of data scientists, having more control, will lead to more knowledge discovery. Improvements in Data Modeling through machine learning will provide citizen data scientists more self-service Data Modeling. However, expert data scientists, while doing less manual work, will be needed for complex business analysis and data model validation. The more complex Data Modeling will continue to be left to the experts but simpler Data Modeling will be optimized for novices.
Focused Data Modeling
Data Modeling will become more focused in 2020. The combination of deploying data systems to the cloud, machine learning projects in the pipeline, increased data volume from the Internet of Things (IoT), and a demand for real-time data will require less comprehensive one size fits all data models based on specific documents and databases.
Instead, Data Modeling will become hybrids of larger enterprise knowledge and technical details. Thomas Frisendal, owner and database consultant at TF Informatik, states that data models need to address multi-level concerns: business level, solution level (logical level concerns), and implementation concerns. Data models will be customized to the specific purpose and audience that will view and use the data model, as Donna Burbank mentioned in her Data Modeling webinar above.
To focus on Data Modeling, Metadata Management will be a bigger priority in 2020. A recent Gartner article states that the number of “use cases at the core of a business, on its edges and beyond, is exploding.” At the same time, capabilities finding enterprise knowledge has become ever-more complex. Grand View Research estimates that the global enterprise search market is expected to reach 5.02 billion by the start of 2020. Data modelers will need to ask for better enterprise search capabilities to find relevant data.
Data Modeling will become more widespread and self-serve in 2020. Just-in-time Data Modeling and machine learning will optimize Data Modeling, making it quicker and easier for some of the tasks. Complex Data Modeling will continue to need data scientists and drive customizable, scaled data-models. Data Modeling will have to be more focused throughout 2020, so access to good metadata will be needed.
Image used under license from Shutterstock.com