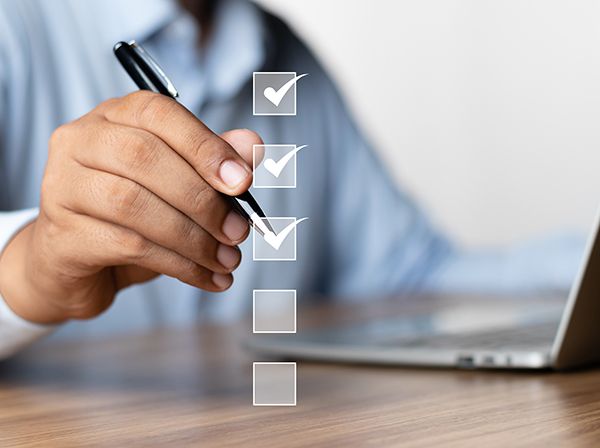
The goal of a Data Quality assessment is not only to identify incorrect data but to also estimate the damage done to the business’s processes and to implement corrective actions. Many large businesses struggle to maintain the quality of their data.
It is important to remember data is not always in storage and static but gets used periodically. After being created, data becomes downloaded, adjusted, reformatted, exchanged, and even destroyed.
If done incorrectly, each action comes with the threat of having a negative impact on the data’s quality. In turn, poor Data Quality may result in bottlenecks and often negatively affects the decisions an organization makes. Without the right measurement system in place, low-quality data might never be noticed or corrected.
Many businesses don’t know they have Data Quality issues. Assessing the data’s quality is a small but very important part of maximizing a business’s efficiency. Issues with the data’s quality may be first noticed by the organization’s business operations or by its IT department. The initial steps in carrying out an assessment of the data’s quality can be considered an “awareness phase.”
A Data Quality assessment supports developing a data strategy, and a well-organized Data Strategy will align the data, supporting the business’s goals, values, and targets.
Data Profiling vs. Data Quality Assessments
Data profiling is often considered a preliminary step to performing a Data Quality assessment, while some people believe the two should be done simultaneously. Data profiling deals with understanding the data’s structure, as well as its content and interrelationships. A Data Quality assessment, on the other hand, evaluates and identifies an organization’s data problems, and the consequences of those problems.
Useful Data Quality Assessment Metrics
Data Quality assessment metrics measure, among other things, how relevant, reliable, accurate, and consistent an organization’s data is. Depending on a business’s type of industry and goals, specific metrics may be needed to determine if the organization’s data meets its quality requirements. Measuring the quality of the data, understanding how data metrics are used, and how the tools and best practices function is a necessary step in becoming a data-driven organization.
Basic Data Quality metrics include:
Relevance: The data might be of high quality, but useless in terms of helping the organization in accomplishing its goals. For example, a business focused on selling customized boots would be interested in useful shipping data but would have no interest in a list of people seeking products for repairing boots. Storing data with the vague hope it will be relevant later is a common mistake. Metaplane offers software for measuring relevance.
Accuracy: Often considered the most important measurement for Data Quality, accuracy should be measured through documentation of the source or some other independent confirmation technique. The accuracy metric also includes status changes to the data as it happens in real time.
Timeliness: Outdated data ranges from useless to potentially damaging. For example, client contact data that is never updated will harm marketing campaigns and advertising. There is also the potential for shipping products to the old, no-longer-correct address. Good business requires all data to be updated for smooth efficient business processes.
Completeness: Data completeness is normally determined by deciding whether each of the data entries is a “complete” data entry. Incomplete data often fails to provide useful business insights. In many situations, the process of assessing completeness is a subjective measurement made by a data professional and not Data Quality software.
Integrity: Data integrity describes the overall accuracy, consistency, and completeness of the data throughout its entire life cycle. Data integrity is also associated with the safety of the data in terms of regulatory compliance regarding personal privacy and security.
Consistency: Different versions of the same data can make doing business confusing. Data and information must be consistent across all the business’s systems to avoid confusion. Fortunately, software is available, so each version of the data does not have to be compared manually. (Master data and its management is an option for centralizing data used repetitively and avoiding multiple versions.)
Prepping for the Assessment
A Data Quality assessment will move along more efficiently and provide better results if a list of concerns and goals is created before the assessment. When creating this list, be aware of the organization’s long-term goals, while listing short-term goals. For example, the long-term goal of making the business more efficient can be broken down into smaller goals, such as fixing the system so the right people get the right bills, and that all the clients’ addresses are correct, etc.
This list can also be presented to a board of directors as a rationale for initiating and paying for Data Quality assessment software or hiring a contractor to perform the assessment. The basic steps for creating the list are presented below.
- Start by making a list of Data Quality problems that have occurred over the last year.
- Spend a week or two observing the flow of data and determine what looks questionable, and why.
- Share your observations with other managers and staff, get feedback, and adjust the results using the feedback.
- Examine the Data Quality problems list and determine which are the highest priorities, based on how they are impacting revenue.
- Rewrite the list, so the priorities are listed first. (This list can be made available to the board of directors and the Data Quality assessment contractor after the scope has been established.)
- Establish the scope – what data will be looked at during the assessment?
- Determine who is using the data, and examine their data usage behavior before and after the assessment to determine if they need to make changes.
Data Quality Assessment Platforms
Performing a Data Quality assessment manually requires so much effort that most managers would never approve it. Fortunately, there are Data Quality platforms and solutions available. Some take a holistic approach, while others focus on certain platforms or tools. Data Quality assessment platforms can help organizations in dealing with the growing data challenges they face.
As the use of the cloud and edge computing services expands, organizations can use Data Quality assessment platforms to analyze, manage, and cleanse data taken from different sources such as email, social media, and the Internet of Things. Some assessment platforms (which include dashboards) are discussed below.
The Erwin Data Intelligence Platform uses AI- and ML-enabled discovery tools to detect data patterns and will create business rules for the Data Quality assessment. The Erwin Data Intelligence Platform automates the Data Quality assessment, provides ongoing data observability, and includes detailed dashboards.
Acceldata’s Enterprise Data Observability Platform integrates well with diverse technologies and works well with public, hybrid, and multi-cloud environments. It provides a highly effective Data Quality dashboard and uses machine learning automation algorithms to help maximize your data’s efficiency. Acceldata’s platform will detect and correct problems at the beginning of the data pipeline, isolating them before they affect downstream analytics.
The IBM Infosphere Information Server for Data Quality Platform provides a broad range of Data Quality tools to help analyze and monitor the data’s quality continuously. The IBM platform will cleanse and standardize data while analyzing and monitoring Data Quality to reduce incorrect or inconsistent data.
Data Ladder’s DataMatch Enterprise has a flexible architecture that provides a variety of tools that can clean and standardize data. It can be integrated into most systems and is easy to use. DataMatch Enterprise is a self-service Data Quality tool that can identify basic anomalies. It measures accuracy, completeness, timeliness, etc. It also performs detailed data cleansing, matching, and merging.
Intellectyx acts as a contractor for a variety of data services, including providing Data Quality assessments and solutions. Their process includes:
- Identifying the business needs
- Defining Data Quality metrics
- Assessing current Data Quality
- Developing a plan for improvement
OpenRefine is not a Data Quality assessment platform, but it is a free, powerful, open-source tool designed to work with messy data. The tool will clean the data, transforming it into the appropriate format. The data is cleaned on your computer system, rather than a data laundering cloud.
The Assessment Report
Data Quality assessment reports are normally designed to describe the results of the assessment, as well as observations and recommendations. The report includes any anomalies that have had a critical impact on the organization, as well as solutions for identifying and eliminating those anomalies.
The report should include:
- Executive summary: An introduction combined with a brief description of the report
- Key findings: Problems with the flow of data and how they impact the business
- The process used: Describe software and the process. (If a contractor has been used, the report is their responsibility)
- Scores and overall ratings (per issue)
- Recommendations (per issue)
- Open issues: Any unresolved problems
- A conclusion: The expected results on the business when the changes are made, and observations or advice regarding the unresolved issues
Image used under license from Shutterstock.com