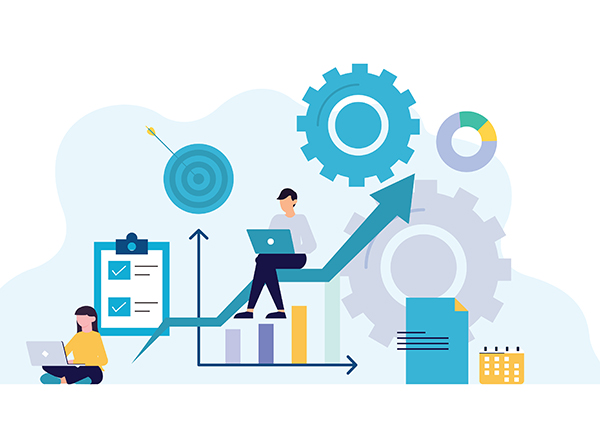
Using Data Quality best practices to gather accurate data is essential for gaining useful results from research and providing visiting customers with a good experience. Data has become a key part of most business transactions and operations. The quality of data that is gathered, stored, and used are major factors in the success of a business. High-quality data represents the real world.
One of the largest contributors to data “inaccuracy,” or poor-quality data, is simple human error.
Other contributing factors for data inaccuracy include a lack of Data Management and Data Governance programs, data acquired from social media, a lack of communication that sometimes takes place between departments, and an underdeveloped Data Strategy. Gartner estimates bad data costs businesses an average of $12.9 million every year.
Changing people’s behavior in the workplace can be difficult. Some will embrace changes, while others will resist. As a rule, most people agree having good Data Quality is good for business. However, there may be disagreements about how to achieve it, and who should be responsible for what. Internal politics and resistance to change can make it difficult to develop policies supporting strong Data Quality.
Resolving poor-quality data issues requires the complete support of all members of management.
The Competitive Edge
In today’s market, it is assumed the competition is constantly improving the quality, efficiency, and profitability of their services, and probably stealing your customers. The current business philosophy promotes the fear that “business may be good now, but how long will that last?” It’s not a completely outrageous fear, especially if the company in question has poor Data Quality.
If customers are not satisfied with a company because of its poor Data Quality, those customers will attempt to find one that is easier to work with. Following a system of Data Quality best practices reduces the problems caused by inaccurate data and minimizes concerns about the customer experience and efficient research.
Using a system of Data Quality best practices increases efficiency, resulting in greater profits.
Symptoms of Poor Data Quality
Some businesses send out two or three email advertisements to the same person, but with slight differences (such as different spellings of their name or assigning different genders to each email), annoying the potential customer. Others fail to recognize the customer has done business with them before, and demand information be uploaded, once again, irritating the customer. There are a variety of ways poor Data Quality can negatively impact an organization.
If more than a few of the following concerns are recognized as taking place within the organization, then it is almost a certainty there are Data Quality problems. Key symptoms of poor-quality data are:
- Information that should be found easily is missing or hard to locate.
- A slow reaction time to new marketing opportunities (resulting in missed opportunities and reduced profits).
- Some of the staff create their own spreadsheets to keep “safe” records.
- Large amounts of time and effort are spent collating weekly and monthly statistics.
- Customers make complaints about staff for not knowing about previous transactions.
- Service failures take place that should have been easily predictable, as well as preventable, if someone had reviewed the data.
- Despite having multiple data lakes and data warehouses, it is impossible to get high-quality data.
- Data researchers use 80% of their time in “preparing” the data for analysis.
- Project budgets are spent on data migration activities, instead of the actual project.
- Manual corrections of data become an obstacle in implementing cost savings programs. (Automation typically does not work well with incomplete, inconsistent data.)
- Compliance requirements are not met (GDPR, health and safety requirements, financial restrictions and requirements).
Starting with Master Data Management and Data Governance
If your organization does not currently have a Master Data Management (MDM) platform or a Data Governance (DG) program, be prepared to make some software investments, and to make some significant changes to the work culture and how data is processed. Improving Data Quality requires changing people’s behavior, changing processes, and adding software. (DG and MDM software should be completely compatible.)
The Master Data Management Platform: This type of software typically includes and coordinates applications such as customer data integration, Data Quality management, product information management, data profiling, etc. It also coordinates with the Data Governance program. Master Data Management transforms raw data into useful information and data that can be used with other business processes.
Implementing an MDM platform includes a focus on the organization’s business strategy and needs. The following should be considered when planning for the MDM implementation:
- Improving the customer experience
- Gaining a complete view of customer profiles
- Centralizing customer information
- Improving Data Quality
- Reducing customer communication costs
- Improving business decisions with high-quality data
Identifying and including the business’s goals are important when developing an MDM strategy.
The Data Governance Program: Creating a Data Governance program includes changing the workplace culture, establishing and assigning responsibilities to key individuals, and installing and using appropriate Data Governance software. While a number of Data Governance roles can be created for large businesses, small and medium-sized organizations should include at least the following three positions of responsibility:
- The Data Governance Committee: This group often comprises the organization’s business managers, IT leaders, and stakeholders. After this group is formed, they are responsible for creating the organization’s policies and standards, which are used to develop a Data Strategy and Data Governance framework. They are also responsible for resolving problems brought to them by the chief data officer.
- The Chief Data Officer: This role is often taken by a senior employee. They act as a mid-level manager between the data steward and the Data Governance committee. This person is responsible for coordinating Data Governance projects and programs. The chief data officer oversees the Data Governance program, and also takes responsibility for resolving any data disputes within the organization.
- The Data Steward: The data steward acts as a cross between an educator and the data police. They must have a good understanding of both business and information technology. This is often added to an existing manager’s responsibilities. Depending on the goals and the size of the business, data stewards can be extremely useful. (Some organizations have multiple data stewards.)
Integrating Data Quality One Small Step at a Time
Changes in the workplace vocabulary are a good first step in altering the culture. Requiring staff to learn a few words and phrases about processing data every few days, and use them, promotes an understanding of the changes about to take place. After that, a few “small” projects (not the big ones at first, but some small successful ones) will demonstrate that improving Data Quality can help in achieving business goals quickly and efficiently. Starting small allows for the development of a repeatable process, and staff can be trained incrementally with small projects.
Best Practices for Improving Data Quality
Changing the workplace culture requires the participation of staff throughout the organization – and to be done successfully it needs the commitment of upper-level management and executives. Some important Data Quality best practices are:
- Use automation whenever and wherever possible. It helps to eliminate human error and completes tasks much more quickly than humans.
- Develop standardized data processing definitions for good communications.
- Identify the organization’s data domains.
- Maintain a focus on the business strategy. Both the MDM platform and the DG framework must reflect the goals of the business.
- Develop a map of the business’s architecture, infrastructure, and tools.
- Identify and establish the roles and responsibilities of the staff and management.
- When organizing projects, set clear, specific goals.
- Educate and update the organization’s stakeholders. This may involve meetings and written updates.
- Identify critical areas needing improvement.
- Communicate frequently, especially at the beginning of the changes. (Weekly and monthly reports are a good idea.)
- Remember that the use of the best practices for Data Quality is a practice, not a project with a completion date.
Image used under license from Shutterstock.com