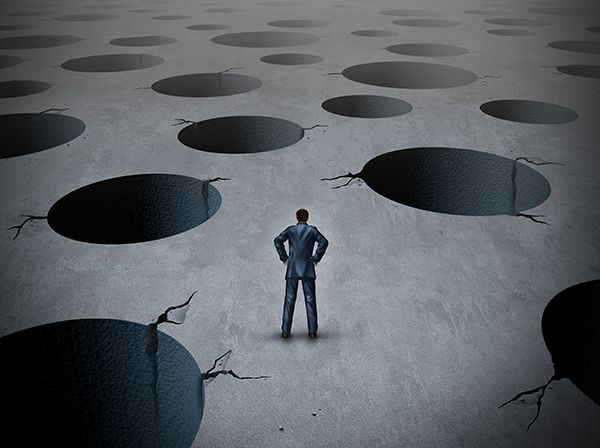
In the digital business world, organizations can’t take advantage of their data assets without a proper Data Strategy in place. The Data Strategy is the plan that enables an enterprise to meet its business goals through its data. Avoiding common Data Strategy pitfalls and creating a successful strategy empowers an organization to realize its mission and vision through the use of data. The absence of a Data Strategy can lead to the following challenges for an organization:
- Siloed data with multiple versions of “truth”
- Low technology awareness
- Manual data preparation and integration methods
- Low data quality
- Poorly defined and managed metrics and KPIs
- Lack of data-driven decisions
- Absence of predictive or prescriptive analytics
- Overdependence on IT department
Data Strategy: A Quick Definition
Over the years, data has transitioned from being a process byproduct to becoming a critical asset that guides decision-making. A Data Strategy offers a blueprint or plan for storing, managing, and governing data throughout the organization. The Data Strategy guides all enterprise data activities like data quality management, data security, data analytics, database management, data governance, and so on. This plan establishes goals and standards for consistent and effective use of data across data projects.
Data Strategy is especially useful when automation or semi-automation is used for data-driven processes. The core idea behind a Data Strategy is making efficient use of all data resources across an organization, no matter where they are located.
A Data Strategy ensures that data is indeed used and managed as a corporate asset generating revenue. The ideal Data Strategy should be accompanied by a roadmap that guides the implementation of the strategy.
The Data Strategy also provides a framework for data engineers to make architecture decisions for achieving business goals. It offers guidance on how analytics can be applied to derive critical insights, with data visualization being a critical element.
Data Strategy Goals: Critical Business Objectives
In the data world, options for data tools are nearly as abundant as data itself, so it becomes difficult for a business to decide on a solution that is just right for meeting its business goals.
The Data Strategy sets forth a master plan for systematic achievements of the following goals:
- Elimination of all unwanted data and preservation of high-quality, accurate data that a business will most likely need
- Setting standards for Data Quality that can be followed by all business units
- Starting effective communication between business units to streamline data sharing within an organization
- Saving data in a uniform, easy-to-use format for easy access, use, and sharing across the business
- Applying standards to newly collected data to maintain data integrity
- Establishing a single source of truth for data, eliminating data silos, and connecting data across consumers, products, and suppliers
- Consolidating all organizational data into a system that is accessible and usable by everyone in your business
Data Strategy Pitfalls (and How to Avoid Them)
While establishing goals related to each component of an enterprise Data Strategy, including data capture, storage, sharing, and so on, the strategy team can often come across many pitfalls or disadvantages. Below are a few of the common Data Strategy pitfalls and their probable solutions.
- Lack of data culture: Data hidden within silos with little communication between business units leads to a lack of data culture. Data Literacy and enterprise-wide data training is required to allow business staff to read, analyze, and discuss data. Data culture is the starting point for developing an effective Data Strategy.
- The Data Strategy is too focused on data and not on the business side of things: When businesses focus too much on just data, the Data Strategy may just end up serving the needs of analytics without any focus on business needs. An ideal Data Strategy enlists human capabilities and provides opportunities for training staff to carry out the strategy to meet business goals. This approach will work better if citizen data scientists are included in strategy teams to bridge the gap between the data scientist and the business analyst.
- Investing in data technology before democratizing data: In many cases, Data Strategy initiatives focus on quick investment in technology without first addressing data access issues. If data access is not considered first, costly technology investments will go to waste. In such a scenario, technology is being forced on a business without considering the business needs first. The better approach is to align overall business needs with data needs, and then adapt technology that best suits the business goals.
- The data models for unstructured data are not scalable: Many times, short-sighted data models provide limited solutions. To make huge amounts of unstructured data work in any circumstance, it is better to locate an experienced technology partner because these solution providers have extensive exposure to wide use cases and sustainable products.
- Looking for a single solution for Data Governance: Businesses often mistakenly believe that having a chief data officer (CDO) and a Data Management function ensures well-tuned Data Governance, but that rarely happens. Actual business statistics indicate that the best DG practices emerge from “decentralization” – with one CDO managing one business unit.
- Decentralized Data Management can lead to duplicated efforts: Although decentralization helps prevent data silos, it can lead to duplicate systems and efforts. A decentralized approach may work for a data offensive strategy.
- A metadata strategy is implemented in a hurry: Creating a metadata strategy in a hurry will lead to compliance gaps, governance issues, and inconsistent data usage. Instead, leadership should carefully plan and implement a metadata strategy to work within a Data Strategy framework.
- Lack of staff training: This pitfall can leave the greatest impact on the implementation phase of a Data Strategy. Organizations are often in a hurry to architect and design a Data Strategy without first training the staff to properly carry on the strategy. This problem can end up leaving the organization with the same old strategy that it had years ago.
Conclusion
Many organizations are guilty of developing data strategies that just collect dust till the next Data Strategy document surfaces some years later by a new expert. But executing an effective enterprise-wide strategy requires careful consideration: thinking in the long term, identifying needs, and creating business goals for now and in the future.
Image used under license from Shutterstock.com