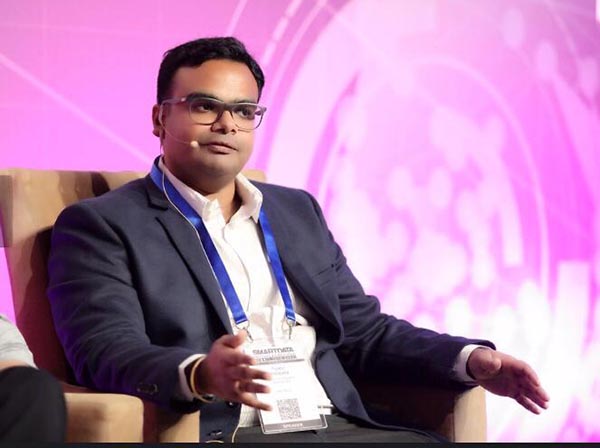
The importance of data has increased multifold as we step into 2022, with an emphasis on active Data Management and Data Governance. Furthermore, thanks to the introduction of new technology and tools, we are now able to automate labor-intensive data and privacy operations. Below are five Data Governance trends organizations can adopt based on digital transformation, data privacy laws, and monetizing data.
1. Data Quality extended into customer journeys and insights delivery
In 2022, the importance of data for monetizable value is being well recognized while the demand for qualitative data is increasing. However, many companies are still living with data silos across their functions, which makes them more product-agnostic rather than domain-centric. As a result, it is difficult to leverage critical data across divisions to generate meaningful insights like marketing and personalizing experiences.
Companies are also realizing the shift in Data Quality priorities towards operations and better servicing customers. But, this can happen when data is curated accurately; so, data acquisition management is a key priority from 2021 that will continue. However, data undergoes transformation along its journey by people and processes along with systems, and lack of consistency can impact the servicing of customers.
Harmonizing Data Quality rules across products and channels will bring consistency in sourcing correct data and leveraging it for operational excellence. A Data Quality rules management tool is an imperative capability that companies have to acquire. Such tools can come with profiling, workflow management capabilities that can be used on batch and real-time data. However, harmonizing generic Data Quality rules can be performed over master data and reference data management solutions while specialized context-based rules will still require access to a Data Quality tool and skills.
Moreover, for many companies, the focus in 2022 will be on recovering bad data and resolving data entry as well as IT issues in application curating data from customers. This is also being influenced by data protection policies around the world that emphasize maintaining accurate data of data subjects for integrity.
Gartner predicts that companies will spend $4.5 trillion this year on digital transformation, a 5.5% increase over 2021. As the focus shifts towards enabling digital journeys and servicing people better within short servicing times, the underlying data needs to be qualitative. Aspects including real-time quality analysis, auto-correction for data recovery, and intelligent automation for standardization of data, for integration, and for delivery using ELT into warehouses or lakes will be a priority. Web services and API integration for real-time quality management while delivering data to consuming applications will be a crucial ask in Data Quality tools.
Analytics and Data Science personnel spend 50% of their efforts on data preparation. Analyzing the quality of data and making it usable for Data Science modeling is a significant part of data preparation. Accurate data is a crucial need to get deliverable insights from artificial intelligence models. How accurate is the data in the real world to give a usable outcome? This is a critical dimension of Data Quality that can be prioritized.
Further, the predictive efficiency of AI models is defined by diversity, scale, and quality of input data. Coverage and availability of data will be key dimensions that can ensure the quality of insights generated by Data Science personnel.
2. Domain-focused data cataloging
Centralized data platforms make it more complex to cascade active management of data and benefit delivery to the business and data owners. As legacy data platforms were based on the availability of special skill-sets, and engineering of data products based on just-in-time requirements, this resulted in non-agility in managing data associated with specific domains like customer servicing or marketing. As new data products evolve, it becomes imperative that coverage of data associated with certain domains be managed at a pace. This would serve new product development, new models and reports, and integrated views with agility.
As a fundamental principle, logically grouping of data into domains and datasets makes it easy to define and actively govern data. Cataloging of data using a strong Metadata Management operating model that includes the business data stewards will be a priority in 2022. Acquiring a data catalog can only support a means of recording metadata and definitions while the right enablement of data stewards, owners of data, and platforms will be required to drive cataloging and observability with these tools. It is possible to use an overarching framework like BIAN for banking, which can assist in logically classifying data.
3. Data fabric and data democratization
There is a growing challenge to better govern data as it increases in variety and volume, and there is an estimate that 7.5 septillion gigabytes of data is generated every single day. Moreover, in organizations, silos are getting created through multiple data lakes or data warehouses without the right guidelines, which will eventually be a challenge in managing this data growth. To achieve nimbleness, we can simplify the data landscape by using a semantic fabric, popularly called data fabric, based on a strong Metadata Management operating model. This can further make data interoperable between divisions and functions while working to a competitive advantage. Data fabric simplifies Data Management, across cloud and on-premise data sources, even though data is managed as domains.
In addition, data democratization can be a strong enabler for managing data across domains with ease and making data available as well as interoperable. Allowing business users to source and consume relevant data for their instantaneous reporting or generation of insights can reduce significant turnaround time in acquiring or sourcing data traditionally. Another advantage of data democratization is having data consumers appraised on new data acquired, along with changes to data.
4. Data privacy and master views of customer consent preferences
In 2022, new data privacy laws will continue to be passed, such as the Personal Data Protection Bill (PDPB) that is expected in India, along with further guidance from industry bodies like the European Data Protection Board on transfer and concepts of de-identification and anonymization. Meanwhile, many organizations have started a data protection program by publishing a privacy policy to customers. Issuing a privacy policy in an easy-to-understand medium to customers is a lucid representation of the privacy principles within the organization to customers. This provides assurance to customers on how personal data is being processed and safeguarded as an asset. However, before publishing a privacy policy, a sensible practice is to understand the purposes and processing activities associated with personal data across business domains through a privacy impact assessment.
Those organizations that start a data protection function will focus on the acquisition of required capabilities, such as identifying data to classify for privacy. They will also focus on defining and encrypting privacy domains as priority domains. Data offices can start leveraging graph to engineer preferences for processing customers’ personal data for purposes like marketing or analytics. While Master Data Management solutions can extend consent and customer preference frameworks, graph databases can naturally answer questions like, “Through which channel are most consents provided by customers?” or “Which customers and their relationships have consented to marketing?” Further, single views of preferences across products, customers, and their households across preferred channels can be easy to analyze.
A data discovery solution or catalog will help classify data across systems, while privacy engineering can help scrub data through a control-based approach based on customer preferences for purposes like personalization. Privacy automation is an important enabler that can assist curation of privacy risks through design while tracking controls with data owners to a closure with a workflow. This will ease communication management along with confusion on ownership of privacy risks.
5. Value realization from Data Management and Data Governance
A common roadblock for a data office is having these divisions own the metrics that monitor the value of governance activities. While there are common enterprise benefits like reduced operational costs and risk, there are benefits that weigh in directly with the value chains of the divisions like client service effectiveness.
1. Every governance enabler should have a metric associated with measurement, such as person hours spent on Metadata Management or number of business terms included in the glossary.
2. The data stewards then, within each business division, can curate information on operational and data value chains.
3. The data stewards along with divisions discover the success factors and metrics used to measure the commercial success of the division, like time to service, customer service effectiveness, cross sell ratio, and many more.
4. Then, it’s recommended to establish a trace between governance enablers and the division’s value chain.
5. The business owner can endorse these metrics and have them monitored at regular intervals, like the increase in integrity of data.
The stated approach creates awareness in the company where you would want governance to seep in. The framework, as I said earlier, should clearly outlay the traceability between Data Governance enablers, technology, and process impact, then followed by business impact and value.