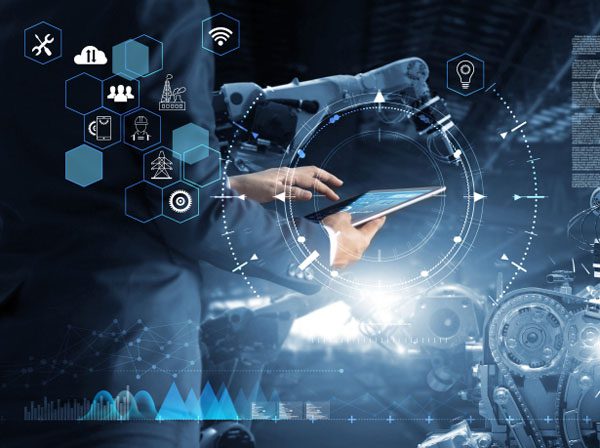
Hyperautomation describes a mixture of advanced technologies – robotics, artificial intelligence, machine learning – currently being used to make automated processes drastically more efficient and to augment humans. It encompasses a range of tools which can be automated, especially the more sophisticated aspects of automation, including analysis, discovery, design, measuring, monitoring, and reassessing.
To function correctly, the tools used must be designed to work and communicate with each other. Ideally, hyperautomation is used to combine technologically advanced tools and create new ways to work.
The design of a hyperautomation tool should prioritize and target the processes needing automation based on strategic goals. For instance, a goal may be to increase production of a certain item or to improve quality control — or both. With hyperautomation, low-value tasks can be performed using a mixture of machine learning and automation tools. With the right design, production can take place automatically, running efficiently with minimal human intervention. Humans working with hyperautomation can create a flexible, agile workplace that uses data to gain insights for quick and efficient decision-making.
While hyperautomation may sound easy, its execution can be difficult and complicated. Hyperautomation requires intelligent planning. There are a variety of technologies involved, and they must be compatible and able to communicate with one another. Choosing easy-to-use tools that communicate well with other systems – and staff – is important. Research software tools that are scalable, and offer “plug and play” solutions can be quite useful. Tools that can accept data taken from different sources and use APIs to communicate with the existing software are also helpful. To minimize confusion, it is important to understand the term “AI” can be a reference to subdivisions of artificial intelligence, such as machine learning, image recognition, natural language processing, voice to text, et cetera, et cetera.
Machine Learning and RPA
One aspect of hyperautomation is referred to as robotic process automation (RPA). Robotics started becoming popular in the 1960s, especially within the manufacturing industry. Hyperautomation merges intelligent business management software with artificial intelligence to create a highly competitive, cost-efficient manufacturing process. A robot is used to perform low-level, repetitive tasks by following rule-based processes. The robot can be programmed to build products by following a series of repetitive steps.
Machine learning is a subdivision of AI being merged with hyperautomation. Machine learning refers to a system that can identify patterns and learn from them. It generally does not require human intervention, using pattern recognition to decide its next action. The system’s algorithm is educated first with training data, which in turn creates a model for learning more about production.
Hyperautomation uses machine learning to follow certain procedures automatically, saving humans time and money while reducing errors. Companies such as FedEx and Amazon have thoroughly embraced automation. They have used hyperautomation to improve the customer experience and to perform management-related tasks.
Other AI Technologies Used in Hyperautomation
Automation can be described as using robotic arms to perform tasks more quickly and with fewer errors. By comparison, hyperautomation can be described as using a robotic brain to perform tasks in smarter ways. This added layer of intelligence often includes various kinds of AI technologies. When these technologies are combined with automation software, the potential for greater flexibility improves dramatically.
Finding technologies and tools that work well with a system is crucial to the success of hyperautomation. Unfortunately, many automation platforms require their users to read and write code. Choosing tools and platforms that are more user-friendly can give a business a significant advantage. A list of some AI technologies used in hyperautomation are as follows:
- Computer Vision: This can be used to analyze visual information, ranging from faces for social media to the chemical composition of objects.
- Speech Recognition: This subdivision of AI can be used communicate in real-time and can gauge various parameters, such as accent, language, and tone.
- Natural Language Processing: NLP for short, this technology generally describes software that uses human language to deal with communications between computers and humans.
- Bioinformatics: This uses information technology to develop methods and software for understanding biological issues.
- Analytics: When combined with RPA, it can become a catalyst for intelligence. Analytics can find patterns in both structured and unstructured content (chat records, voice files, emails, images, and video). It can also be used to uncover fraud.
- Case Management: Appian offers software that maps the interactions between people, data, processes, and content. It uses a graphical design format to associate relationships and provides visual images of the relationships.
- Optical Character Recognition: This converts images to readable text for the robot/AI.
- Digital Twins: A digital model of an organization’s physical assets and equipment. It can be used for predictive purposes and proof-of-concept models.
Use Cases
STL, a data networks innovator, faced the challenge of dealing with growth up to ten times larger than its original design capabilities. As their clients demanded more data at higher speeds, STL chose to scale up exponentially and incorporate an Agile philosophy to deal with their growth issues. The goal was to increase output and efficiency without increasing the workforce. Faster, error-free processes became an essential part of scaling up.
UiPath provided an RPA solution that included an automated invoicing process and helped STL bring about the changes in its business transformation.
MAS Holdings is the leading textile and apparel manufacturer in South Asia, with over 99,000 employees. After a comprehensive research study, they chose to embrace robotic process automation. MAS Holdings manufacturing process required significant amounts of manual intervention. They were able to use UiPath “bots” to make manufacturing corrections and cut their labor costs by 13,000-14,000 days of labor.
Jayantha Peiris, the CIO of MAS Holdings, stated:
“We have a huge front-end process and key processes across customer care, procurement, and finance. The growth that we envisaged required us to achieve augmented business outcomes around productivity, efficiency, and cost savings – outcomes that would be impossible without automating these processes. We knew that RPA will have to form the base of our digital transformation agenda for it to be truly impactful.”
Benefits of Hyperautomation
Hyperautomation offers a way to make organizations more efficient. Gartner predicts that by 2024, organizations will lower their operational costs by up to 30% with a combination of hyperautomation technologies and redesigned operational processes. Hyperautomation can help in optimizing business growth by:
- Minimizing the risk of human error with machines calibrated for precision
- Increasing productivity and higher output
- Using analytics from process mining and discovery tools to measure, track, and improve business outcomes through automation. (Process mining is basically an analysis ofapplication event logs. Event log analyzers are used to provide an analysis. Events contain information regarding what was done, who did it, who it was done for, when, where, etc.)
- Through discovery tools, providing an understanding of how teams work, what should be automated, and who should be augmented
- Helping people and robots work together on basic processes and complex, long-running business projects
- Ideally, initiating a nonstop optimization cycle. The platforms offer powerful analytical tools that allow performance to be tracked, identify areas needing improvement, and measure automated process improvements
Planning for Hyperautomation
A thorough internal assessment of processes and infrastructure is necessary. An assessment of the investment return should also be made. Staff should be trained to use the new automated services. Hyperautomation requires collaboration with humans. Humans are vital decision-makers, and quite useful at interpreting data and applying logic.
Hyperautomation requires strategic planning and architecture. It is important to consider the organization’s short and long-term goals. Consider whether or not a platform is truly designed for hyperautomation and if it will support the components and integrations needed to provide a continuous flow of production.
Image used under license from Shutterstock.com