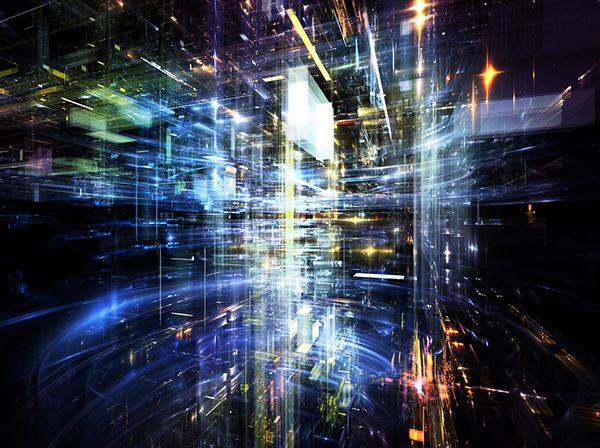
In the evolving world of business, the emergence of new technologies is aiding organizations to make agile and accurate decisions. Real-time analytics – which involves collecting and analyzing data as it happens – is one such technology that is changing the business landscape.
With superfast identification of trends and patterns, businesses can leverage real-time analytics for early detection of business issues. In 2023, real-time analytics will become even more sophisticated thanks to advancements in artificial intelligence (AI) and machine learning (ML). These technologies will allow companies to not only analyze data in real-time but also predict future trends and outcomes. The benefits are clear: faster decision-making, improved operational efficiency, reduced risk, enhanced customer experience, and increased profitability.
With cheaper hardware, economical hardware architectures, affordable data storage platforms, and hosted “data centers” being available to businesses at every corner of the globe, the modern businesses have no excuse for delaying real-time analytics platforms for faster, better, and more accessible data-driven solutions.
Machine Learning and Predictive Analytics
In 2023, machine learning and predictive analytics are at the core of real-time reporting. Machine learning algorithms can process large amounts of data quickly and accurately, making it possible for organizations to gain insights into their operations in real time.
Predictive analytics, on the other hand, allows businesses to forecast future trends based on historical data. By combining these two technologies, organizations can gain a comprehensive understanding of their operations, allowing them to identify potential issues before they occur and take preemptive measures. Furthermore, machine learning algorithms can learn from past patterns and behaviors within an organization’s dataset to predict future outcomes with greater accuracy.
Overall, machine learning and predictive analytics will continue to play an increasingly important role in real-time reporting over the next few years. As organizations seek new ways to leverage data for competitive advantage, these technologies will undoubtedly become even more critical for success.
Real-Time Decision-Making: Empowering Businesses with Actionable Insights
Real-time analytics is revolutionizing the way businesses operate in today’s world. With the emergence of advanced technologies like AI, machine learning, and IoT, organizations can now obtain real-time data insights that provide them with actionable insights to make informed business decisions.
Businesses rely more on real-time analytics than ever before. This trend is driven by the need for speed and agility in an ever-changing business landscape. Moreover, real-time analytics will enable businesses to optimize their operations by predicting potential issues before they occur. This predictive capability will help companies save time and resources while improving overall performance.
Personalization and Customer Engagement: Leveraging Real-Time Data for Competitive Advantage
Real-time analytics continues to play a significant role in personalization and customer engagement strategies. Companies that can leverage real-time data to deliver personalized experiences have a significant competitive advantage over their competitors.
With the increasing use of artificial intelligence and machine learning algorithms in real-time analytics, companies are able to deliver highly targeted and personalized experiences at scale. These technologies enable businesses to analyze vast amounts of data quickly and accurately, allowing them to personalize their offerings in real-time based on individual customer behavior.
Overall, the ability to leverage real-time data for personalization and customer engagement is increasingly important for companies looking to stay competitive. Businesses that invest in these capabilities now will be well-positioned for success as the importance of personalized experiences continues to grow.
Edge Computing: Enabling Predictive Maintenance in Real Time
Real-time analytics allow organizations to perform predictive maintenance through edge computing. This approach allows for faster response times and reduced network latency. With the help of edge computing, organizations can collect and analyze vast amounts of data from sensor devices and other IoT sources. Real-time analytics algorithms can then be applied to this data to identify patterns and anomalies that may indicate potential equipment failures or maintenance needs.
This approach not only helps businesses save costs but also schedule maintenance activities at optimal times to minimize disruption to operations.
Moreover, edge computing enables real-time monitoring of equipment performance across multiple locations, making it easier for organizations to track their assets’ health. This approach provides valuable insights into how different factors affect equipment performance and helps businesses optimize their operations accordingly.
Edge computing is a game-changer in enabling predictive maintenance through real-time analytics. The overall benefits also include operational efficiency and optimal asset management.
The Road Ahead: Embracing the Power of Real Time for Business Success
The road ahead for businesses is paved with the power of real-time analytics. It will allow businesses to monitor their operations in real time, identifying patterns and trends that can be leveraged for competitive advantage. This means that they can adjust their strategies on the fly, optimizing their performance and increasing efficiency.
Additionally, real-time analytics will help businesses to deliver custom products and services based on customer needs. By analyzing customer data, companies can deliver tailored solutions that meet individual requirements and preferences. To fully embrace the power of real-time analytics, companies need to invest in cutting-edge technologies such as artificial intelligence and machine learning. Companies that adopt this technology early on will be well-positioned for growth and profitability in the years ahead.
Real-Time Analytics: An Overview
Expect real-time analytics to continue to grow in importance and become even more widespread across different industries.
One of the key use cases for real-time analytics is in healthcare. With an increasing amount of data generated by medical devices and patient monitoring systems, real-time analytics play a crucial role in helping doctors and healthcare providers make faster and more accurate diagnoses.
In the retail industry, real-time analytics are used to provide personalized recommendations and targeted marketing campaigns based on individual customer behavior.
Real-time analytics also play a critical role in the financial services industry by providing fraud detection and prevention capabilities. This will help banks and other financial institutions protect their customers’ assets while reducing their own risk exposure.
Overall, real-time analytics is set to revolutionize many industries over the coming years by providing businesses with valuable insights that can be used to drive growth and improve operational efficiency.
Advancements in Streaming Data and Machine Learning for Real-Time Analytics
Real-time analytics has become increasingly important in the world of data processing, and advancements in streaming data and machine learning make it possible to analyze data as it is generated.
One of the most significant advancements is the ability to process streaming data at scale. With the proliferation of IoT devices, social media platforms, and other sources of real-time data, there is a growing need for tools that can handle large volumes of continuously flowing information.
Machine learning algorithms are also becoming more sophisticated, allowing organizations to gain insights from complex datasets in real-time. Another key development is the integration of real-time analytics with business processes. By using machine learning algorithms to predict outcomes and identify patterns, organizations can make informed decisions based on up-to-date information.
As this technology continues to evolve, we can expect even more exciting use cases for real-time analytics across industries.
Predictive Analytics and Cloud Computing
As the use of real-time analytics continues to grow, predictive analytics and cloud computing are emerging as key components of future fraud detection, supply chain optimization, and risk management.
With predictive analytics, organizations can use historical data to forecast future events and take proactive measures to prevent potential risks. This allows for more accurate predictions and faster response times when dealing with potential fraud or supply chain disruptions.
It also enables organizations to manage risks more effectively by providing real-time insights into potential vulnerabilities. In the future, we can expect to see even greater integration between predictive analytics and cloud computing as these technologies become more sophisticated. This will allow companies to optimize their supply chains by predicting demand patterns and identifying potential bottlenecks before they occur.
Ultimately, the combination of predictive analytics and cloud computing offers enormous potential for businesses looking to stay ahead of the curve in terms of fraud detection, supply chain optimization, and risk management.
Healthcare Analytics, Social Media Analytics, Mobile App Analytics, Video Streaming Analytics, Advertising Optimization
Real-time analytics is gaining popularity in various industries, including healthcare, social media, mobile app development, video streaming, advertising optimization, and financial analysis.
Healthcare analytics can help professionals monitor patient health data in real-time and make timely decisions to improve treatment outcomes. Social media analytics can help companies track customer engagement with their brand and analyze consumer behavior patterns to improve marketing strategies.
Mobile app analytics can provide insights into user behavior and preferences to enhance app performance. Video streaming analytics can help content creators identify popular content and optimize their video delivery systems for better user experience.
Advertising optimization through real-time analytics allows businesses to adjust ad campaigns on the fly for maximum impact. Real-time analytics will continue to play a critical role in shaping the future of various industries by enabling faster decision-making based on accurate data insights.
Real-Time Inventory Management, Traffic Flow Analysis, Environmental Monitoring
Real-time inventory management will allow retailers to optimize their stock levels based on consumer demand. By analyzing data from point-of-sale systems, retailers can make quick predictions about product demands and make appropriate adjustments to their inventory.
Traffic flow analysis can be used to improve urban planning and reduce congestion by analyzing real-time data from sensors and cameras. This will help city planners optimize traffic signals, reduce travel times, and improve safety for pedestrians and cyclists.
Environmental monitoring is also an important use case for real-time analytics. By collecting data on air quality, water pollution, and other environmental factors in real-time, scientists can quickly identify emerging threats to public health or ecosystems.
Overall, real-time analytics offers a powerful tool for addressing emerging challenges in these fields by providing accurate predictions based on real-world data. As technology continues to evolve, we can expect even more exciting use cases for this powerful tool in the years ahead.
Image used under license from Shutterstock.com