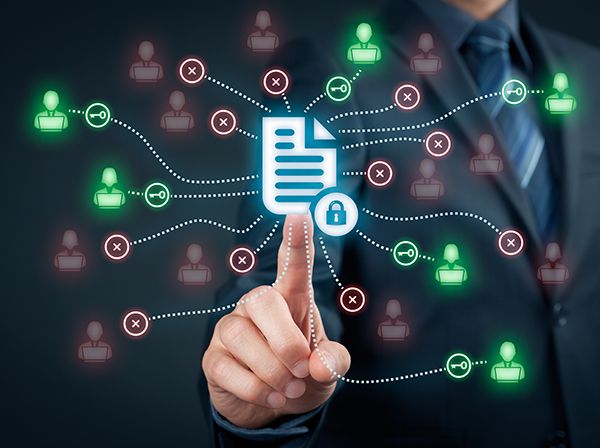
Large organizations want to create a flexible environment to innovate and respond quickly based on new data insights. But at the same time, these businesses want some structure for good Data Quality, data fit for consumption, simplifying and speeding up data access. Using a data mesh, which is a decentralized data architecture (collecting, integrating, and analyzing data from disconnected systems), with federated Data Governance (focusing on enablement and access in compliance with privacy requirements) fits the objectives. This article will explain how data mesh and Data Governance intersect and explore the benefits of each.
Data Mesh: A Decentralized Architecture
A decentralized architecture makes up the core of a data mesh. Hub nodes (the blue boxes above) represent the domains that serve up their data to other company sections. Think of a hub node as a field of business knowledge using a combination of hardware devices or software services around a particular context. For example, human resources (HR) may have one hub, and finance has a different hub.
Spokes connect the hub nodes in a network, directing data traffic to or from the nodes through a central point, so data flows quickly across multiple networks. For example, through spokes, HR can simultaneously connect to various sections like finance, customer support, or any other department.
Links, physical cables or wires, or a software connection weave between the spokes. So, HR may link its data only to finance with no other domains connected.
A data mesh describes the hub, spoke, and link model, routing data between hub nodes through spokes and multiple links. The links provide data mesh options for flexibility. For example, if a spoke from HR fails, but finance has a good connection, then finance can continue to get HR data.
A data mesh in one organization looks very different from that in others. The construction depends on what the individual business needs.
Why Do Companies Choose a Data Mesh Architecture?
Companies choose a data mesh to overcome the limitations of “centralized and monolithic” data platforms, as noted by Zhamak Dehghani, the director of emerging technologies at Thoughtworks.
Technologies like data lakes and warehouses try to consolidate all data in one place, but enterprises can find that the data gets stuck there.
A company might have only one centralized data repository – typically a team such as IT – that serves the data up to everyone else in the company. This slows down data access because of bottlenecks. For example, having already taken days to get HR privacy approval, the finance department’s data access requests might then sit in the inbox of one or two people in IT for additional days.
Instead, a data mesh puts data control in the hands of each domain that serves that data. Subject matter experts (SMEs) in the domain control how this data is organized, managed, and delivered.
The resulting flexible, federated technology through domain Data Management gives organizations three core benefits:
- Simplicity: Users across the organization have self-service access to the data they need. They can find and interface with data on the fly independently, without having to pass through a departmental gatekeeper.
- Scalability: Data mesh distributes data across different organizational domains so that they have control of that data. If the core business wishes to expand or pare back its business unit, it can do so quickly while continuing to provide access to the other domains.
- Reliable remote connectivity: Data mesh connects and integrates data from various separate systems. Its flexible network can reroute data requests should a link or spoke fail.
Drawbacks of Using Data Mesh Alone
A data mesh without any Data Governance faces two disadvantages:
Complexity: While users can obtain data quickly from any domain, getting data from multiple domains can grow quite complex. Users find that each person or team has a unique system or process to allow access to their data.
For example, HR may require users to query data using JavaScript, whereas finance responds only to data queries formed in Visual Basic.
Imagine if each department, spread across an enterprise, has a specific set of programming languages or processes to get the data. Then a department would have a headache getting combined data sets by patching all this information together.
Low performance: Since each domain can uniquely deliver its data through the mesh, combining data from multiple domains can take time. Querying this data will be limited by the slowest connection to a specific domain.
Furthermore, individuals or teams face a steep technical learning curve to make their domain data available across their businesses. Unless someone has deep expertise in the organization’s decentralized architecture, users need to put in the time to figure out how to get combined datasets efficiently. Data mesh’s complexity and low-performance issues highlight a lack of organizational coherence.
Unifying the Company with a Data Product Mindset
To counteract issues with complexity and low performance, an organization with data mesh should adopt a data product mindset. In this approach, each domain takes the role of an internal vendor, responsible for the refined data it delivers across the mesh and how this service meets the needs of its customers, other business units, or external customers.
Consequently, each domain defines what its data product does, why the other domains need it, and its key capabilities. Then, that team or individual associated with the domain promotes these data products in an internal data marketplace, determines when to release them, and supports its customers (other domain members).
A domain can offer one or more products from the data it produces, and schedule when to release a product. Combining the internal data products underlies the goods or services that external customers purchase from the company.
Domains adapt their products for a better fit across the organization or go by the wayside. A healthy culture sets the stage for multiple domains to find technological and systematic commonalities to develop their products economically.
Guiding Domains with a Federated Data Governance Model
Data Governance comes into the picture to guide data product creation and usage across the organization. Without Data Governance, a company can be slowed by politics, increasing complexity, and decreasing performance.
For example, one group requires JavaScript programming language for data access, but another domain requires Ruby. The other domains want to simplify and standardize but must agree on what programming language to use. Federated Data Governance evens the organization’s data marketplace, helping the company meet operational objectives through its data products.
In the federated model, the business sets up a “community of practice” or a guild of data architects. The guild contains at least one representative per domain who work together to agree on standards and recommend where to apply them to data products.
Typically, a core group or a center of excellence (CoE) moderates the discussions around the standards, and steps in when there is conflict. The guild designs requirements at a high level so that users across the company find different data products interoperable.
A well-run federated Data Governance framework does the following:
- Tames complexity by simplifying ownership: Federated Data Governance makes the person or team who owns the data product accountable. Additionally, Data Governance clarifies general subject boundaries between domains, making it more explicit who oversees what information.
- Decreases latency by reducing duplication: As the guild of data architects comes together, they develop greater awareness of other data products created by different domains. As a result, they are more likely to adopt another team’s tools or processes than create their own.
Consequently, this kind of Data Governance saves reinventing the wheel and improves efficiencies in accessing data throughout the company. Teams better understand what their data products bring to the organization and can more easily navigate and use ones developed by other groups.
Conclusion
Data mesh with federated Data Governance balances expertise, flexibility, and speed with data product interoperability among different domains. With a data mesh, the people with the most knowledge about their subject matter take charge of their data.
In the future, organizations will continue to face challenges in providing good, federated Data Governance to access data through a data mesh. Data ownership gets tricky as companies adapt to a fluctuating economy by laying off, re-assigning, or hiring workers. But if companies keep in mind the balance between their data mesh and federated Data Governance, they will more easily navigate these difficulties and thrive.
Image used under license from Shutterstock.com