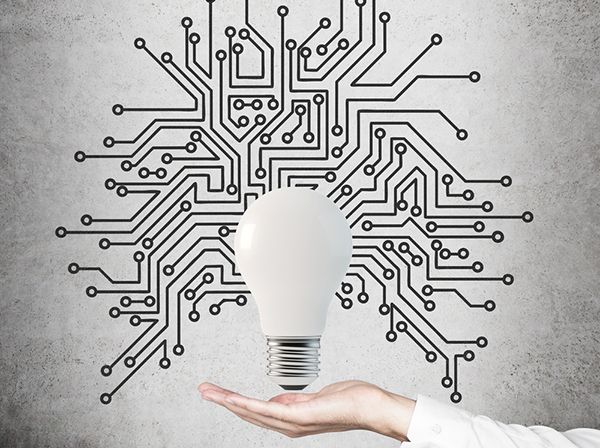
In 2023, machine learning (ML) is a powerful enabler for Data Management, revolutionizing the way organizations handle and analyze vast amounts of data. ML, a subset of artificial intelligence (AI), offers various approaches to tackle Data Management challenges. With the ever-increasing “volume, velocity, and variety of data” being generated today, traditional methods of Data Management have become inadequate. Machine learning techniques and use cases provide a solution by enabling automated data analysis and interpretation of complex datasets.
Machine learning algorithms are unique in their ability to learn from data and improve their performance over time without explicit programming. This capability makes it particularly well-suited for addressing challenges associated with Data Management.
Use of Machine Learning Beyond Traditional Data Management
Machine learning has revolutionized traditional Data Management techniques by enabling advanced functionalities such as anomaly detection, image recognition, and natural language processing. These capabilities have opened up new possibilities for businesses to extract valuable insights from their data and make informed decisions.
- Anomaly detection is a key application of machine learning in Data Management. By leveraging algorithms that learn patterns and trends from historical data, anomalies can be identified in real-time. This is particularly useful in fraud detection, where machine learning models can analyze vast amounts of transactional data to flag suspicious activities.
- Image recognition has become increasingly crucial as organizations generate vast amounts of visual content. Machine learning algorithms can be trained to recognize objects, faces, or even classify images based on specific criteria. This technology finds applications in various sectors like healthcare (diagnosing medical images), autonomous vehicles (identifying obstacles), and e-commerce (visual search).
- Natural language processing (NLP) empowers machines to understand and interpret human language. By using sophisticated algorithms and linguistic rules, NLP enables tasks such as sentiment analysis, language translation, chatbots, and voice assistants. These capabilities have transformed customer service experiences and opened new avenues for communication between humans and machines.
In 2023, ML will continue to transform Data Management across industries. From enhancing customer relationship management systems to improving supply chain optimization, ML algorithms will drive strategic decision-making.
Use Case: Enhancing Data Quality Through ML
In the realm of Data Management, ensuring high-quality data is paramount for accurate analysis and decision-making. ML algorithms have emerged as powerful tools to enhance Data Quality by automating various tasks. ML techniques can identify and rectify inconsistencies, errors, and missing values in datasets, thereby reducing human error and improving data accuracy. One application of ML for Data Quality enhancement is anomaly detection. By learning patterns from historical data, ML models can identify outliers or anomalies in real-time datasets, enabling swift action to rectify any issues.
ML algorithms can also identify duplicate records across large databases and merge them into a single accurate entry. Furthermore, ML algorithms can also predict missing values by analyzing existing patterns within the dataset.
Use Case: Automating Data Integration
Data integration plays a crucial role in managing and analyzing large volumes of data efficiently. However, traditional methods often require manual effort, making the process time-consuming and prone to errors. ML can revolutionize data integration by automating various tasks. Using ML algorithms, organizations can automatically match and link disparate datasets from different sources, eliminating the need for manual mapping. These algorithms learn from historical data mappings and patterns to identify similarities and create accurate links between datasets.
Moreover, ML techniques enable automated data cleansing and transformation. Algorithms can identify inconsistencies or missing values within datasets and suggest appropriate actions to improve Data Quality. By automating data integration with machine learning, organizations can streamline their operations, reduce costs, enhance accuracy, and gain valuable insights from their Data Management processes.
Use Case: Predictive Analytics for Efficient Data Storage and Retrieval
Predictive analytics is set to revolutionize the way organizations store and retrieve their vast volumes of data. By leveraging ML algorithms, predictive analytics can analyze historical data patterns and make accurate predictions about future storage needs. This proactive approach enables businesses to optimize their data storage infrastructure, avoiding unnecessary costs associated with overprovisioning or underutilization.
Moreover, predictive analytics can enhance data retrieval processes by predicting user behavior and preferences. By analyzing user interactions with stored data, algorithms can anticipate which information will be accessed more frequently or which files may become obsolete over time. This foresight allows for intelligent caching strategies, prioritizing frequently accessed data and reducing retrieval latency.
Use Case: Machine Learning for Real-Time Data Processing and Analysis
Real-time data processing and analysis is crucial for organizations to make informed decisions in today’s fast-paced business environment. ML techniques play a significant role in enhancing the efficiency and accuracy of real-time data processing. This enables proactive decision-making, such as identifying potential issues or opportunities as they happen.
ML models can continuously learn from incoming data streams, improving their accuracy over time. They can automatically classify incoming data into relevant categories or predict outcomes based on historical patterns. These algorithms can provide real-time insights, which organizations can use to optimize processes, personalize customer experiences, and detect fraud or cyber threats before they happen.
Use Case: Improving Data Security with ML Techniques
As data continues to grow exponentially, organizations face an increasing challenge of safeguarding their valuable information from potential threats. ML techniques have emerged as a powerful solution for enhancing data security in the digital age. By leveraging advanced algorithms, ML can identify patterns and anomalies in vast datasets, enabling proactive threat detection and prevention.
By analyzing historical data and identifying abnormal patterns, ML models can flag potential security breaches or unauthorized access attempts.
Finally, ML can be used to enhance authentication systems by analyzing user behavior patterns and identifying suspicious activities that may indicate fraud or unauthorized access. This enables organizations to implement more robust authentication mechanisms that adapt to evolving threats.
As we move forward in 2023, the utilization of ML techniques in Data Management is expected to rise across various sectors. In this context, understanding sector-wise ML use cases becomes crucial for organizations seeking to leverage data-driven strategies effectively.
Healthcare Analytics
Healthcare analytics will continue to make significant strides in leveraging ML to enhance patient care and operational efficiency. ML algorithms can analyze patient records and treatment data such as lab reports or genetic information to detect patterns and predict treatment procedures. This enables healthcare providers to make more accurate diagnoses and develop personalized treatment plans tailored to individual patients.
Additionally, ML can streamline healthcare administrative tasks such as scheduling appointments and managing resources efficiently.
Financial Services
In the financial services sector, ML algorithms in fraud detection and risk assessment are expected to enhance security measures and mitigate potential risks. By leveraging advanced Data Management techniques, ML algorithms can analyze vast amounts of financial data to identify patterns and anomalies that may indicate fraudulent activities. These algorithms can adapt and learn from new emerging fraud patterns, enabling financial institutions to take immediate action.
Additionally, ML algorithms can aid in risk assessment by analyzing historical data, market trends, and customer behavior to predict potential risks accurately. Such predictive models can help financial institutions make informed decisions regarding lending practices, investment strategies, and customer profiling, ultimately leading to improved operational efficiency and reduced losses due to fraud or risky investments.
Retail Analytics
Retail analytics is revolutionizing the way businesses operate. By harnessing the power of ML, retailers are able to drive sales, optimize inventory management, and enhance customer experience like never before. ML algorithms analyze vast amounts of customer data to identify patterns and trends, enabling retailers to make data-driven decisions in real-time. Sales forecasting models based on ML algorithms accurately predict consumer demand, allowing retailers to stock their shelves with the right products at the right time.
Additionally, ML-powered recommendation systems can suggest appropriate products to customers based on past buying records. This not only increases sales but also enhances customer satisfaction and loyalty. With ML at its core, retail analytics is transforming the retail industry.
Manufacturing Optimization
In the manufacturing sector, ML is revolutionizing quality control and predictive maintenance processes. ML algorithms can analyze vast amounts of data collected from sensors, machines, and production lines to identify patterns and anomalies. This enables manufacturers to detect defects in real-time, ensuring product quality while minimizing waste and rework. Moreover, ML algorithms can predict equipment failures by analyzing historical data on machine performance.
This allows manufacturers to schedule maintenance proactively, preventing costly downtime and maximizing operational efficiency. By leveraging ML for quality control and predictive maintenance, manufacturers can optimize their production processes, reduce costs associated with defects or breakdowns, enhance product reliability, and ultimately improve customer satisfaction.
Social Media Analytics
Social media analytics is rapidly evolving, and it will increasingly rely on ML to understand consumer sentiment and drive effective marketing strategies. ML algorithms can analyze vast amounts of social media data, extracting valuable insights to gauge public opinion toward brands, products, or services. By accurately understanding consumer sentiment, businesses can tailor their marketing campaigns to resonate with target audiences.
ML-powered sentiment analysis models can detect emotions and opinions expressed in social media posts, comments, and reviews. This enables companies to identify emerging trends or issues promptly and respond proactively.
Telecommunications Network Optimization
Telecommunications network optimization is a crucial aspect in ensuring enhanced connectivity and network performance. ML will continue to play a pivotal role in providing intelligent solutions for this sector. ML-driven algorithms can analyze vast amounts of data, including network traffic patterns, user behavior, and equipment performance. By utilizing this data, ML models can accurately predict network congestion points and proactively allocate resources to optimize bandwidth utilization.
Moreover, ML algorithms can identify potential faults or anomalies in the network infrastructure, enabling proactive maintenance and minimizing downtime. These ML-driven solutions will ultimately lead to improved connectivity for end-users, reduced latency, and enhanced overall network performance.
Environmental Monitoring
Environmental monitoring is a critical aspect of ensuring sustainability and reducing risks in various sectors. By leveraging ML algorithms, Data Management in environmental monitoring can be significantly enhanced. ML can analyze large volumes of data collected from sensors, satellites, and other sources to identify patterns and predict changes in the environment.
ML algorithms can help in detecting and predicting natural disasters such as floods, wildfires, or earthquakes with greater accuracy and speed. This enables proactive measures to be taken for risk mitigation and disaster response planning.
Additionally, ML can assist in monitoring air quality, water pollution levels, deforestation rates, and wildlife habitats to inform effective conservation strategies. By harnessing ML capabilities for environmental monitoring, organizations across sectors can make informed decisions that promote sustainability while minimizing potential risks to ecosystems and human populations.
Agricultural Data Analysis
Agricultural data analysis is witnessing a revolutionary transformation through the application of ML techniques. Precision farming, a key area of focus, leverages ML algorithms to analyze vast amounts of agricultural data and optimize crop yield. By integrating data from weather sensors, satellite imagery, and IoT devices, ML models can provide insights on soil health, irrigation requirements, pest infestations, and crop growth patterns.
These models help farmers make informed decisions about fertilizer usage or irrigation schedules for maximizing productivity and minimizing resource wastage. Additionally, ML-driven predictive analytics enables early detection of diseases or nutrient deficiencies in crops, aiding timely interventions. The use of ML in agricultural data analysis promises to enhance efficiency and sustainability in farming practices for improved food production.
Energy Management
The field of energy management will witness significant advancements in Data Management through the integration of machine learning technologies. ML algorithms will play a crucial role in optimizing energy consumption and reducing carbon footprints.
By analyzing vast amounts of historical and real-time data from various sources like weather forecasts, and smart meters, ML models can accurately predict energy demand patterns. This enables organizations to proactively adjust their energy usage to avoid peak hours and optimize efficiency.
Additionally, ML algorithms can identify areas of excessive energy consumption within buildings or industrial processes, leading to targeted interventions for reducing waste and improving overall sustainability practices.
Image used under license from Shutterstock.com