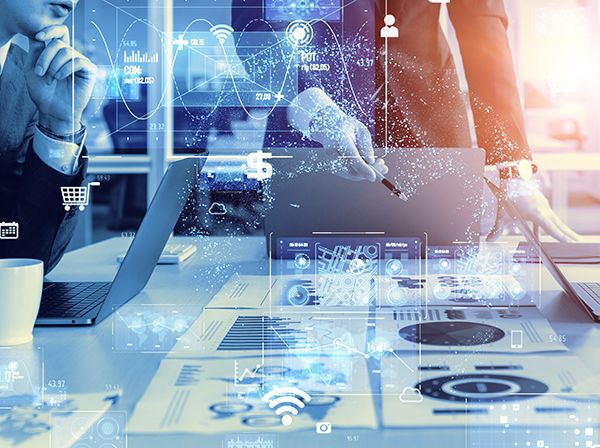
The concept of managing “data as a product” involves a paradigm shift. By treating data as a product designed for consumer use, rather than a pool of semi-chaotic information, businesses can increase their profits. Many businesses have set up customized data pipelines – or other extreme and expensive steps – in unsuccessful efforts to maximize the value and use of their data.
Managing data as a product should result in high-quality data that is easy to use and can be applied to different projects.
Businesses are constantly making significant investments in an effort to improve their data architecture with the goal of streamlining research, yet researchers continue to have difficulty finding, using, and customizing the data they want. This difficulty is primarily the result of viewing data as a tool, rather than as a product being made available to consumers. The end result is massive amounts of data being stored in data lakes and warehouses that may never be used, or is used minimally.
What Is Data as a Product?
To eliminate any confusion, data as a product is different from data products.
In his book, “Data Jujitsu: The Art of Turning Data into Product,” DJ Patil, a former U.S. Chief Data Scientist, defined a data product as “a product that facilitates an end goal through the use of data.” His description includes such tools as data dashboards, data warehouses, and self-driving cars.
Data as a product, on the other hand, is a mindset that combines tools and strategy to treat data as a product consumed by internal customers (in-house staff). The “product” should include such features as discoverability, explorability, understandability, security, and trustworthiness. The data should be consumer-friendly and of high quality.
Benefits of Managing Data as a Product
The reason for managing data as a product is to improve Data Quality. By viewing data as a product, you can see the data as something that can be improved upon. Data becomes something needing improvements to satisfy your consumer base. The goal of a “data as a product” philosophy is to provide high-quality, trustworthy data that is easy to access and work with.
Consider the behavior of a modern online consumer who wants to purchase a summer shirt. The online consumer has come to expect the ability to:
- Trust the seller will provide what is ordered, while keeping personal information private (some customers only shop at Amazon, Etsy, and a few other “trusted” vendors)
- Search for different kinds of shirts (a search for short-sleeve summer shirts)
- Find details about the shirts displayed on the computer screen (prices, colors, sizes, type of material)
- Select the desired shirt with the correct size and color
- Order and pay for the shirt and where it should be delivered
- Receive the shirt within a certain amount of time (the estimated delivery time)
Applying a similar model to the concept of “data as a product” produces the following consumer model, which expects the ability to:
- Trust the desired data
- Search for different topics within the organization’s data storage
- Find details about the data (metadata is useful for this process)
- Select the desired data
- Access the data
- Receive the data
- Work with the data
How to Apply Product Management Principles to Data
Consider the best products you use. They are easy to locate, understand, and use, and are consistent in meeting our expectations. These features are not coincidental, but part of a deliberate effort. The delivery system is also a deliberate decision. A person, or team, made decisions that maximized the easy use of these products, provided a trustworthy delivery system, and delivered high quality (or at least reasonable quality).
Applying product management principles to data includes attempting to address the needs of as many different potential consumers as possible. This requires developing an understanding of the consumer base. The consumers are typically in-house staff accessing the organization’s data. (The data is not being “sold,” but is being treated as a product available for distribution, by identifying the consumers’/in-house staff’s needs.)
From a big-picture perspective, the business’s goal is to maximize the use of its in-house data. Managing data as a product requires applying the appropriate product management principles. These principles are listed below.
- Maintain and understand a map of the organization’s data flows: By tracking the “product,” a data steward can determine which consumers are using the data, and for what projects. The map should be as detailed as possible.
- Seek feedback from the consumers: An extremely important requirement involves listening to and understanding the needs of the consumer base. Having developed a map of the business’s data flows, individuals using the data can be interviewed regarding their frustrations when working with the organization’s data. This feedback can be used to find solutions that make working with the data easier and more efficient.
- Make improvements incrementally: The largest problems facing the most staff should be dealt with first, satisfying the most people within the consumer base.
- Establish standardized procedures for working with the data across the business: The use of standardized processes minimizes the amount of time spent learning different processes and improves overall efficiency.
- Provide self-service analytics for your consumers: Self-service analytics is a way of collecting and analyzing information to develop business intelligence. It allows users to access and analyze their data, and develop useful insights. The primary difference between traditional analytics solutions and self-service analytics is that the former requires special training and the scheduling of projects, while the latter can be used spontaneously by people lacking a technical degree.
Data Mesh
Developed in 2018 by Zhamak Dheghani, the director of emerging technologies in North America for ThoughtWorks, data mesh has become a controversial topic in Data Management discussions. It offers an alternative to the shortcomings of a centralized architectural model.
Data mesh is an architectural model that is complemented and supported by the philosophy of data as a product. The concept has generated some interest among corporations as an alternative to storing data in data lakes and data warehouses.
The data as a product philosophy is an important feature of the data mesh model.
Data mesh is a decentralized form of data architecture. It is controlled by different departments or offices – marketing, sales, customer service – rather than a single location. Historically, a data engineering team would perform the research and analytics, a process that severely limited research when compared to the self-service approach promoted by the data as a product philosophy, and the data mesh model.
The use of a data mesh architecture does not eliminate the need for a data engineering team, but instead shifts their responsibility to finding and developing the best data solutions for the organization. (Some believe data mesh may not be worth the effort.)
In a data mesh organization, domain owners (one or two in each location, department, or office) are responsible for maintaining a uniform standard for all the organization’s data. This allows staff from any location access to data stored at other locations. During her keynote presentation at DATAVERSITY’s Data Architecture Online (DAO), Zhamak Dheghani said,
“Everyone in the organization has responsibility for their data. As the organization grows with new use cases and integrates new touchpoints, a new domain gets added with a new team responsible for that data.”
Image used under license from Shutterstock.com