Companies rely on Data Governance, the formal Data Management of people, technology, and practices, to balance data risks and opportunities. Managing Data Governance takes on even more importance across the data lifecycle, from data planning to data disposal, as shown in the diagram below
Organizations tend to neglect more comprehensive Data Governance throughout the lifecycle. They take a project, team, or programmed approach and can miss governing some lifecycle activities, like data storage, after completing a project.
This less comprehensive Data Governance mindset leaves companies vulnerable. Take British Airways (BA), which had to deal with a 2018 cyberattack and was fined 20 million pounds for a GDPR data breach.
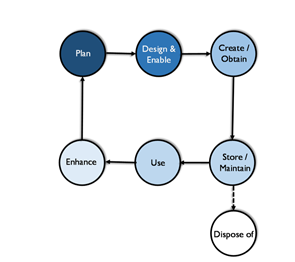
As part of the BA incident, the attacker accessed payment cards with personal and financial information. BA had logged this information and stored it for 95 days to test a software application under development and release in 2015.
Unfortunately, BA continued to collect the payment card data, without a good business reason, even after the project ended. Three years later, in 2018, regulators found this data processing relic, which was costly to BA in both money and reputation.
Doing Data Governance throughout the data lifecycle catches and updates overlooked data practices, helping a company avoid risks and capitalize on benefits. This article will discuss some tools to make managing Data Governance across the data lifecycle easier:
- Note risks and rewards across the data’s lifecycle
- Use a Data Governance framework for the data lifecycle
- Train employees to be data literate
- Track how Data Governance removes data debt
Note Risks and Rewards for the Data’s Lifecycle
Like business products and services, business drives data activities that flow through an organization. Each step in the data lifecycle results in risks and rewards.
“Risks” describe the challenges in data practices to meet regulations and avoid data breaches. “Rewards” represent the opportunities for data practices to foster innovation and growth across the business.
By listing risks and rewards for each lifecycle phase in line with business objectives, companies can start to see Data Governance structures (roles, policies, and rules) that need to exist. Data managers can consider some general goals for each step (e.g., creating and obtaining data can risk collecting information that is not permitted by data regulations).
But specific challenges and advantages depend on a business’s strategy, industry, and culture.
Take the table below as a starting point to add or modify for business use:
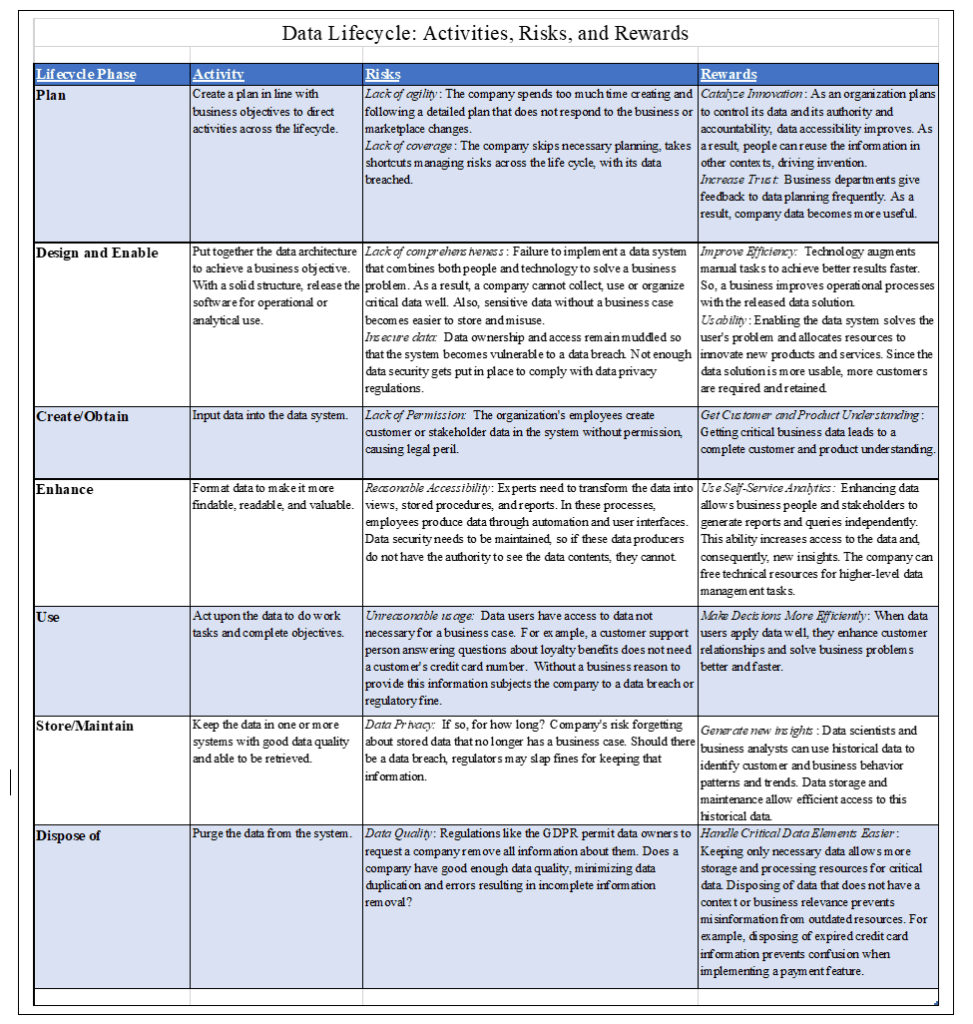
Knowing risks and rewards across the data lifecycle provides the whys for organizational Data Governance. Also, it helps inform about a Data Governance framework that works for the business.
Use a Data Governance Framework for the Data Lifecycle
Any Data Governance framework for the data lifecycle needs to be sensitive to a company’s needs while capturing core Data Governance mechanisms. Combining ideas from Gregory Vial’s “Data Governance in the 21st Century Organization” article and Robert Seiner, a Data Governance expert and DAMA awarded professional, achieves both.
Vial describes five key Data Management domains that organize Data Governance authority and accountability. One of these areas includes the data lifecycle. So, constructing a Data Governance framework with each phase of the data lifecycle makes sense.
Through his Non-Invasive Data Governance® (NIDG) approach, Seiner has identified core foundational components needed for a successful Data Governance program. Seiner has experience looking at existing Data Governance structures in various organizations and improving them to meet business requirements. He lists:
- Data: The assets a company governs
- Roles: Accountability of an organization’s employees that is made formal
- Processes: Application enforcement that gives people an appropriate level of data access and accountability to succeed in each step they take
- Communications: The training and education every person in an organization needs to support business success with data
- Metrics: The organization formalizes quantitative measurements of Data Governance activities
- Tools: Technology and things purchased or developed to support Data Governance activities
Combining both the data lifecycle conception as a series of Data Management components and the foundational Data Governance modules results in a Data Governance framework matrix. See below:
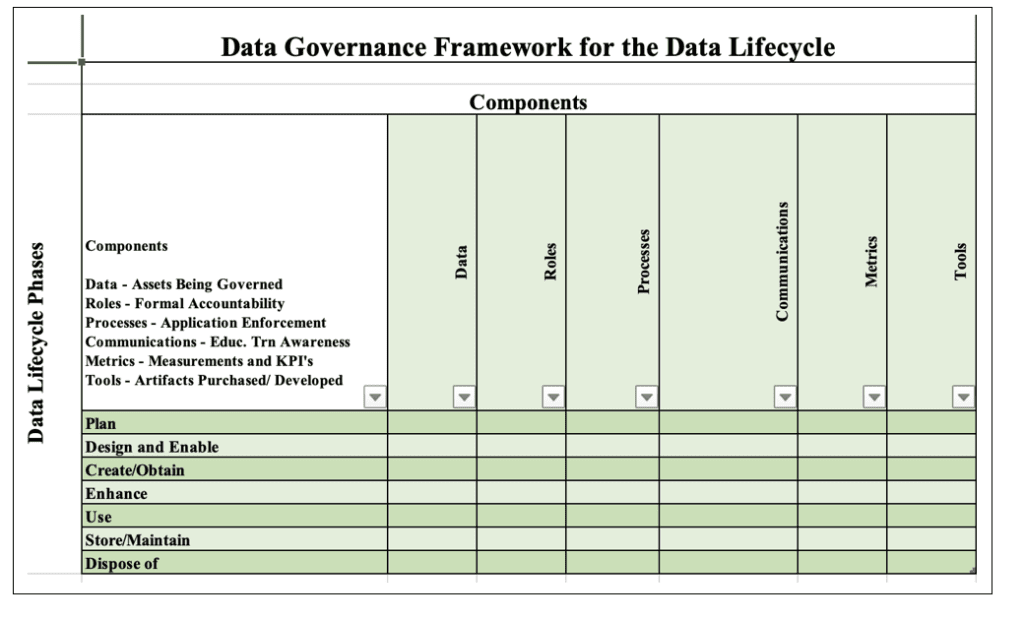
Place this “Data Governance Framework for the Data Lifecycle” matrix next to the “Data Lifecycle: Activities, Risks, and Rewards” matrix. By doing so, data managers and their teams can start to develop Data Governance services.
For example, say a company wants to increase data lifecycle rewards by enhancing data assets, making data more accessible to nontechnical business personnel through self-service. A data manager could meet with the business team and plan a data catalog (a tool) promoting self-service.
This executive/business team could then cover other Data Governance ingredients necessary for a self-service data catalog: roles, processes, communication, and metrics. The framework has space for jotting Data Governance approaches for each.
Train Employees to Be Data Literate
A Data Governance framework can provide some guidance; however, as Seiner says, “Governance will always be about people’s behavior.” To optimize people’s Data Governance behaviors, it helps if they have basic data literacy.
Data literacy means that people know and feel comfortable reading, working, arguing, and analyzing data sets. Unfortunately, according to a 2020 Human Impact of Data Literacy report, only 21 percent of the global workforce feel fully confident of their data literacy skills, and 36 percent would find an alternative method to complete a task without using data.
That people want to stay away from data does not seem surprising, given that data literacy education tends to be fragmented (people pick up pieces of these skills through their experience or, if they are lucky, through math classes in school). However, businesses do not have the luxury to wait for their workers, stakeholders, and customers to fill in their data literacy gaps through self-teaching.
Each data lifecycle step requires people to perform some action with data that needs a basic data literacy level. Without it, people cannot implement Data Governance efficiently in their work to reduce legal risk or to grow their business. For example, misunderstanding how to read data and graphs generated by self-service analytics lowers the innovation potential and increases misuse.
In the British Airways breach mentioned above, if the employees had received training in questioning data sources, someone may have questioned the stored payment card data’s origins in plaintext log files. In figuring out that BA had produced and stored sensitive and personal data, employees could’ve flagged management, who would’ve have taken steps to protect or handle the information better.
Track How Data Governance Removes Data Debt
Each time any employee plans, designs, enables, creates, obtains, enhances, uses, stores, maintains, or disposes of data, it incurs some sort of data debt. The company risks that some personal data gets overlooked and data privacy becomes vulnerable.
These data costs accumulate when delaying needed Data Management and decrease with good Data Management. When BA produced payment information in 2015 to test a feature quickly, BA had data debt. This data debt increased sharply by wrongly storing and maintaining the information and not developing a plan to dispose of information no longer needed.
BA’s data debt, accumulated over three years until the data breach, ended up with the GDPR demanding payment of 20 million pounds, and made the company subject to additional litigation. Good Data Governance would’ve paid down that debt.
Having a Data Quality reporting process that records data debt through issues and resolutions provides another way to get effective Data Governance across the data lifecycle. Data literate employees can flag problems leading to excessive data debt and initiate processes toward resolution.
Tickets generated in the Data Quality reporting process can be compared to the Data Governance framework for the data lifecycle, highlighting improvements executives should consider in data, roles, processes, communications, metrics, and tools. These Data Governance upgrades can justify themselves over the data lifecycle by the debt they pay down.
Summary
Throughout the data lifecycle, Data Governance needs to be continuous to meet regulations, and flexible to allow for innovation. Understanding risks and rewards through each lifecycle phase and addressing them through a Data Governance framework through the data lifecycle starts organizations on the path toward better Data Management. Data literacy and tracking data debt underpins valuing and executing good Data Governance for each data lifecycle activity.
Image used under license from Shutterstock.com