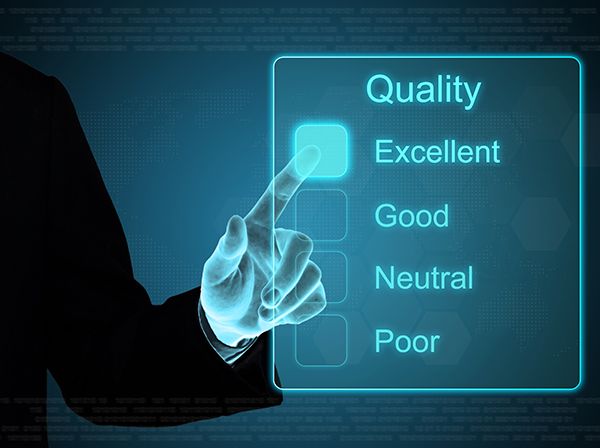
For the data-driven, high-volume businesses of today, improving Data Quality is essential to ensure trustworthy data and operational efficiency. But the process doesn’t have to be daunting, said Ryan Doupe, VP and chief data officer at American Fidelity Assurance. In a presentation at DATAVERSITY’s Enterprise Data Governance Online event, Doupe laid out a nine-step program for better Data Quality that is affordable, approachable, and scalable.
“This approach is aimed to be both practical and tactical, and can be implemented without any incremental investment in the form of money or labor,” he explained. Below, we’ve highlighted how and why to implement each of these nine steps, according to Doupe.
1. Identifying Critical Data Elements (CDEs)
Your organization might manage thousands of data elements, so figuring out the critical data elements (CDEs) – data elements that are vital to the successful operation of the business – will create a sense of focus. Which data elements do the heavy lifting within operations? For example, if a company regularly deals with supply chain logistics, data such as height and width will be indispensable.
Doupe recommends sending a survey to key team members (executive team, Data Governance committee, data stewards, data curators, and other “heavy data users”) to help identify CDEs. The ideal number of CDEs will vary from company to company:
“If this is your company’s first time formally approaching a Data Quality process, I would suggest starting with 10 to 15 critical data elements,” said Doupe. “If your organization is familiar with Data Quality approaches, I would recommend 20 to 30. Anything more than 30 becomes unwieldy.”
2. Clarifying Definitions
One CDE might have multiple definitions across departments within an organization, so it’s critical to implement a common business language. “Tax ID,” for example, might apply to either an individual or an entire organization, opening the door for confusion and error.
When compiling definitions, Doupe follows several do’s and don’ts:
- Do amass CDE definitions in a spreadsheet (or a data catalog tool, if your organization is mature enough) where team members can easily access it
- Don’t use opaque, circular labels, or so-called “cheeseburger” definitions (“a cheeseburger is a burger with cheese”) that offer nothing beyond the obvious
- Do collect the name of each CDE and its corresponding definitions, synonyms, and acronyms used frequently by the organization
- Don’t include excessive jargon and tech-speak – definitions should be easily understood even to “outsiders”
3. Documenting Business Impacts
After you’ve identified and evaluated operational definitions, the next step is to determine and catalog the purpose and impact of each CDE within the business.
“You’ll want to be able to understand what sort of impact occurs if Data Quality is poor, and you’ll also want to try to make that impact quantifiable,” said Doupe. “If you can quantify the impacts of bad Data Quality, you can much better represent the importance of fixing Data Quality to executives within your organization.”
In addition to assessing how and where data impacts various pressure points of the business, data stewards should document the functionality of these CDEs: How frequently does the company rely on the data element in question, how often are there Data Quality issues associated with the data element, and what steps, if any, are being taken toward improving Data Quality?
4. Mapping Data Locations
Once you’ve identified your company’s CDEs and their roles, Doupe suggests tagging the location where the data lives, so to speak, at every level of hierarchy – including all corresponding applications, databases, schemas, tables, and columns.
Tracing the source of data can prove to be an arduous task, so Doupe stresses the importance of assigning the right team members to the job.
“Data architects, software architects, and application technical owners are generally the best individuals to help with this documentation because they understand both the application and the system, and perhaps the database that is sitting behind it and the corresponding data that’s managed within it.”
Because these specialists already know the terrain, they can be useful not just in sourcing, but also in building cross-functional ties with business glossaries and data dictionaries.
5. Data Profiling
From here, the process crosses the threshold of what Doupe deems the essential “meat of Data Quality”: examining data sources at a multifaceted, granular level to check for inconsistencies. Such aspects may include anything from the lengths of data points and possible maximum or minimum values to the alphabetical or numeric categorization of the elements.
If you don’t have a data profiling tool, you can use SQL scripts. But Doupe warns that in this arena, you generally get what you pay for: “The value add of having an off-the-shelf data profiling tool is that they generally provide a nice user interface. It’s just a nice way to visualize your data source,” he explained. “And second, there’s the capability to slice and dice the data set faster than you would be able to with writing some humongous SQL script.”
6. Crafting Data Quality Rules
In addition to data profiling, organizations must clearly define the business requirements for each CDE. The following six Data Quality dimensions should figure into the equation:
- Timeliness: Is the data where it needs to be at the time it’s needed?
- Completeness: Is the data ready to be used as is?
- Uniqueness: Can the data be mistaken for similar elements?
- Consistency: Does the data retain its integrity within and across sets?
- Validity: Does the data fall within the specified limit requirements?
- Accuracy: Does the data represent reality?
7. Creating Data Quality Metrics
Measuring the effectiveness of these Data Quality rules helps create transparency across the organization. You can calculate a “Data Quality score” – dividing the total number of data failures by the sum of all data observations – to generate a percentage rating for the overall success of the data, then share the results using a data visualization BI tool.
“I stress the importance of making Data Quality metrics so that everyone has a common understanding of where true Data Quality issues exist, and where to focus efforts to fix them,” said Doupe. “If you’re currently at a Data Quality score of 80%, and your goal is to get to 85%, then you could put together a plan and track progress towards that plan.”
8. Locating Authoritative Sources
Although Doupe noted that this step might be conceived as an element of Data Architecture, he emphasized that its impact is relevant enough to be included as a step for improving Data Quality. This phase involves evaluating the longevity of data sources, in order to help data leaders decide where to concentrate their efforts.
“Let’s say your company is working on implementing a centralized master data management solution,” said Doupe. “Over the next five years, you’re going to go from having five systems that can create and update data down to just one authoritative source that creates and updates data. Knowing that piece of information then allows the Data Quality remediation efforts to be much more future-focused and less about firefighting – and spending all your time on the old legacy stuff.”
9. Planning Data Quality Remediation
The journey of improving Data Quality reaches its peak with constructing a framework that proactively prevents inaccuracies and discrepancies at the roots, rather than reacting to issues after they occur. Start with the CDEs that have low Data Quality scores, drilling down to figure out where and why Data Quality issues are starting.
“Like any sort of action plan your organization may develop, you’ll want to clearly define who is going to do what and by when,” said Doupe. “If you don’t have deadlines, we all know what happens: Action doesn’t happen.”
Conclusion
With these nine steps, it’s possible to create a robust, sustainable, cost-effective Data Quality program. Doupe advises working in batches of roughly 15 CDEs at a time, repeating as often as needed. This roadmap of operations will create a “virtuous cycle,” building upon itself to continually improve your Data Quality – and your business.
Want to learn more about DATAVERSITY’s upcoming events? Check out our current lineup of online and face-to-face conferences here.

Here is the video of the Enterprise Data Governance Online presentation:
Image used under license from Shutterstock.com