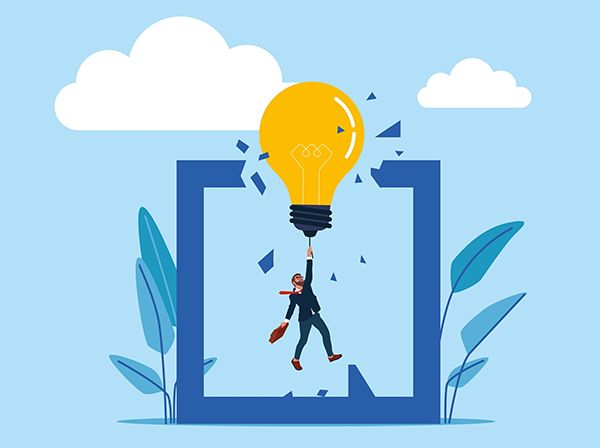
Leaders want “everyone, everywhere, and all at once to become highly data-literate, demonstrate a high capability to read, work with, and analyze data,” says Dr. Wendy Lynch, founder of Analytic-Translastor.com and Lynch Consulting. As a consultant to numerous Fortune 100 companies, she understands why organizations want all their members to have a high level of Data Literacy. Dr. Lynch highlights some of the biggest Data Literacy barriers and how to solve them during a DATAVERSITY webinar, “Overcoming Challenges to Achieving Data Literacy.” In her presentation, she explains and reframes challenges to Data Literacy training and encouraged a three-pronged approach to address them.
In the webinar, Dr. Lynch cited a study from McKinsey, noting that at least $1 out of $5 from a company’s earnings before interest and taxes (EBIT) translates into value from data assets. Moreover, businesses with the highest level of data mastery, including policies, people, and technology, have a 70% higher revenue per person.
Yet, nearly 80% of people lack confidence in their Data Literacy skills, and studies show that 90% do not have high Data Literacy. So, as Lynch points out, “Businesses want everyone to function as data scientists but are starting at a difficult place.”
Barriers to Data Literacy
Dr. Lynch cites eight themes from DATAVERSITY focus groups conducted in early 2023 to understand why people and organizations find Data Literacy training difficult. They include:
1. Buy-In: Leaders overestimate their workers’ capabilities with data and may not understand the importance of Data Literacy training or the priority of such efforts.
2. Ownership: Organizations need to clarify who drives Data Literacy efforts. Is it the person with the highest Data Literacy score, a C-level person, or a new role? Dr. Lynch observes that employees may hesitate or feel apprehensive about learning Data Literacy because they do not have the interest or aptitude. So, is the person who drives Data Literacy training responsible for mitigating these issues?
3. Measurements: How do organizations assess current levels of or improvements in Data Literacy? What represents a good level of Data Literacy? Moreover, based on a Forbes article, she mentions that should companies not get to a good level of Data Literacy, they would create a toxic divide between data producers and consumers – those who are literate and those who need to get to a higher level. So, how can measurements help advance Data Literacy without creating such a contentious environment among employees?
4. Training Approach: Lynch asks how we approach Data Literacy training. Do organizations do it company-wide? Do they select training from a vendor or from within the organization? Additionally, how does an organization’s trainer cover all significant steps to reach high Data Literacy, as listed below?
- Gain awareness of data available in the organization.
- Identify these different data sources.
- Know how to select the right sources at the right time.
- Understand the value and limitations of the data sets selected.
- Manipulate data to define and filter information capably.
- Analyze data, including using the calculations to get there.
- Interpret data and the results that follow reasonably.
- Apply this information to meet business and job requirements.
5. Duration/ Levels: How often do employees undergo training? Is it ongoing or once done? To illustrate this challenge, Dr. Lynch relates an experience examining AI’s implications in a medical institution. Physicians in this organization distrust AI at times and need some training. But she asks, “Do we want a doctor who has undergone 12 years of medical school to return to school to become a data scientist?”
6. Personnel: Does the organization have people that can help advance others’ Data Literacy to a higher level? Consider that one-third of Americans don’t know that a quarter of a pie chart is the same as 25%, and 22% don’t understand everyday numeric information like bank statements. Additionally, 20% of people have severe math anxiety that freezes their brains. So, does an organization have the resources to handle all these significant gaps?
7. Cost: Does the organization have the budget for Data Literacy? Training everyone costs a lot. Some organizations may consider saving money by encouraging employees to take no-cost self-driven online courses. However, several studies question the effectiveness of such an approach.
8. Time: Dr. Lynch highlights that time represents people’s most scarce resource. Organizations need to leverage time for daily operations and their data applications. So, how can companies allot time to combine Data Literacy training and have people learn, especially if employees are spread out geographically?
Reframing Data Literacy Training Barriers
As mentioned above, Dr. Lynch finds many complex Data Literacy training barriers when employees need to achieve high Data Literacy everywhere. So, she recommends reframing this Data Literacy problem at a team level to reduce these barriers most efficiently.
Not everyone has the same ability or interest in Data Literacy but has different ones that a business needs, like people literacy (emotional maturity and communication skills) and business literacy (understanding business priorities and strategic imperatives and how one’s job connects with that). When viewing Data Literacy in this way, Data Literacy challenges change and become more meaningful in the aggregate.
Then, organizations must ask how to best utilize their teams with collections of people with different strengths. Dr. Lynch explains it this way:
“Leaders want better Data Literacy not because they want every employee to love math. Instead, they want their organizations to get better insights. As more people, collectively, can go higher up in Data Literacy, the more you can get at these insights.”
In other words, managers want data skillsets or work collaborations to give each employee the knowledge and analytical access to do the job well.
A Three-Pronged Approach: Training, Roles, and Access
Given this new perspective, Dr. Lynch suggests organizations use a three-pronged approach through training, roles, and accessibility to achieve higher Data Literacy for organizational insights. She explains each of these further:
Training: Based on past data, Dr. Lynch advises the following best practices when doing Data Literacy:
- Designate a competent expert who owns the effort to improve literacy, and this person should be from something other than the Data Governance or a data area.
- Have a clear business case for what the organization will achieve when it reaches higher Data Literacy.
- Structure education to fit in with normal business operations and give relevant examples that tie any teaching to an employee’s role when that person learns.
Roles: As Dr. Lynch explores advancing Data Literacy collectively, she wonders about designating work to capitalize on people’s strengths and accommodate their weaknesses in addition to training. She even suggests possible combination roles.
For example, as Lynch works with her medical client, she sees AI experts (more tech-savvy) and clinical experts (better able to diagnose and treat patients). So, while allowing team members to improve their data skills, she implements translator roles between the AI and clinical experts.
These translator roles help the AI, and the clinical employees get data insights. Dr. Lynch asserts:
“Perhaps translators familiar with different data insights and who have basic SQL skills give information to everyone else. Then everyone has access to more advanced insights from the data.”
This way, the team can better process the information and complete each job. This approach also saves the time and money needed to train every individual to manipulate data, especially if that person is not interested in doing the math.
Access: Complex technology restricts how much training is needed, requiring extra time to show trainees how to find, retrieve, and manipulate data. To counter this issue, Dr. Lynch advocates for platforms that use data interfaces that require less technical skill, opening an organization’s usage, as the marketplace has done with computers.
She explains that in the 1970s, programmers and specialized engineers only used computers because they knew how. Then, advances in hardware, PCs, and GUIs opened computing access to everyone. Now, most people seamlessly use computers for their work, regardless of their knowledge of algorithms.
In the same way, Dr. Lynch says:
“We can start to think about analytics as more accessible. For example, instead of limiting data analysis to dashboard interactions and SQL queries, we could think of technology that converts queries, formed in a natural language, into analytics.”
Advances in AI and machine learning (ML) can potentially increase access to analytical data. Lynch points out that GPT-4 can convert spoken questions into SQL and produce graphics, showing the analysis, lowering Data Literacy requirements for insight.
Conclusion
Data Literacy barriers look complex and difficult, especially in getting every employee up to a higher level. So, while training provides a tool, organizations need other approaches.
Translator roles promise a bridge between data-literate and non-technical team members. Also, technological advances can lower the bar to gaining insights by opening access to less technical members. With this new perspective, executives can rethink Data Literacy training to address the eight barriers listed in this article.
Watch the webinar here:
Image used under license from Shutterstock.com