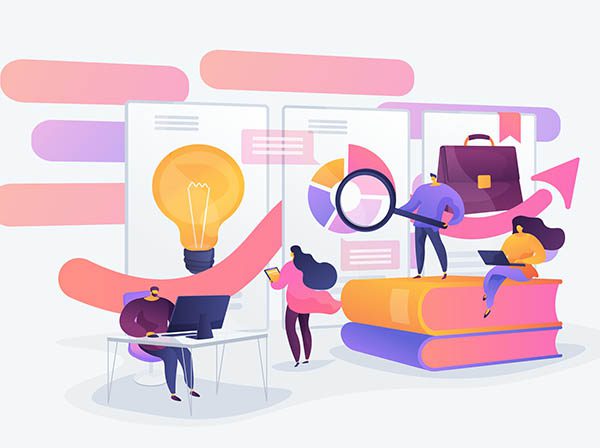
Every graph, data set, and chart has a story behind it. Knowing how to make sense of that narrative across an organization requires data literacy. Increasing the data literacy of smaller teams can be complicated, but equiping an entire organization is an even bigger task. So many teams bring in so many different skill sets with vastly different knowledge about the technical, business, and subject content of the company’s data. As a result, getting everyone to the same level of data literacy remains elusive.
Oliver Hughes, CEO and co-founder of Count.co. and Taylor Brownlow, Head of Data at Count.co, wanted to understand the biggest causes of data iliteracy across all levels. They watched thousands of participants in community and organizational workshops struggle with data confidence and understanding. From their fieldwork, they identified numerous issues involving corporate-wide data literacy gaps.
In a recent DATAVERSITY® interview Hughes said:
“Data literacy spans beyond servicing and empowering just one or two sub-groups. The whole team needs to use data better, collectively. This becomes problematic with a group of people who have a cross-functional range of skills, some really technical and very analytical, and others not. They have trouble finding a shared understanding of what the data is and how to use it effectively.”
Data Literacy Ties to Context and Complexity
During the interview, Hughes remarked that “the overarching question for organizations is: ‘How do our diverse teams, with different abilities, get to a common ground to use data effectively together and make great business decisions?”
He explained that this goal is actually more of a moving target than people realize. Using data effectively is something anyone can struggle with if they don’t have sufficient context. Take a data scientist, someone who has all the necessary skills to extract value from data, but in a certain circumstance can be totally ineffective if they do not understand the context of the information they are using. Hughes explained that if they do not understand where data is from or its subject matter then this would render even the most proficient data expert effectively illiterate because they would have no idea how to use and answer questions with the data.
He added:
“In an extreme example, a person receives a column of numbers without a header and without any idea as to the field name of that column. In that context, that person may as well have no data skills because they can’t do anything meaningful with the data.”
Every team member has to have the context of a data set to use it effectively, while also understanding its importance. As the world gets more complicated the urgency for doing so has become even more crucial.
“About fifty years ago, a foreman running a factory could eyeball different mechanisms and processes while walking on the factory floor to know how to improve their factory. Now such a person has so many interconnected pieces offering incrementally smaller improvement margins that they can’t be seen by the naked eye. Data, then, becomes the only effective means to view your operations, understand trends, and make improvements,” he said.
Outside of the factory floor, across the board in every industry, as Hughes said, the business value depends on data, just as corporate operations have become more invisible and sophisticated. The challenge now becomes how to make sure this data is defined and understandable so it can be used effectively.
The Data Literacy Expertise Dilemma
Throw into the mix different skills, data contexts, and business intricacy, and the collective data literacy gap widens. Brownlow stated:
“The increased use of complex data has led to specialization. Anyone using data now needs to obtain skills not required ten years ago to do anything meaningful with it.”
Given the clear value of data in business each worker feels a pressure to adapt, learn, and be proficient with data. But the time it takes can be a real barrier. As people try to get more skills, said Brownlow, technology moves fast away from them. Data keeps increasing in size and complexity which drives the need for ever-new technologies which can cope.
“Businesses have so many different data types and sources all with different meanings and version types that new technologies to help manage and use all these differences are constantly required.”
There is just too much for one person to know. To solve this, companies purchase data tools to make simpler and prettier dashboards and charts, so a user can access data in an intuitive way. But, as Hughes emphasized, this kind of product does not give someone an understanding of the underlying data set, the definitions around it, or how to succinctly communicate this information to others. That information remains hidden behind the typical data platform.
The Top Drives Collective Data Literacy
Notes about the data and its narrative is key, they both agreed. But the data’s story needs to be coherent, where collaboratively, people are on the same page about the data and the world around it. Organizations typically have three types of proficiencies:
- Business Intelligence (BI): Understanding data
- Data Governance: Valuing data
- Data Science: Leveraging data
When asked which capability is most important, Brownlow suggested that each contributes equally and build and reinforce each other; it is just as important for the business to invest in Data Science as infrastructure. She believes in a top-down approach, where leaders make a strategic decision to invest in data literacy.
Often, data literacy challenges are most evident when executives ask for data-driven evidence. Their query flows down the company, requiring, as Brownlow said:
“If there isn’t a good baseline of understanding within an organization about what data is available, how it connects together and represents the outside world then things can quickly become a real mess for reactive decision making. This kind of basic data understanding, with everyone interacting with it day-to-day, needs to come first.”
Hughes suggested friction points can become more obvious when management’s requests take too long to deliver and people realize that the supporting information is not quite right. These situations can be a great way to demonstrate the value of investing further in data literacy.
Executives drive the business and their effectiveness is only as good as the baseline level of data literacy within their business.
Data Literacy is Not Just Syntax
Even when managers lead a data literacy charge, Hughes noted that people confuse training as akin to teaching a particular tool, such as SQL or Excel. But this approach has a problem. Hughes said:
“Teaching how a tool works is very different to explaining the principles behind good analysis, interrogation, and the statistics in a way that makes the analysis happen. People first need the confidence to know how to ask the right questions then they can learn how to apply that to a particular tool. You can’t do it the other way around.”
He noted that often these core data handling skills are often under-invested-in and have to be picked up through repetition – slowing progress dramatically. Fortunately, formal training does exist and promises to be more effective if used before or in parallel with tool-focused teaching. For example, Count has put together a series of resources which can be used by organizations regardless of the data tools they use internally.
Formalizing Data Literacy Training with Lunch and Learn
Brownlow believes in formally teaching data analysis principles, rather than letting people flounder alone. The training should be short but regular, focusing on a few key points at a time. As suggested in their training material, lunch and learn sessions can be a great way to start. She said:
“Lunch and learn sessions provide a way to teach and gather insights because they allow all sides to come together in an informal way and have an open conversation about the data and any challenges which need to be addressed. From this kind of session, a team member, for example, can find out that a certain data table needs better explanation or edits because a particular field is no longer in use.”
Brownlow believes lunch and learn sessions help the entire organization become more data literate as a unit, from the bottom up, by getting people together and having an open conversation about the data and its implications.
Narrowing the Collaborative Data Literacy Gap
In addition to lunch and learns, a software platform like Count’s narrows the gap by empowering “cross-functional and cross-skill teams to be better at data together,” through the following three benefits:
- Transparency in a shared context: Trust binds teams together, and Hughes strongly believes in transparency to see what has been done to the data, where, and from what context. Doing this builds consensus and allows teams to make decisions faster.
- Value added by everyone: “Great decisions require everyone’s input,” said Hughes. Although some people can manipulate raw data better than others, everyone has a perspective about the information, and these views can be added to the narrative.
- Focus on all users: They advocate for a simple, common place where everyone can work together and enjoy working with the tools.
Count’s platform puts these principles front and center through an electronic notebook for everyone. The tool combines data cleaning, analysis, visualization, discussion, and narration in one document. “It’s the first data platform where there is end-to-end visibility of every analytical stage, from raw data to final report.” according to Hughes.
Also, the web-based and mobile-friendly notebooks store context and definition, clarifying contexts and reducing complexities. “From there, everyone can comment about the definitions in the notebooks and be confident with how the data is being represented, making group decision-making easier.”
Count also provides three modules about fundamental data skills, querying data, and assembling the analysis. These training tools are agnostic and teach data analysis principles to people with a wide range of abilities, closing the collaborative data literacy gap more tightly. They find transparency and common language essential to increase data literacy and the effectiveness of teams to work with data and are making available a platform supporting high data literacy achievements by everyone.
Image used under license from Shutterstock.com